Real-Time Sequential Security-Constrained Optimal Power Flow: A Hybrid Knowledge-Data-Driven Reinforcement Learning Approach
IEEE TRANSACTIONS ON POWER SYSTEMS(2024)
摘要
To confront the inaccuracy and imperfection of the environmental model, this article proposes a hybrid knowledge-data-driven reinforcement learning (KDD-RL) approach to solve the sequential optimal power flow problem during real-time operation. An improved soft actor-critic algorithm is proposed to train the control policy and formulate the sequential dispatch commands to the generators. To promote the safe exploration of the reinforcement learning algorithm, a hybrid knowledge-data-driven safety layer is developed to convert the unsafe actions into the safety region. Furthermore, a security-constrained linear projection model with an inactive constraint identification process is proposed to accelerate the computation efficiency of the safety layer. Numerical simulation results verify the superiority and scalability of the proposed approach in improving the decision-making efficiency and promoting the security operation of the power systems.
更多查看译文
关键词
Power systems,Safety,Reinforcement learning,Security,Load flow,Generators,Training,Optimal power flow,economic dispatch,reinforcement learning,safety layer
AI 理解论文
溯源树
样例
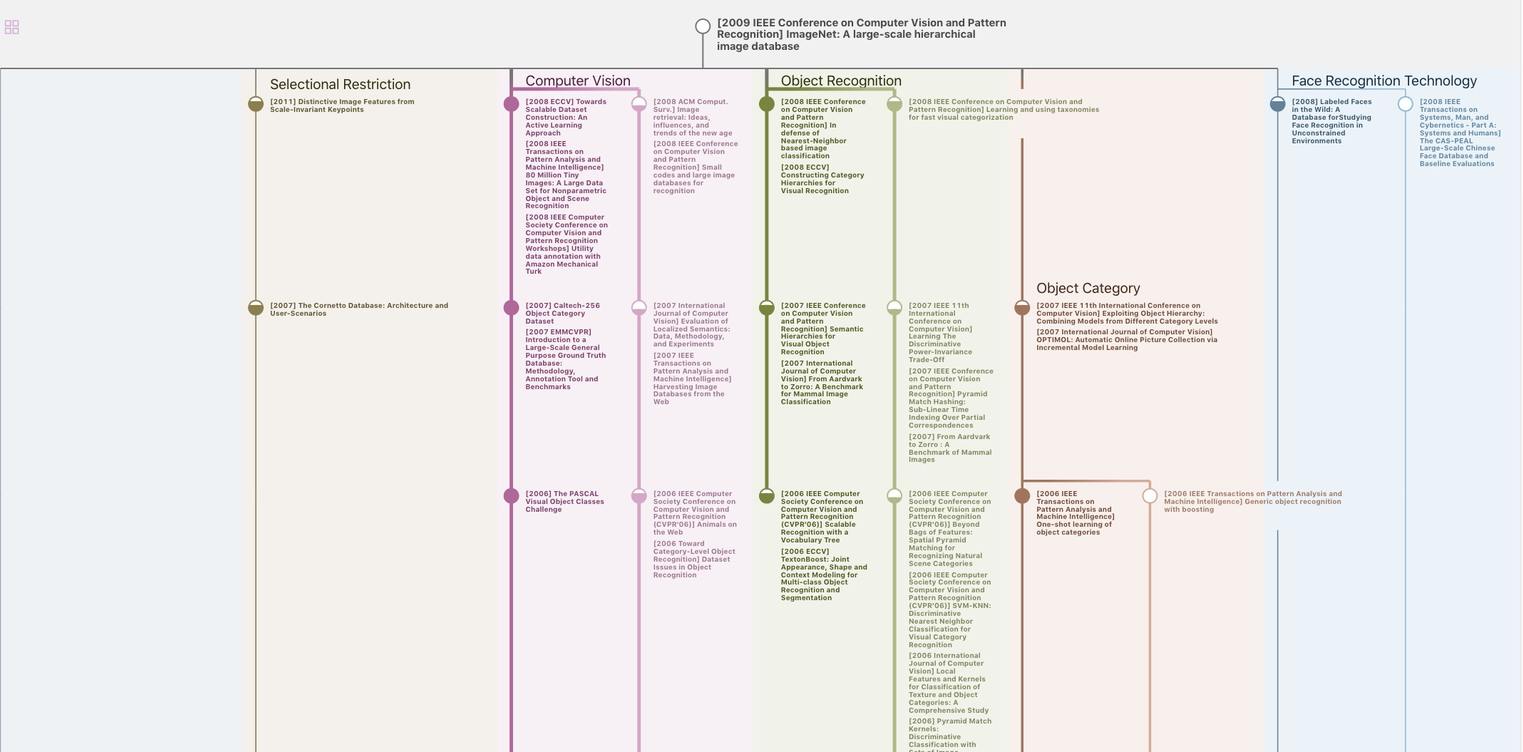
生成溯源树,研究论文发展脉络
Chat Paper
正在生成论文摘要