HIPODE: Enhancing Offline Reinforcement Learning with High-Quality Synthetic Data from a Policy-Decoupled Approach
arXiv (Cornell University)(2023)
摘要
Offline reinforcement learning (ORL) has gained attention as a means of training reinforcement learning models using pre-collected static data. To address the issue of limited data and improve downstream ORL performance, recent work has attempted to expand the dataset's coverage through data augmentation. However, most of these methods are tied to a specific policy (policy-dependent), where the generated data can only guarantee to support the current downstream ORL policy, limiting its usage scope on other downstream policies. Moreover, the quality of synthetic data is often not well-controlled, which limits the potential for further improving the downstream policy. To tackle these issues, we propose \textbf{HI}gh-quality \textbf{PO}licy-\textbf{DE}coupled~(HIPODE), a novel data augmentation method for ORL. On the one hand, HIPODE generates high-quality synthetic data by selecting states near the dataset distribution with potentially high value among candidate states using the negative sampling technique. On the other hand, HIPODE is policy-decoupled, thus can be used as a common plug-in method for any downstream ORL process. We conduct experiments on the widely studied TD3BC and CQL algorithms, and the results show that HIPODE outperforms the state-of-the-art policy-decoupled data augmentation method and most prevalent model-based ORL methods on D4RL benchmarks.
更多查看译文
关键词
offline reinforcement learning,reinforcement learning,synthetic data,high-quality,policy-decoupled
AI 理解论文
溯源树
样例
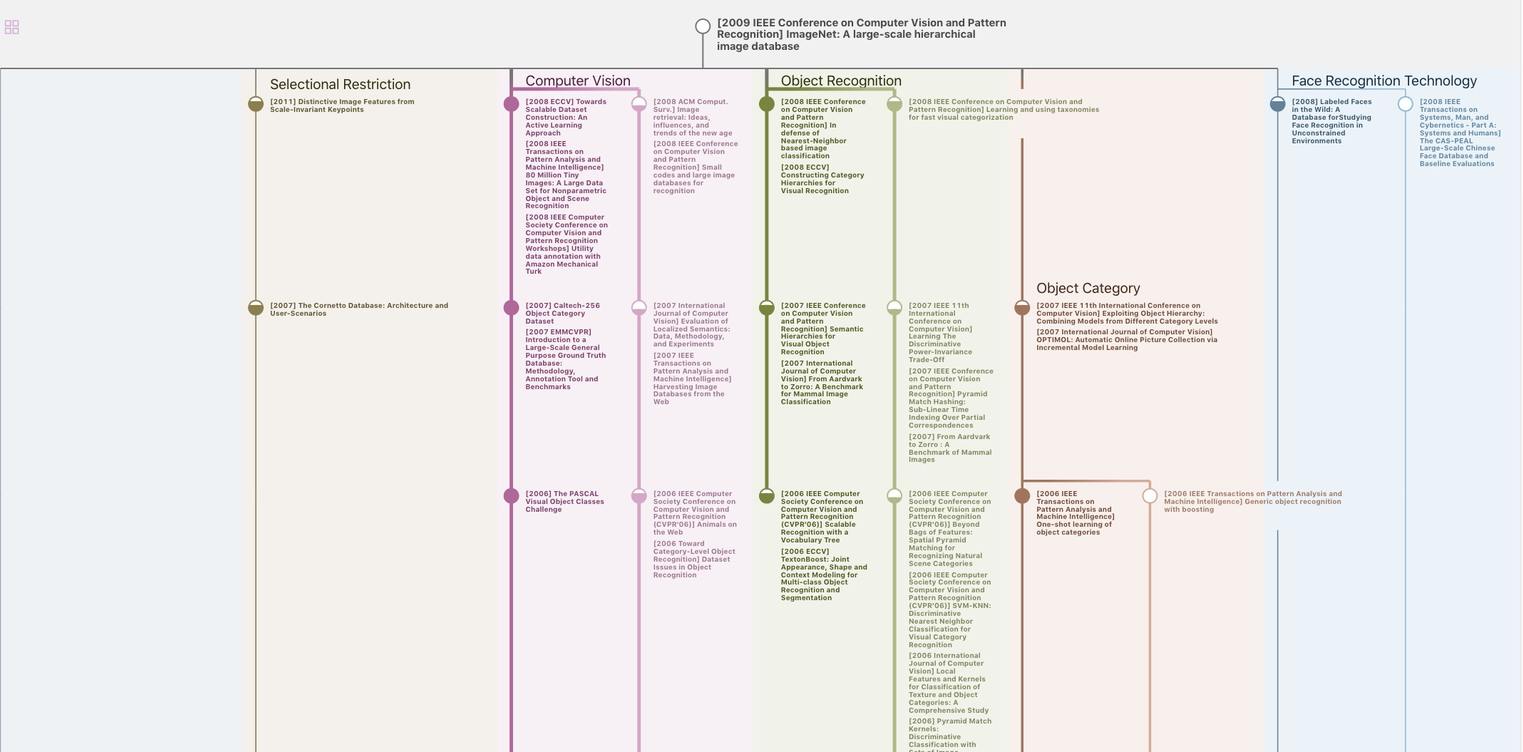
生成溯源树,研究论文发展脉络
Chat Paper
正在生成论文摘要