Online Modeling and Monitoring of Dependent Processes under Resource Constraints
arXiv (Cornell University)(2023)
摘要
Adaptive monitoring of a large population of dynamic processes is critical for the timely detection of abnormal events under limited resources in many healthcare and engineering systems. Examples include the risk-based disease screening and condition-based process monitoring. However, existing adaptive monitoring models either ignore the dependency among processes or overlook the uncertainty in process modeling. To design an optimal monitoring strategy that accurately monitors the processes with poor health conditions and actively collects information for uncertainty reduction, a novel online collaborative learning method is proposed in this study. The proposed method designs a collaborative learning-based upper confidence bound (CL-UCB) algorithm to optimally balance the exploitation and exploration of dependent processes under limited resources. Efficiency of the proposed method is demonstrated through theoretical analysis, simulation studies and an empirical study of adaptive cognitive monitoring in Alzheimer's disease.
更多查看译文
关键词
dependent processes,online modeling,monitoring
AI 理解论文
溯源树
样例
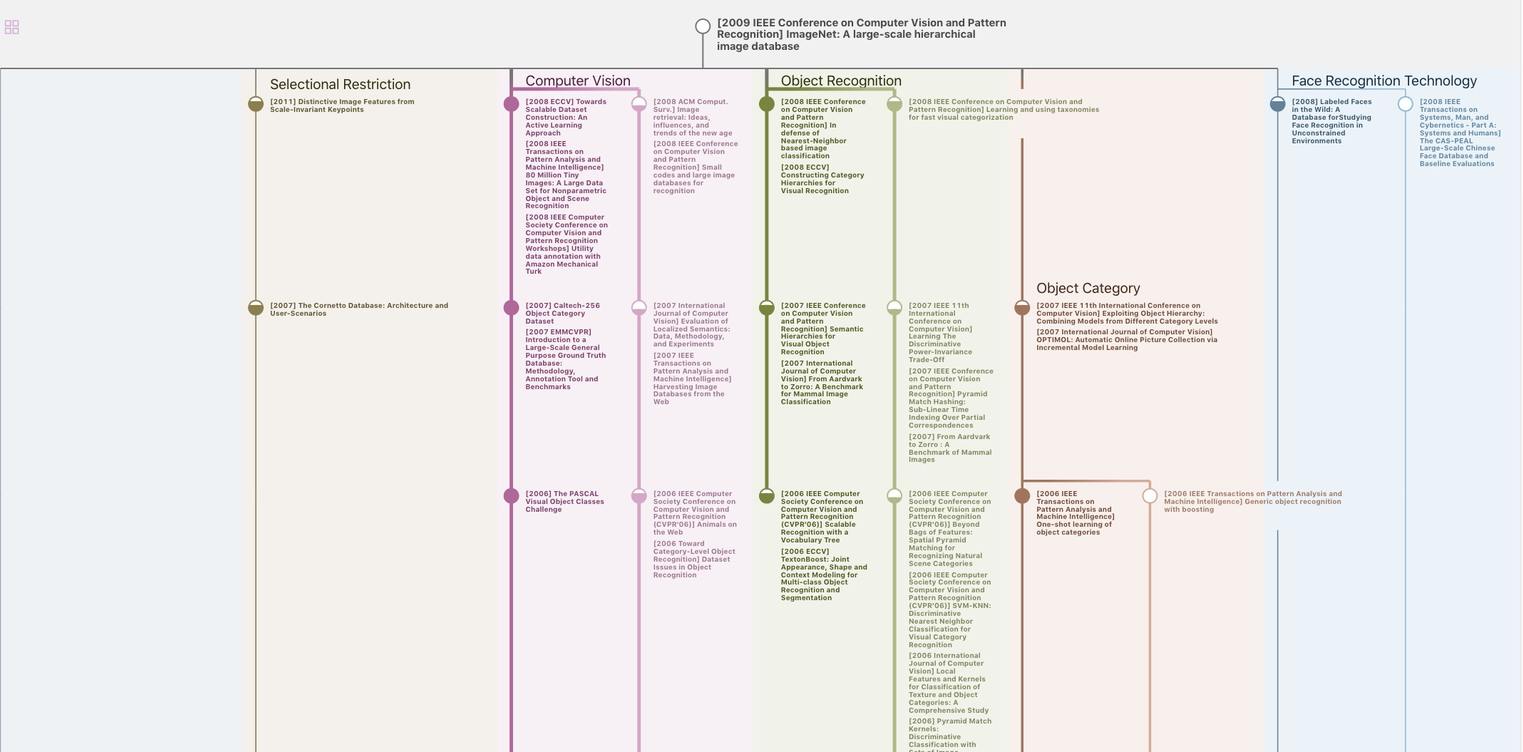
生成溯源树,研究论文发展脉络
Chat Paper
正在生成论文摘要