Adversarial Graph Disentanglement With Component-Specific Aggregation.
IEEE Trans. Artif. Intell.(2024)
摘要
A real-world graph has a complex topological structure, which is often formed by the interaction of different latent factors. Disentanglement of these latent factors can effectively improve the robustness and expressiveness of the node representation of a graph. However, most existing methods lack consideration of the intrinsic differences in relations between nodes caused by factor entanglement. In this paper, we propose an
A
dversarial
D
isentangled
G
raph
C
onvolutional
N
etwork (ADGCN) for disentangled graph representation learning. To begin with, we point out two aspects of graph disentanglement that need to be considered, i.e., micro-disentanglement and macro-disentanglement. For them, a component-specific aggregation approach is proposed to achieve micro-disentanglement by inferring latent components that caused the links between nodes. On the basis of micro-disentanglement, we further propose a macro-disentanglement adversarial regularizer to improve the separability among component distributions, thus restricting the interdependence among components. Additionally, to reveal the topological graph structure, a diversity-preserving node sampling approach is proposed, by which the graph structure can be progressively refined in a way of local structure awareness. The experimental results on various real-world graph data verify that our ADGCN obtains more favorable performance over currently available alternatives. The source codes of ADGCN are available at
https://github.com/SsGood/ADGCN
.
更多查看译文
关键词
Adversarial learning,graph disentanglement,graph neural networks,graph representation learning
AI 理解论文
溯源树
样例
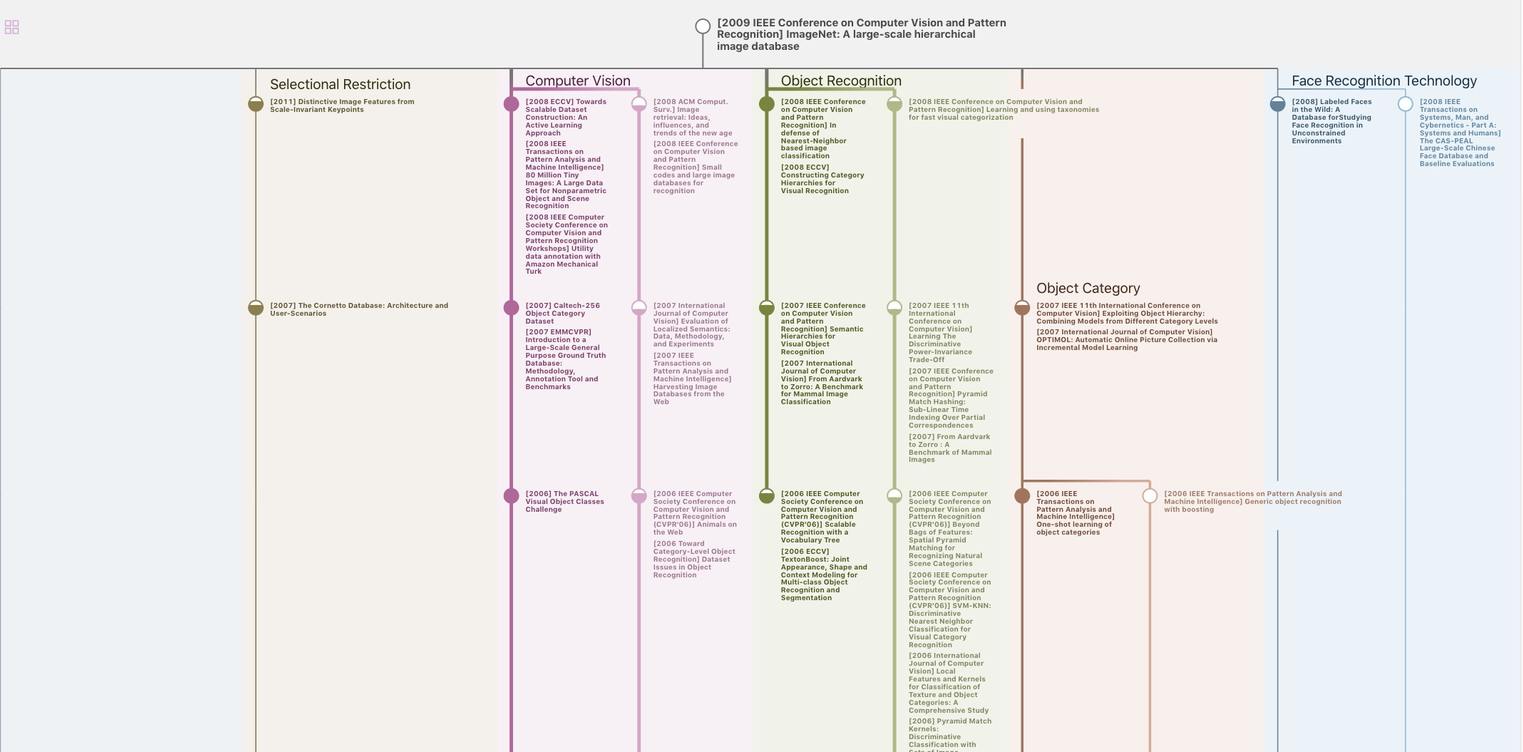
生成溯源树,研究论文发展脉络
Chat Paper
正在生成论文摘要