Abstract 5411: Efficient Development of Prognostic Tests for Detecting Cancer Risk Using Proteomic Technology
Cancer research(2023)
摘要
Background: Prognostic models for assessing future health outcomes can be developed using time-to-event (also known as “survival”) data. This methodology is ubiquitous in statistical literature and in the analysis of cancer outcomes, but its use in high-dimensional analyses tends to be limited as the methods are difficult to implement in a machine learning environment. Additionally, development of certified prognostic clinical tests using proteomic biomarkers for detecting future cancer risk can be time-consuming, prone to overfitting issues, and difficult to navigate. We demonstrate the utility of combining SomaScan® proteomic data with pipeline machine learning tools and survival analysis methodology to identify powerful and robust LDT-certifiable prognostic tests for assessing future risk of cancer. Methods: Data pipeline and analysis tools were developed using R. In addition to standard machine learning techniques, statistical methods include elastic net AFT models, subsampling survival techniques, and metrics for assessing predictive survival models. The pipeline takes the analyst from data processing and QC through identification of optimal models for prediction of clinical endpoints, and then through validation on a hold-out test set. The tools include an assessment of model robustness against sample handling issues, longitudinal stability, the impacts of assay noise on model performance, effects of putative interferents, and risk of failure during CLIA validation in the lab. We demonstrate the utility of the tools and methods for development of a lung cancer risk model. Results: Analysis time for validation of an optimal clinical model was reduced by at least 80%, resulting in the development of 7 LDT-certified tests within 3 years, including a test for lung cancer risk. Inclusion of methods that allow for subsampling and penalized regression using AFT models show improved predictive performance and identification of top features related to clinical endpoints. Conclusion: Not only are powerful, prognostic tests do-able, but they can be LDT certified in an efficient manner and made to be robust to real-life lab settings. Survival analysis in a machine-learning setting allow us to leverage proteomic technology in new ways, leading to tests that assess future cancer risk, which can be used for precision medicine applications. Citation Format: Yolanda Hagar, Leigh Alexander, Jessica Chadwick, Gargi Datta, Joe Gogain, Rachel Ostroff, Clare Paterson, Laura Sampson, Caleb Scheidel, Sama Shrestha, Amy Zhang, Michael Hinterberg. Efficient development of prognostic tests for detecting cancer risk using proteomic technology. [abstract]. In: Proceedings of the American Association for Cancer Research Annual Meeting 2023; Part 1 (Regular and Invited Abstracts); 2023 Apr 14-19; Orlando, FL. Philadelphia (PA): AACR; Cancer Res 2023;83(7_Suppl):Abstract nr 5411.
更多查看译文
AI 理解论文
溯源树
样例
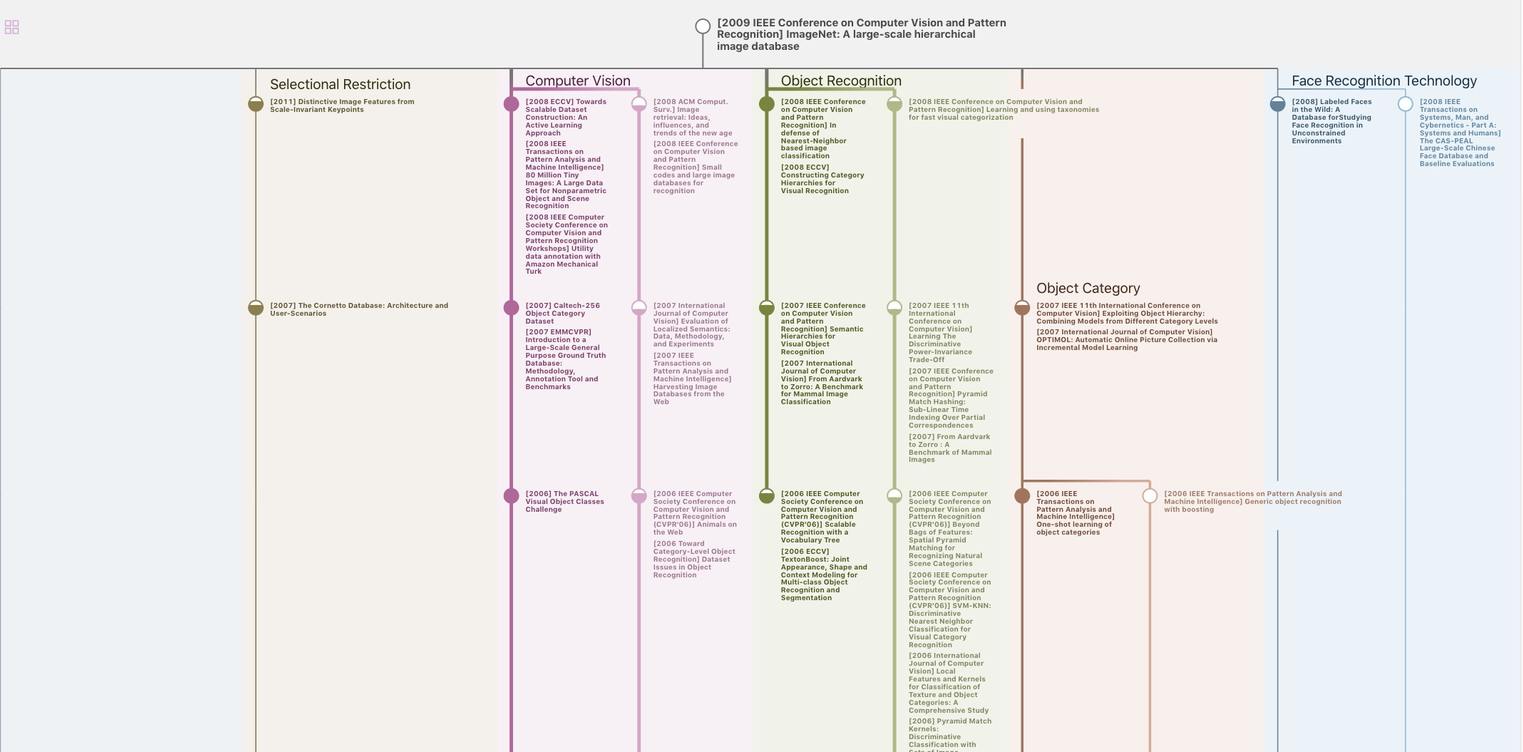
生成溯源树,研究论文发展脉络
Chat Paper
正在生成论文摘要