MTDAN: A Lightweight Multi-Scale Temporal Difference Attention Networks for Automated Video Depression Detection
IEEE Transactions on Affective Computing(2023)
摘要
Deep learning based video depression analysis has been recently an interesting and challenging topic. Most of existing works focus on learning single-scale facial dynamics of participants for depression detection. Besides, they usually adopt expensive deep learning models with high computational complexity, resulting in difficulty in real-time clinical applications. To address these two issues, this work proposes a lightweight Multi-scale Temporal Difference Attention Networks (MTDAN) integrating the temporal difference and attention mechanism to model both short-term and long-term temporal facial behaviors for automated video depression detection. Initially, two simple yet effective sub-branches, i.e., a Short-term Temporal Difference Attention Network (ST-TDAN), and a Long-term Temporal Difference Attention Network (LT-TDAN), are designed to perform individually short-term and long-term depressive behavior modeling. Then, a simple Interactive Multi-head Attention Fusion (IMHAF) strategy is employed for integrating short-term and long-term spatiotemporal features, followed by a linear fully-collected layer for depression score prediction. Experiments on two public AVEC2013 and AVEC2014 datasets show that our proposed method not only achieves highly competitive performance to state-of-the-art methods, but also has much smaller computational complexity than them on video depression detection tasks.
更多查看译文
关键词
Deep learning,video depression detection,temporal difference,attention,multi-scale,computational complexity
AI 理解论文
溯源树
样例
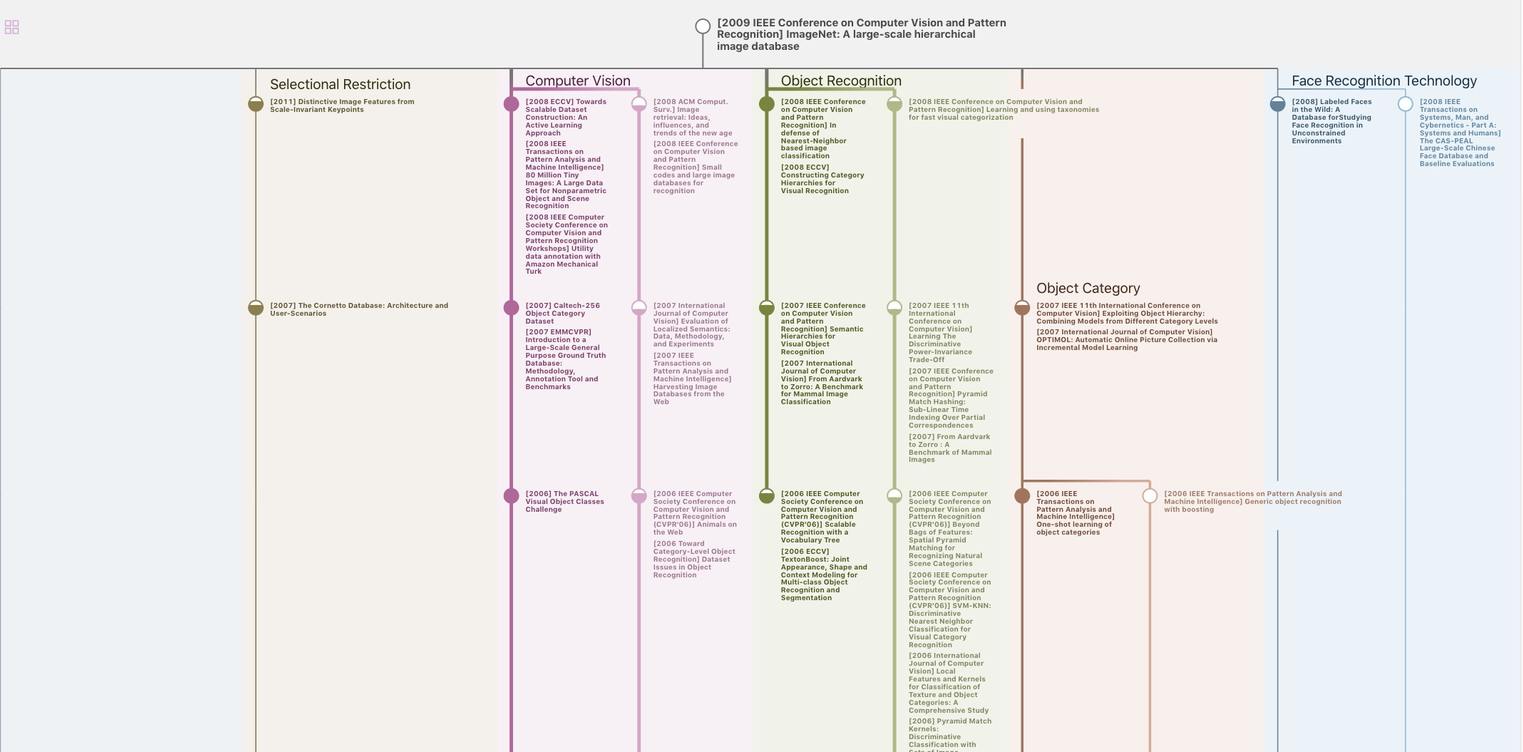
生成溯源树,研究论文发展脉络
Chat Paper
正在生成论文摘要