Class-Aware Dual-Supervised Aggregation Network for Video Object Detection.
IEEE TRANSACTIONS ON MULTIMEDIA(2024)
摘要
Video object detection has attracted increasing attention in recent years. Although great success has been achieved by off-the-shelf video object detection methods through delicately designing various types of feature aggregation, they overlook the class-aware supervision and thus still suffer from the problem of classification incapability, which means the classification between objects with deteriorated or similar appearances is error-prone. In this paper, we propose a novel class-aware dual-supervised aggregation network (CDANet) for video object detection, including three substantial improvements to effectively alleviate the classification incapability problem of previous methods. First, we develop a class-aware cross-modality distillation supervision that transfers the semantic knowledge of label data to the features of video data, effectively enhancing the semantic representations of features. Second, we design a graph-guided feature aggregation module that effectively models the structural relations between features by leveraging the dynamic residual graph convolutional network, enabling our CDANet to perform more effective feature aggregation in the temporal domain. Third, we present a class-aware proposal contrastive supervision to maximize the intra-class agreement and inter-class disagreement, which is conducive to improving the semantic discriminability of features. The class-aware dual supervision and feature aggregation are tightly tied into a unified end-to-end framework to make our CDANet fully exploit class-specific semantic knowledge and inter-frame temporal dependencies to enhance object appearance representations, which facilitates the classification of detected objects. We conduct experiments on the challenging ImageNet VID dataset, and the results demonstrate the superiority of our CDANet against state-of-the-art methods. More remarkably, our CDANet achieves 85.4% mAP with ResNet-101 or 86.5% mAP with ResNeXt-101.
更多查看译文
关键词
Video object detection,distillation supervision,graph-guided feature aggregation,contrastive supervision
AI 理解论文
溯源树
样例
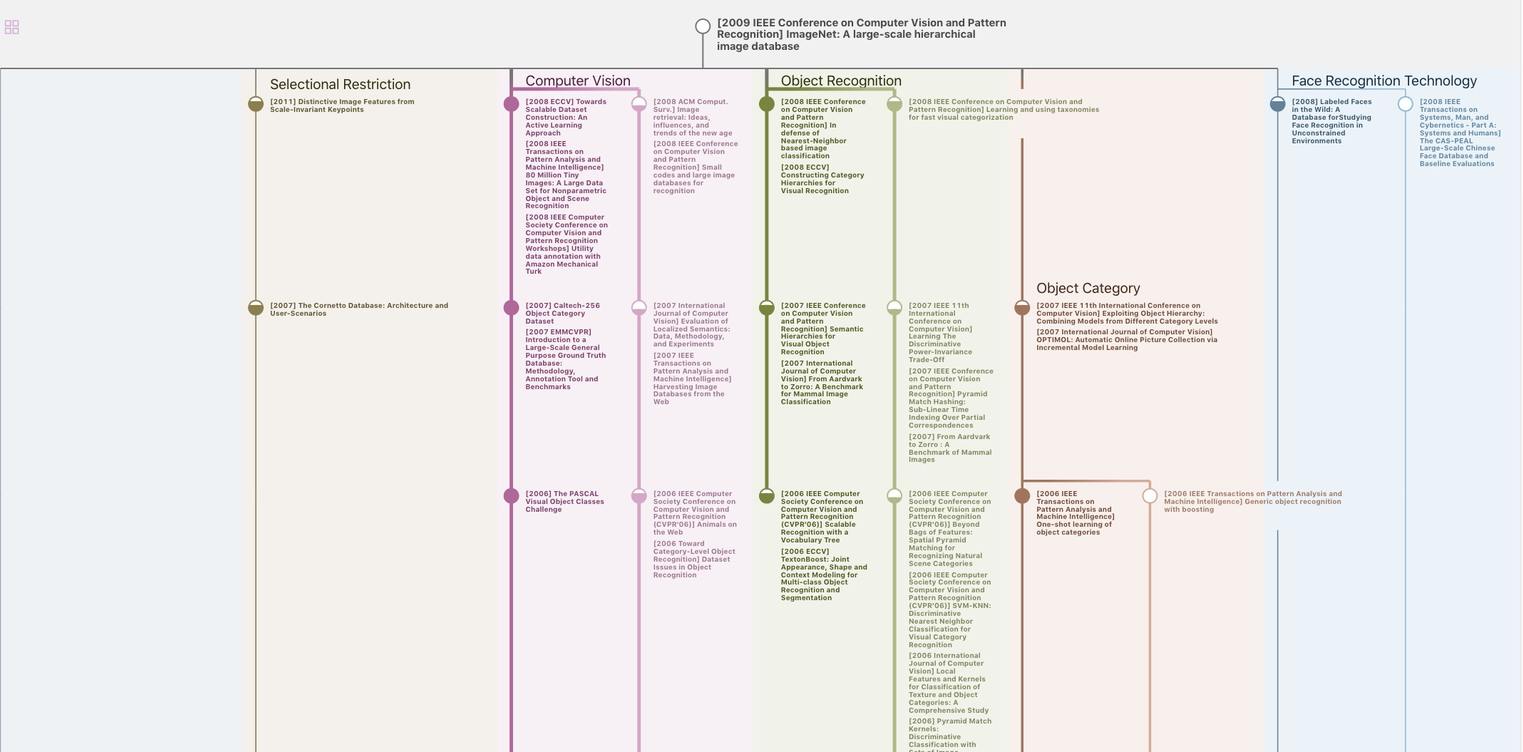
生成溯源树,研究论文发展脉络
Chat Paper
正在生成论文摘要