Mix and Match: Learning-free Controllable Text Generationusing Energy Language Models
Proceedings of the 60th Annual Meeting of the Association for Computational Linguistics (Volume 1: Long Papers)(2022)
摘要
Recent work on controlled text generation has either required attribute-based fine-tuning of the base language model (LM), or has restricted the parameterization of the attribute discriminator to be compatible with the base autoregressive LM. In this work, we propose Mix and Match LM, a global score-based alternative for controllable text generation that combines arbitrary pre-trained black-box models for achieving the desired attributes in the generated text without involving any fine-tuning or structural assumptions about the black-box models. We interpret the task of controllable generation as drawing samples from an energy-based model whose energy values are a linear combination of scores from black-box models that are separately responsible for fluency, the control attribute, and faithfulness to any conditioning context. We use a Metropolis-Hastings sampling scheme to sample from this energy-based model using bidirectional context and global attribute features. We validate the effectiveness of our approach on various controlled generation and style-based text revision tasks by outperforming recently proposed methods that involve extra training, fine-tuning, or restrictive assumptions over the form of models.
更多查看译文
关键词
text,energy,language,models,learning-free
AI 理解论文
溯源树
样例
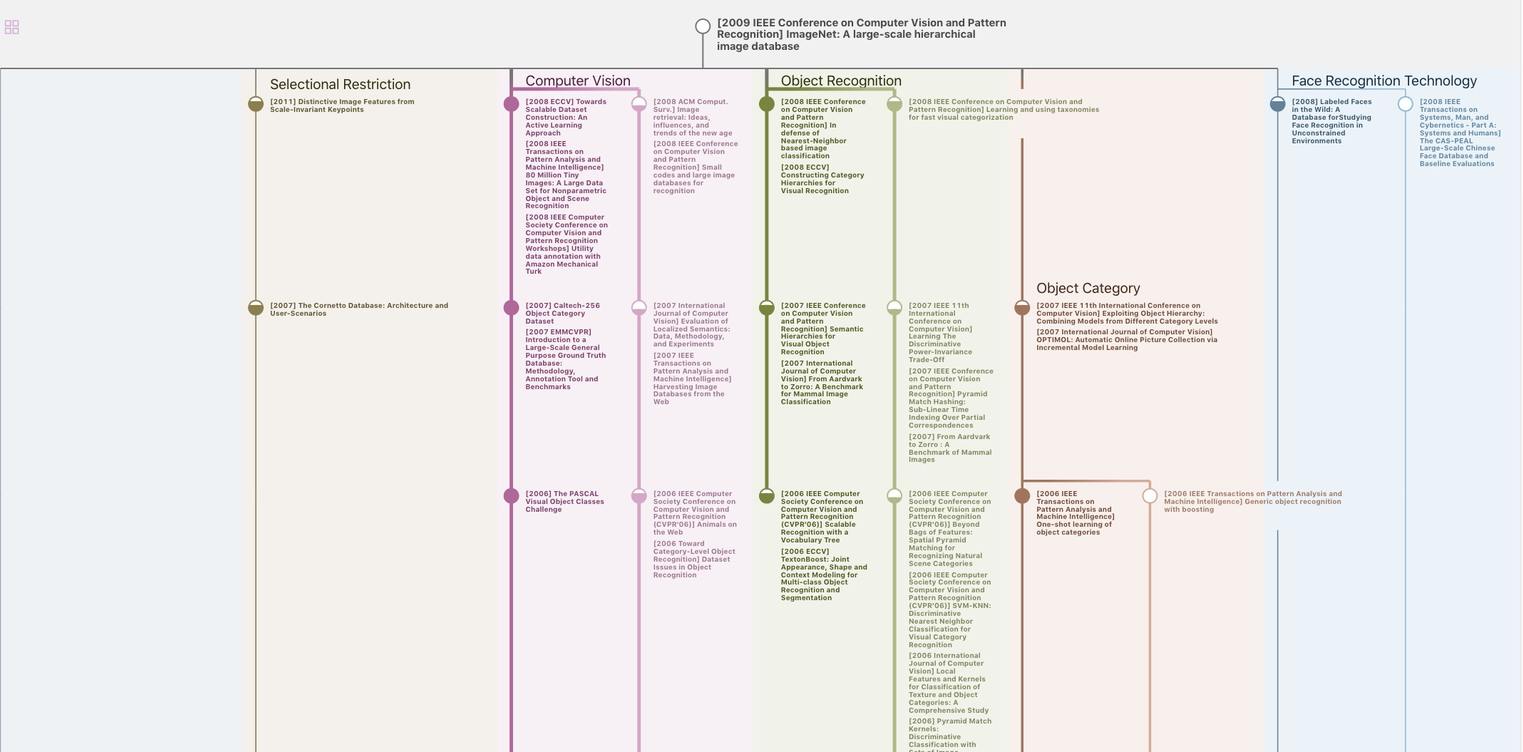
生成溯源树,研究论文发展脉络
Chat Paper
正在生成论文摘要