Collaborative filter pruning for efficient automatic surface defect detection
IEEE TRANSACTIONS ON INDUSTRIAL INFORMATICS(2024)
摘要
Surface defect detection is a critical task in industrial production, and numerous methods have been proposed to achieve high detection accuracy. Although deep learning-based approaches have achieved state-of-the-art performances, their vast computational cost and high memory footprint prevent their deployment in resourceconstrained environments. To address this problem, we propose a collaborative filter pruning method for the defect detection model, which significantly reduces the number of required calculations and parameters while maintaining high performance, even in cases with tasks suffering from the class imbalance problem. Our method aims to obtain lightweight pruned models by removing unimportant filters according to their importance evaluated by both structural similarity and detail richness of corresponding feature maps. Moreover, to improve the performance of pruned models, we propose a knowledge-fused fine-tuning approach that fuses the knowledge derived from two teacher networks to look after both representation learning and classifier learning, alleviating the class imbalance problem. Experimental results on four public datasets demonstrate that the proposed approach performs favorably relative to the state-of-the-art methods. In particular, the proposed method achieves 39x and 59x parameter compression for VGG-16 and ResNet-50, respectively, on the NEU-CLS dataset, with a very small detection accuracy loss (< 0.2%). The code is available at https://github.com/HXuanWang/CFP.
更多查看译文
关键词
Defect detection,convolutional neural network (CNN),model compression,network pruning,collaborative pruning
AI 理解论文
溯源树
样例
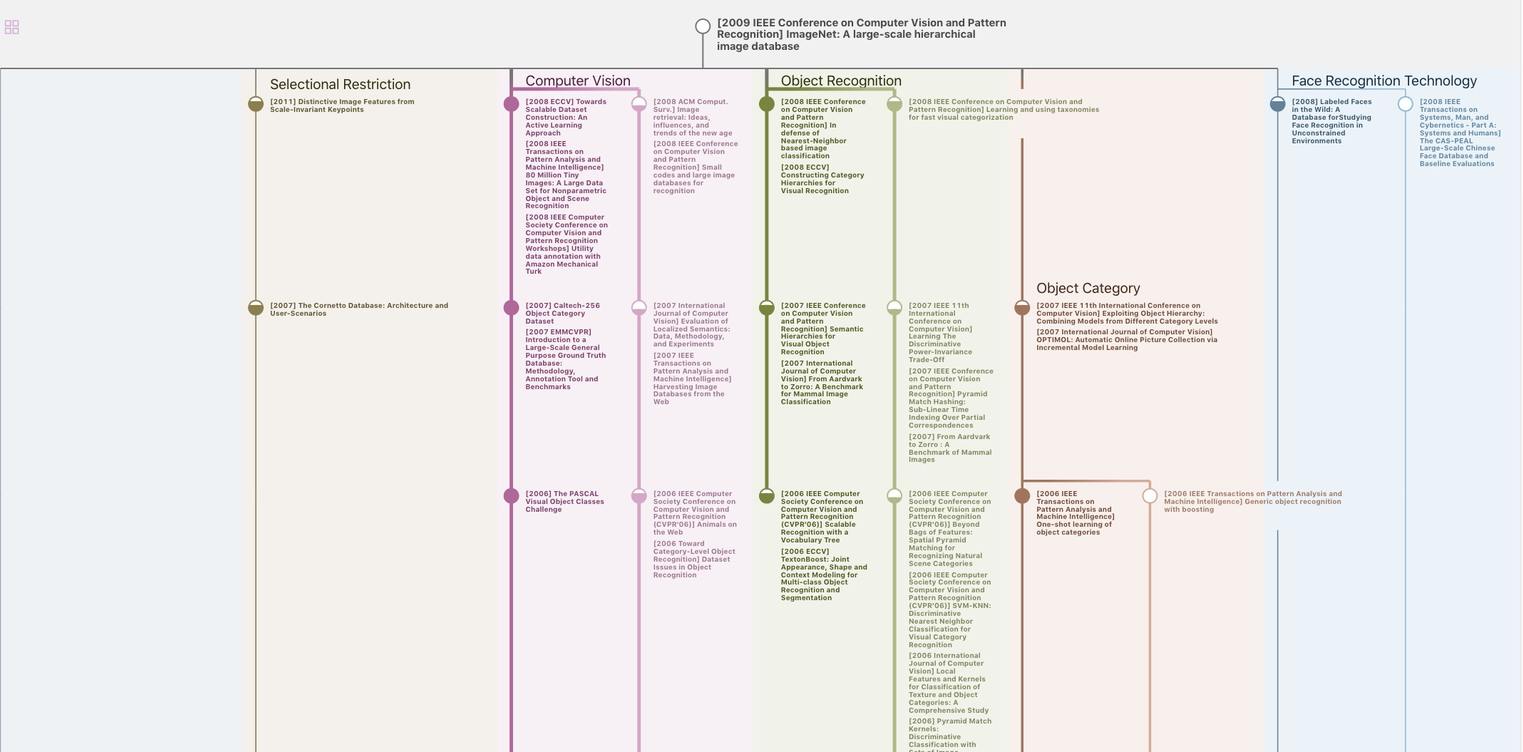
生成溯源树,研究论文发展脉络
Chat Paper
正在生成论文摘要