Case Study: Siemens – Flexible Robot Grasping with Deep Neural Networks
Springer handbooks(2023)
摘要
Universal grasping of a diverse range of objects is a major challenge in e-commerce order fulfillment and manufacturing. Recently, deep learning-based approaches for grasp point computation have demonstrated promising results that make them increasingly interesting for industrial deployments. This case study explores the problem of industrial flexible grasping from an automation systems point-of-view. We introduce a robotic grasping system that is based on a deep convolutional neural network, which is fully integrated at the controller level. The neural network is deployed on a novel industrial AI hardware acceleration module close to a PLC with a power footprint of less than 10 W for the overall system. The software is tightly integrated with the hardware allowing for fast and efficient data processing and real-time communication. The system runs reliably at a pick rate of more than 600 picks per hour. It has been deployed and tested in various industrial scenarios.
更多查看译文
关键词
flexible robot grasping,neural networks,siemens
AI 理解论文
溯源树
样例
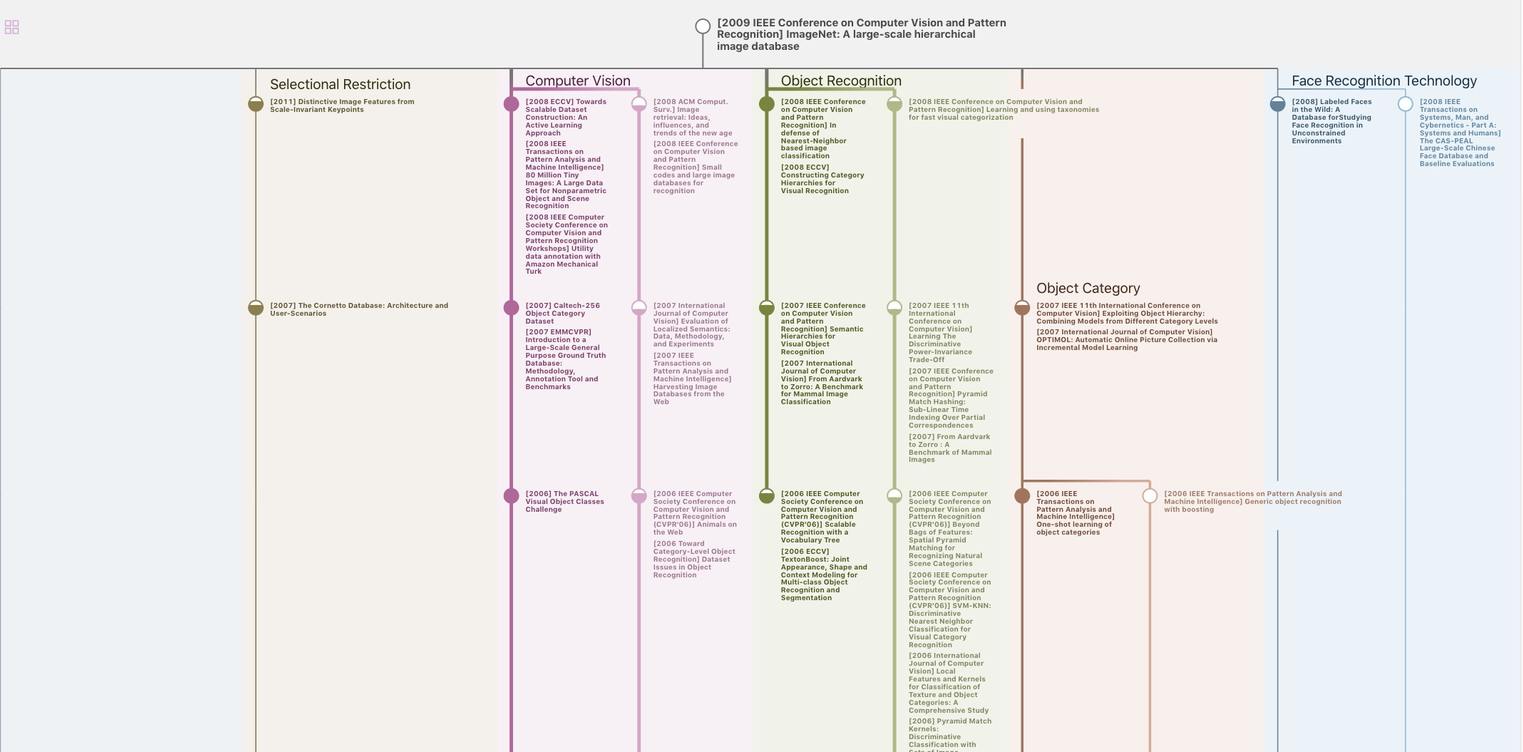
生成溯源树,研究论文发展脉络
Chat Paper
正在生成论文摘要