PhotoVerse: Tuning-Free Image Customization with Text-to-Image Diffusion Models
arXiv (Cornell University)(2023)
摘要
Personalized text-to-image generation has emerged as a powerful and sought-after tool, empowering users to create customized images based on their specific concepts and prompts. However, existing approaches to personalization encounter multiple challenges, including long tuning times, large storage requirements, the necessity for multiple input images per identity, and limitations in preserving identity and editability. To address these obstacles, we present PhotoVerse, an innovative methodology that incorporates a dual-branch conditioning mechanism in both text and image domains, providing effective control over the image generation process. Furthermore, we introduce facial identity loss as a novel component to enhance the preservation of identity during training. Remarkably, our proposed PhotoVerse eliminates the need for test time tuning and relies solely on a single facial photo of the target identity, significantly reducing the resource cost associated with image generation. After a single training phase, our approach enables generating high-quality images within only a few seconds. Moreover, our method can produce diverse images that encompass various scenes and styles. The extensive evaluation demonstrates the superior performance of our approach, which achieves the dual objectives of preserving identity and facilitating editability. Project page: https://photoverse2d.github.io/
更多查看译文
关键词
photoverse,diffusion
AI 理解论文
溯源树
样例
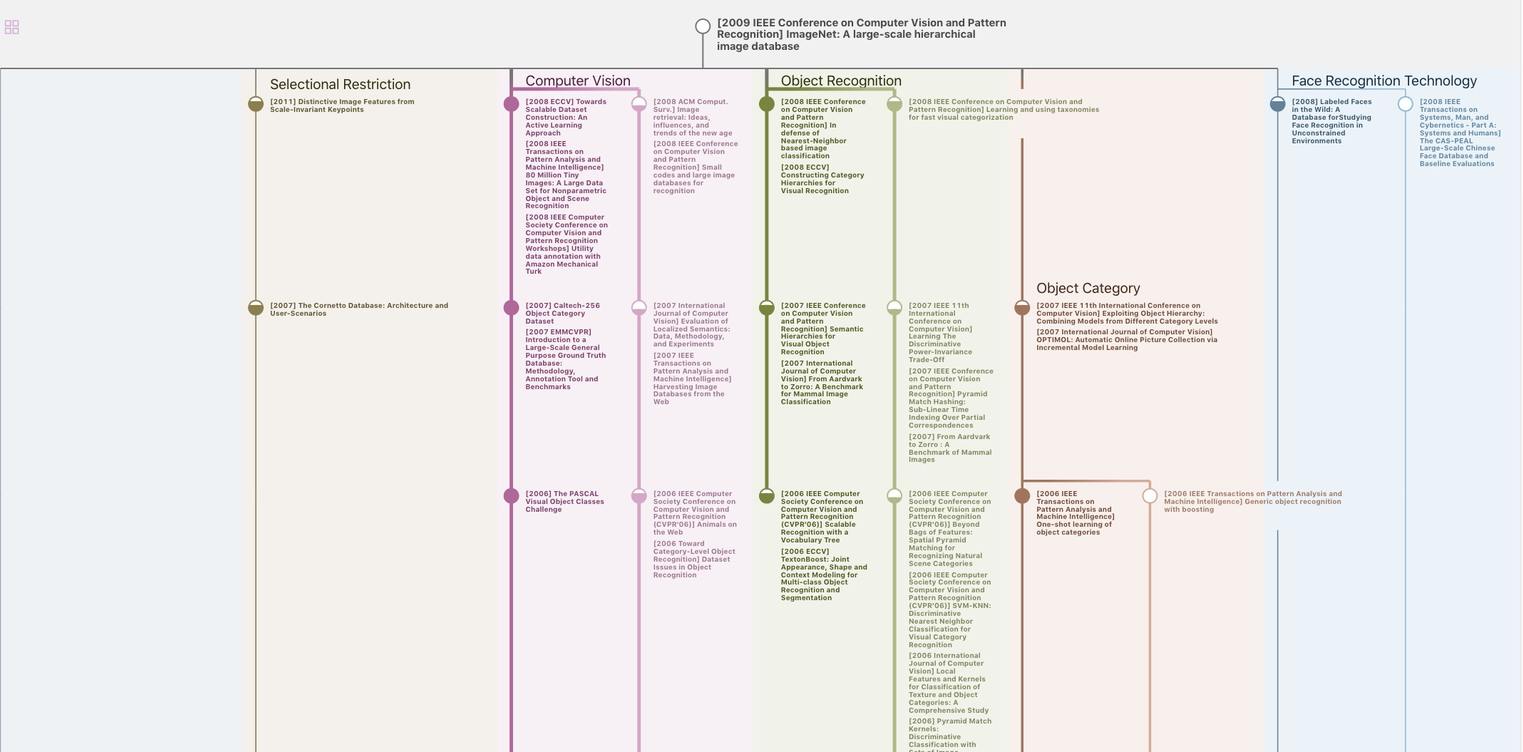
生成溯源树,研究论文发展脉络
Chat Paper
正在生成论文摘要