Dynamically Shifting Multimodal Representations via Hybrid-Modal Attention for Multimodal Sentiment Analysis
IEEE TRANSACTIONS ON MULTIMEDIA(2024)
摘要
In the field of multimodal machine learning, multimodal sentiment analysis task has been an active area of research. The predominant approaches focus on learning efficient multimodal representations containing intra- and inter-modality information. However, the heterogeneous nature of different modalities brings great challenges to multimodal representation learning. In this article, we propose a multi-stage fusion framework to dynamically fine-tune multimodal representations via a hybrid-modal attention mechanism. Previous methods mostly only fine-tune the textual representation due to the success of large corpus pre-trained models and neglect the inconsistency problem of different modality spaces. Thus, we design a module called the Multimodal Shifting Gate (MSG) to fine-tune the three modalities by modeling inter-modality dynamics and shifting representations. We also adopt a module named Masked Bimodal Adjustment (MBA) on the textual modality to improve the inconsistency of parameter spaces and reduce the modality gap. In addition, we utilize syntactic-level and semantic-level textual features output from different layers of the Transformer model to sufficiently capture the intra-modality dynamics. Moreover, we construct a Shifting HuberLoss to robustly introduce the variation of the shifting value into the training process. Extensive experiments on the public datasets, including CMU-MOSI and CMU-MOSEI, demonstrate the efficacy of our approach.
更多查看译文
关键词
Transformers,Acoustics,Visualization,Feature extraction,Task analysis,Logic gates,Sentiment analysis,Multi-stage fusion framework,intra- and inter-modality dynamics,multimodal representations shifting,hybrid-modal attention
AI 理解论文
溯源树
样例
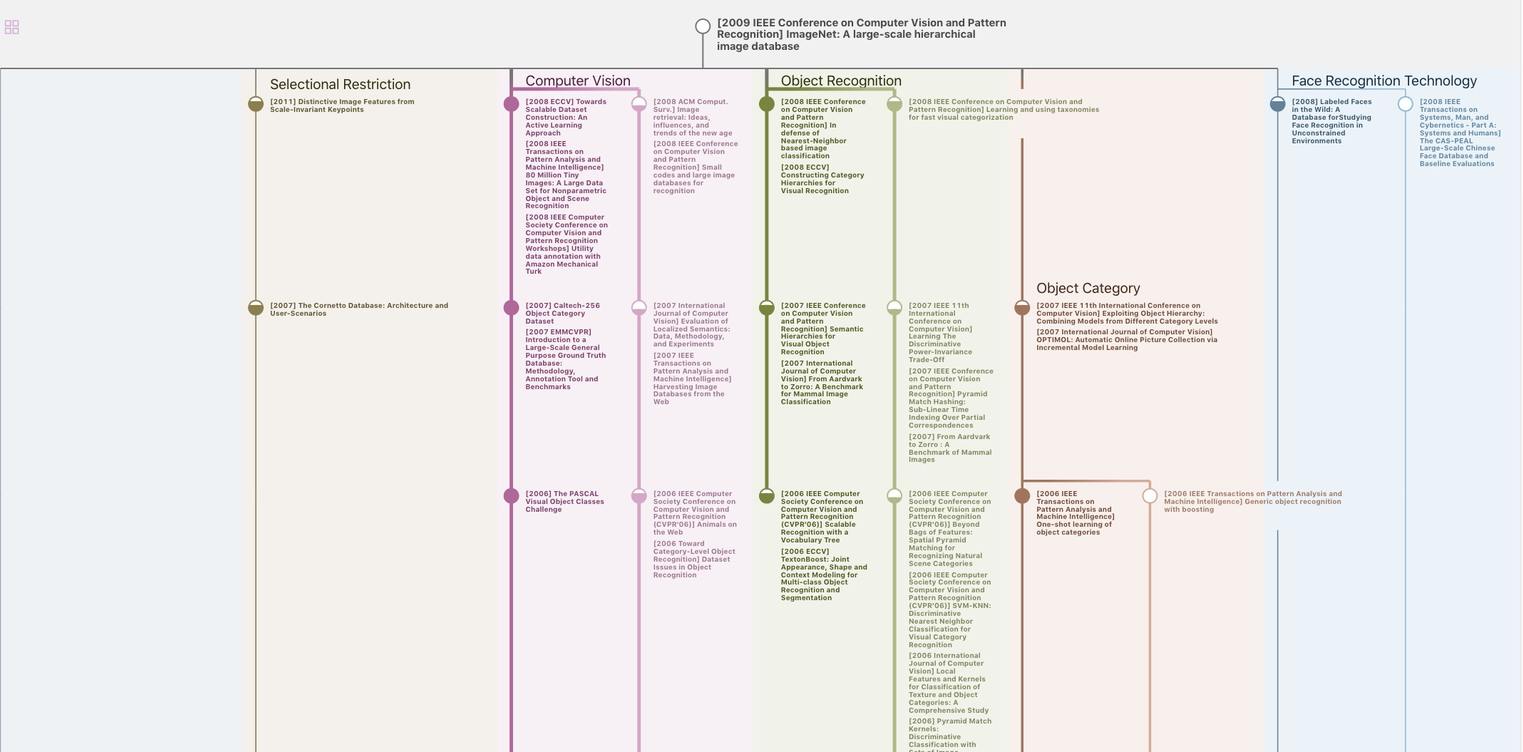
生成溯源树,研究论文发展脉络
Chat Paper
正在生成论文摘要