Tri-Level Modality-Information Disentanglement for Visible-Infrared Person Re-Identification
IEEE TRANSACTIONS ON MULTIMEDIA(2024)
摘要
Aiming to match the person identity between daytime VISible (VIS) and nighttime Near-InfraRed (NIR) images, VIS-NIR re-identification (Re-ID) has attracted increasing attention due to its wide applications in low-light scenes. However, dramatic modality discrepancies between VIS and NIR images lead to a considerable intra-class gap in the feature space, which impacts identity matching. To bridge the modality gap, we propose a Tri-level Modality-information Disentanglement (TMD) to disentangle modality information at the levels of raw image, features distribution and instance features. Our model consists of three key modules, including Style-Aligned Converter (SAC), Two-Steps Wasserstein Loss (TSWL) and Self-supervised Orthogonal Disentanglement (SOD) to handle the modality information at the three levels. Firstly, aiming at reducing modality discrepancy at image-level, the SAC is introduced to generate style-aligned images by the designed style converter and A-distance learning approach. The SAC can effectively alleviate the style discrepancy between VIS and NIR images with a negligible increase in model complexity. Secondly, considering the heterogeneity of VIS and NIR feature distribution caused by the structure- and style-misaligned raw images, we propose the TSWL to decrease the VIS-NIR gap at distribution-level by two distribution alignment steps. Specifically, after generating style-consistent images, we eliminate modality-related discrepancy by aligning the distribution between structure-aligned original and generated VIS/NIR images and bridge the modality-unrelated gap by aligning the style-consistent generated VIS-NIR images. Thirdly, focusing on further reducing the modality discrepancy at instance-level, the SOD is presented to construct orthogonal constraints between the extracted modality- and identity-related features. Since the modality-related factors are disentangled from the instance features, the proposed TMD efficiently learns the modality-unrelated and identity-discriminative representations, which are productive to conduct person Re-ID task on the VIS-NIR images. Comprehensive experiments are carried out on two cross-modality pedestrian Re-ID datasets to demonstrate the effectiveness of TMD.
更多查看译文
关键词
Feature extraction,Complexity theory,Cameras,Representation learning,Bridges,Pedestrians,Adaptation models,NIR-VIS Re-ID,modality-invariant repre- sentation learning
AI 理解论文
溯源树
样例
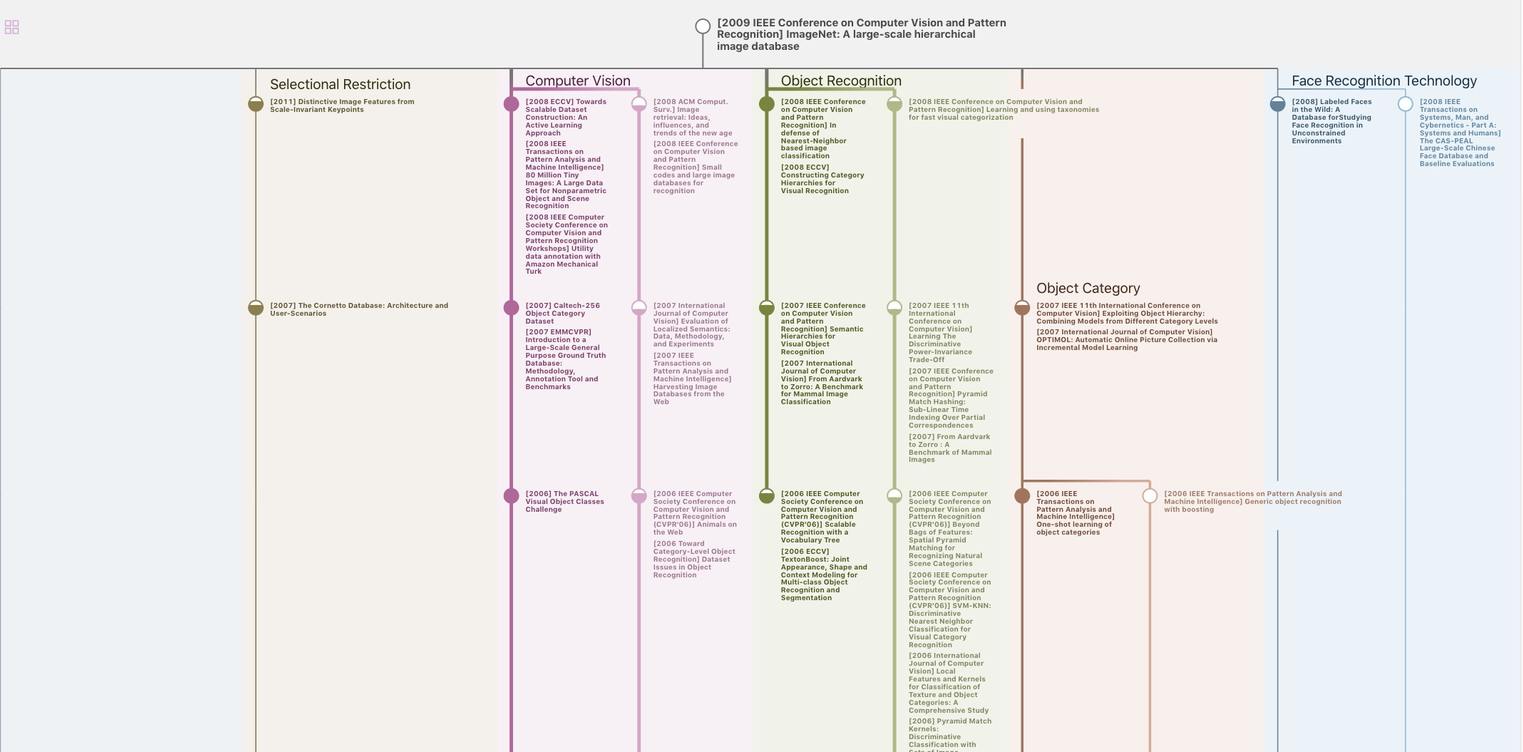
生成溯源树,研究论文发展脉络
Chat Paper
正在生成论文摘要