Exploring Rich Semantics for Open-Set Action Recognition
IEEE TRANSACTIONS ON MULTIMEDIA(2024)
摘要
Open-set action recognition (OSAR) aims to learn a recognition framework capable of both classifying known classes and identifying unknown actions in open-set scenarios. Existing OSAR methods typically reside in a data-driven paradigm, which ignore the rich semantics in both known and unknown categories. In fact, we humans have the capability of leveraging the captured semantic information, i.e., knowledge and experience, to incisively distinguish samples from known and unknown classes. Motivated by this observation, in this paper, we propose a Unified Semantic Exploration (USE) framework for recognizing actions in open-set scenarios. Specifically, we explore the explicit knowledge semantics by simulating the unknown classes with knowledge-guided virtual classes based on an external knowledge graph, which enables the model to simulate open-set perception during model training. Besides, we propose to learn the implicit data semantics by transferring the knowledge structure of action categories to the visual prototype space for semantic structure preservation. Extensive experiments on several action recognition benchmarks validate the effectiveness of our proposed method.
更多查看译文
关键词
Semantics,Prototypes,Knowledge graphs,Visualization,Task analysis,Uncertainty,Training,Open-set action recognition,video action recognition,semantic relation modeling
AI 理解论文
溯源树
样例
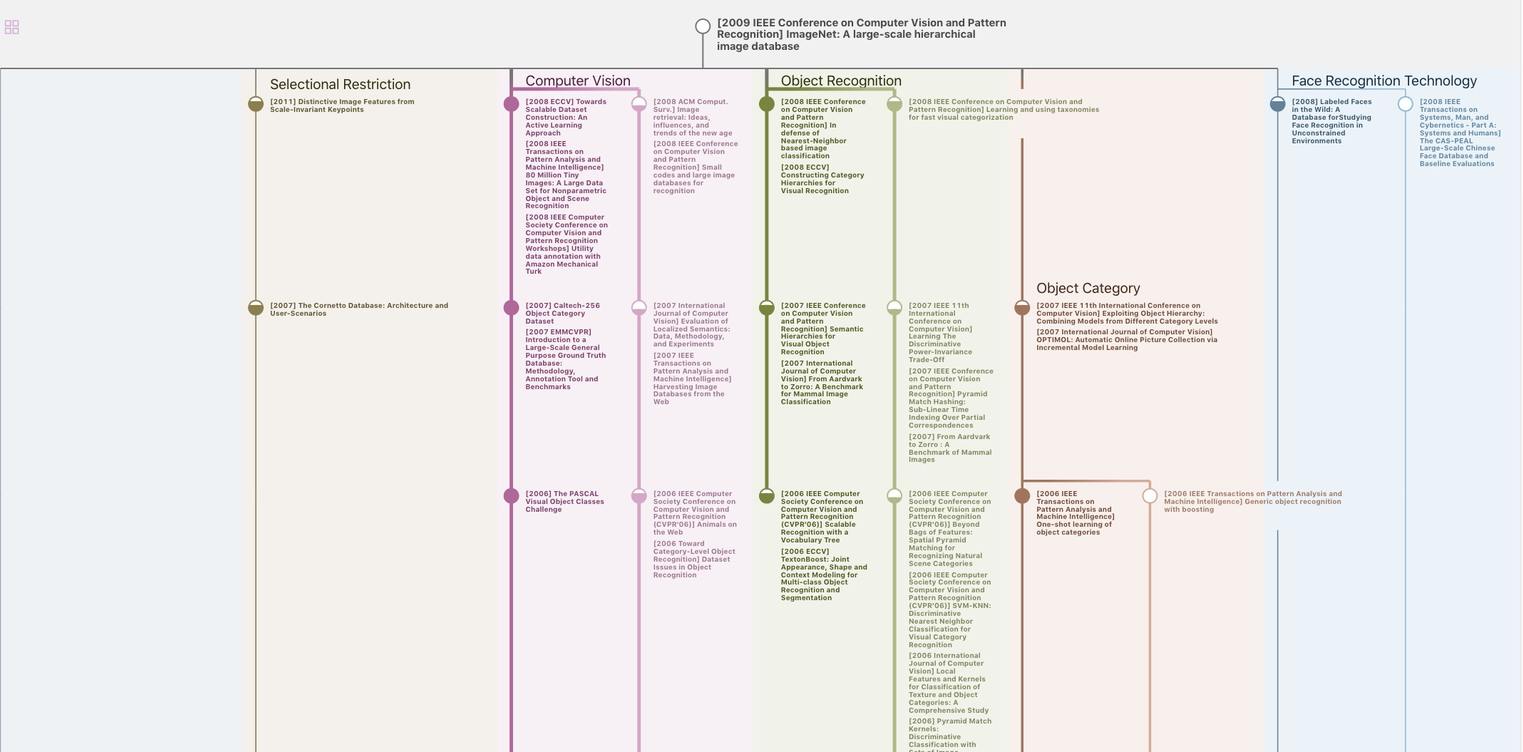
生成溯源树,研究论文发展脉络
Chat Paper
正在生成论文摘要