Neural Abstractive Summarization for Long Text and Multiple Tables
IEEE transactions on knowledge and data engineering(2024)
摘要
The Abstractive summarization aims to generate a concise summary covering the input document's salient information. Within a report document, the salient information can be scattered in the textual and non-textual content. However, existing document summarization datasets and methods usually focus on the text and filter out the non-textual content. Missing tabular data can limit produced summaries' informativeness, especially when summaries require covering quantitative descriptions of critical metrics in tables. Existing datasets and methods cannot meet the requirements of summarizing long text and dozens of tables in each report document. To deal with the scarcity of available datasets, we propose FINDSum, the first large-scale dataset for long text and multi-table summarization. Built on 21,125 annual reports from 3,794 companies, FINDSum has two subsets for summarizing each company's results of operations and liquidity. Besides, we present four types of summarization methods to jointly consider text and table content when summarizing reports. Additionally, we propose a set of evaluation metrics to assess the usage of numerical information in produced summaries. Our summarization methods significantly outperform advanced baselines, which verifies the necessity of incorporating textual and tabular data when summarizing report documents. We also conduct extensive comparative experiments to identify vital model components and configurations that can improve summarization results.
更多查看译文
关键词
Document summarization,natural language generation,natural language processing,text summarization
AI 理解论文
溯源树
样例
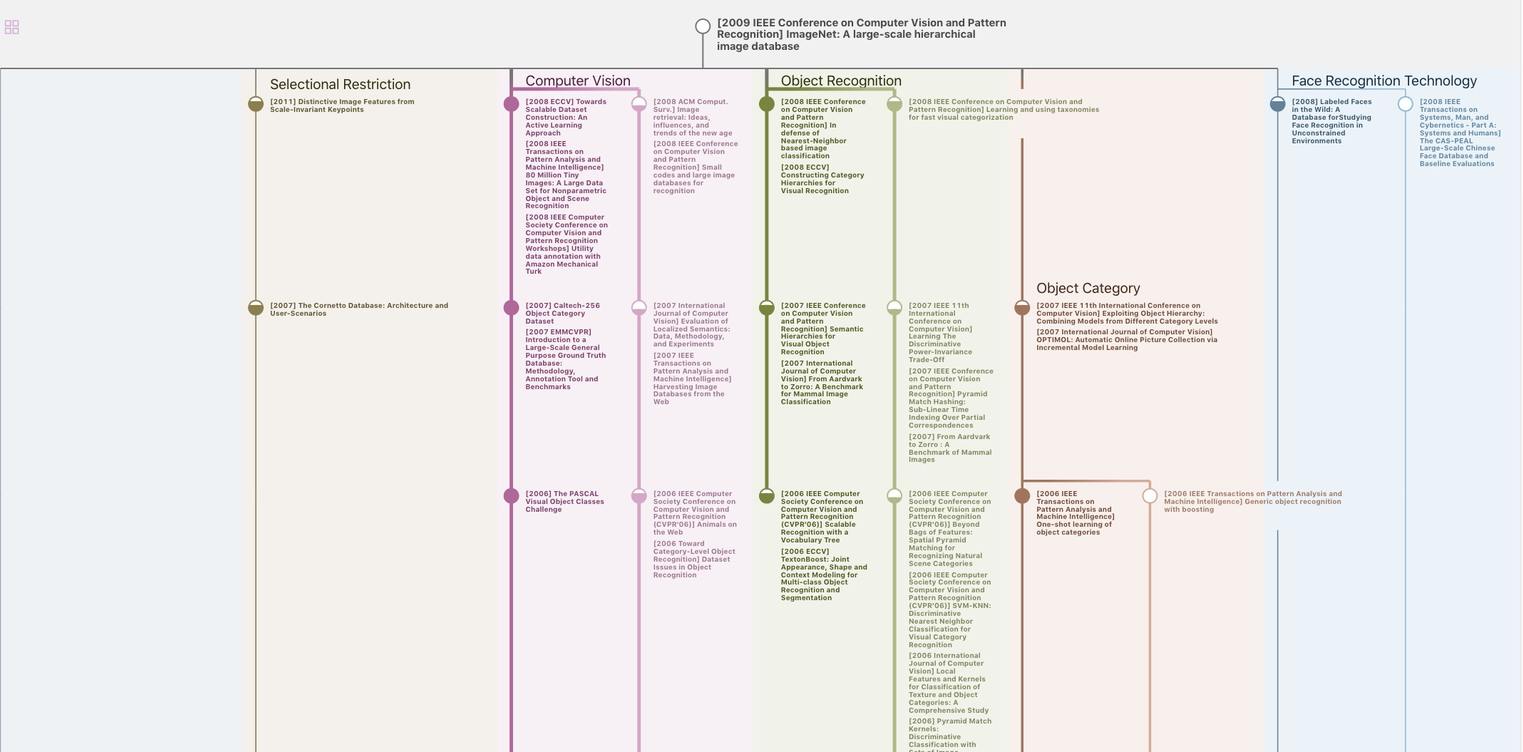
生成溯源树,研究论文发展脉络
Chat Paper
正在生成论文摘要