Autoencoder-Based Collaborative Attention GAN for Multi-Modal Image Synthesis
IEEE TRANSACTIONS ON MULTIMEDIA(2024)
摘要
Multi-modal images are required in a wide range of practical scenarios, from clinical diagnosis to public security. However, certain modalities may be incomplete or unavailable because of the restricted imaging conditions, which commonly leads to decision bias in many real-world applications. Despite the significant advancement of existing image synthesis techniques, learning complementary information from multi-modal inputs remains challenging. To address this problem, we propose an autoencoder-based collaborative attention generative adversarial network (ACA-GAN) that uses available multi-modal images to generate the missing ones. The collaborative attention mechanism deploys a single-modal attention module and a multi-modal attention module to effectively extract complementary information from multiple available modalities. Considering the significant modal gap, we further developed an autoencoder network to extract the self-representation of target modality, guiding the generative model to fuse target-specific information from multiple modalities. This considerably improves cross-modal consistency with the desired modality, thereby greatly enhancing the image synthesis performance. Quantitative and qualitative comparisons for various multi-modal image synthesis tasks highlight the superiority of our approach over several prior methods by demonstrating more precise and realistic results.
更多查看译文
关键词
Image synthesis,Collaboration,Task analysis,Generative adversarial networks,Feature extraction,Data models,Image reconstruction,Multi-modal image synthesis,collaborative attention,single-modal attention,multi-modal attention
AI 理解论文
溯源树
样例
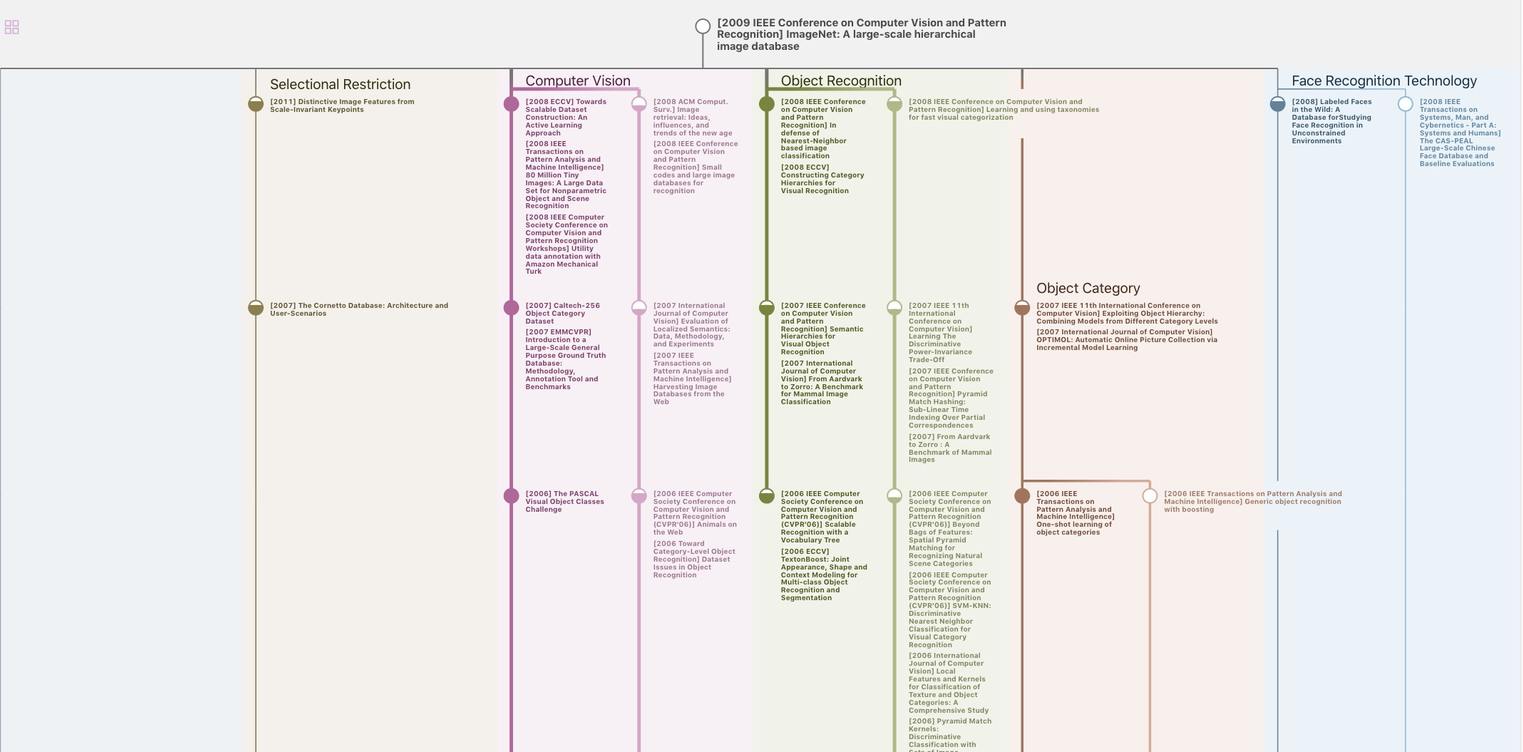
生成溯源树,研究论文发展脉络
Chat Paper
正在生成论文摘要