Error-robust and Label-efficient Deep Learning for Understanding Tumor Microenvironment from Spatial Transcriptomics
IEEE Transactions on Circuits and Systems for Video Technology(2023)
摘要
Spatial transcriptomics (ST) has become an important methodology in the analysis of the tumor microenvironment (TME) due to its ability to provide gene expression information with spatial resolution, enabling the identification and characterization of TME gene markers. Deep learning methods are proposed for analyzing spatial transcriptomic data for clustering the spatial regions of the TME based on gene expression. However, deep learning methods are often imposed by errors, which can impact the accuracy of gene expression quantification and TME gene identification. To address this issue, we propose a label-efficient method that utilizes curriculum learning and confidence learning to identify errors in graph deep learning when analyzing ST data. Our method explicitly incorporates the effect of noise in the learning process and employs probabilistic models or uncertainty estimates to represent the uncertainty in the data. Validated on human breast cancer ST data, we studied spatial gene expression in HER2-positive breast tumors using our method. The evaluation results suggest that the error quantification helps identify the noisy samples and subset the samples that results in more accurate gene expression quantification and TME gene identification. Additionally, there are biological insights obtained from the new subset formed by error samples. This error-robust deep learning method offers promising avenues for the analysis of spatial transcriptomic data, enabling accurate and label-efficient quantification of gene expression and identification of TME gene markers.
更多查看译文
关键词
Tumor Microenvironment,Spatial Transcriptomics,Gene Expression,Deep Learning,Error Quantification,Label Efficiency
AI 理解论文
溯源树
样例
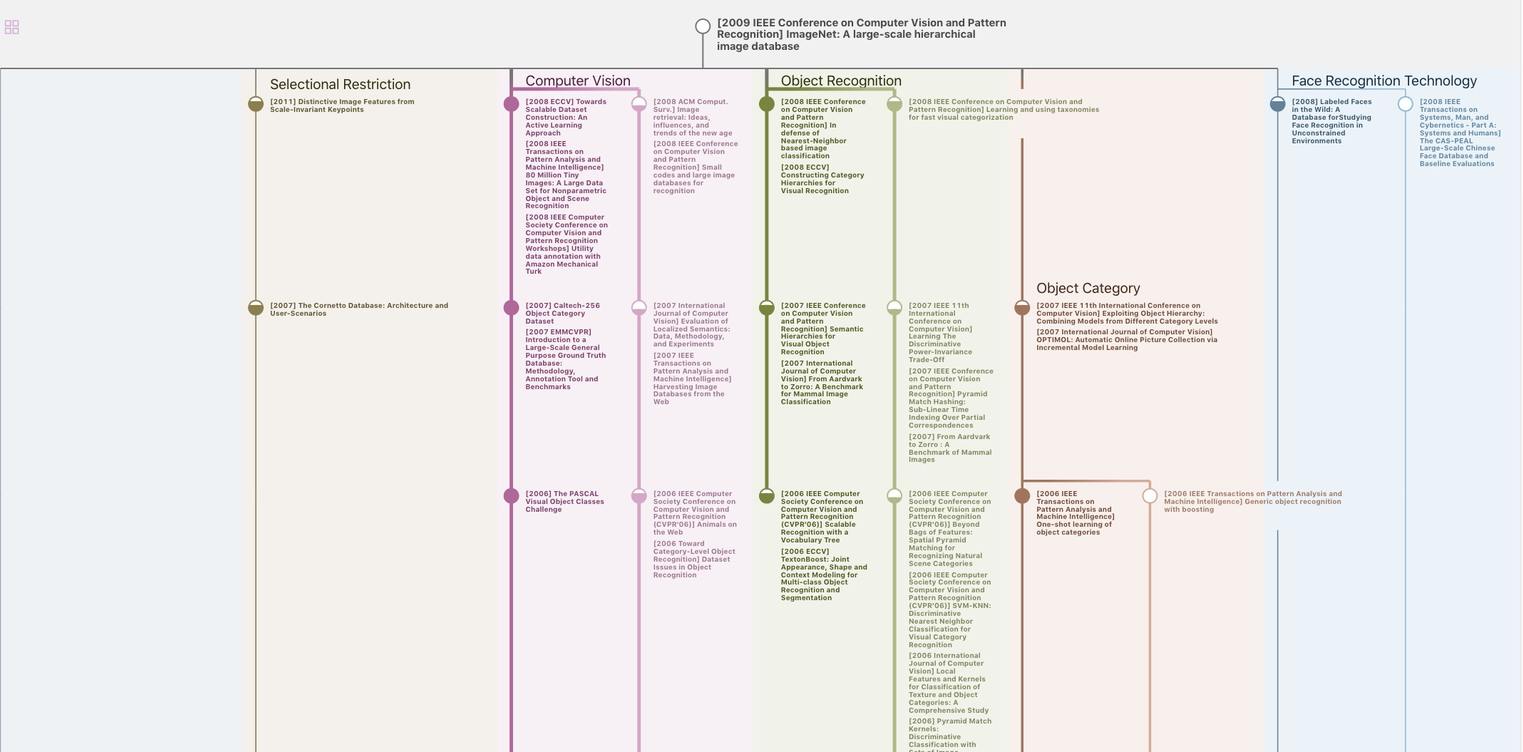
生成溯源树,研究论文发展脉络
Chat Paper
正在生成论文摘要