COMBHelper: A Neural Approach to Reduce Search Space for Graph Combinatorial Problems
Proceedings of the AAAI Conference on Artificial Intelligence(2024)
摘要
Combinatorial Optimization (CO) problems over graphs appear routinely in manyapplications such as in optimizing traffic, viral marketing in social networks,and matching for job allocation. Due to their combinatorial nature, theseproblems are often NP-hard. Existing approximation algorithms and heuristicsrely on the search space to find the solutions and become time-consuming whenthis space is large. In this paper, we design a neural method called COMBHelperto reduce this space and thus improve the efficiency of the traditional COalgorithms based on node selection. Specifically, it employs a Graph NeuralNetwork (GNN) to identify promising nodes for the solution set. This prunedsearch space is then fed to the traditional CO algorithms. COMBHelper also usesa Knowledge Distillation (KD) module and a problem-specific boosting module tobring further efficiency and efficacy. Our extensive experiments show that thetraditional CO algorithms with COMBHelper are at least 2 times faster thantheir original versions.
更多查看译文
AI 理解论文
溯源树
样例
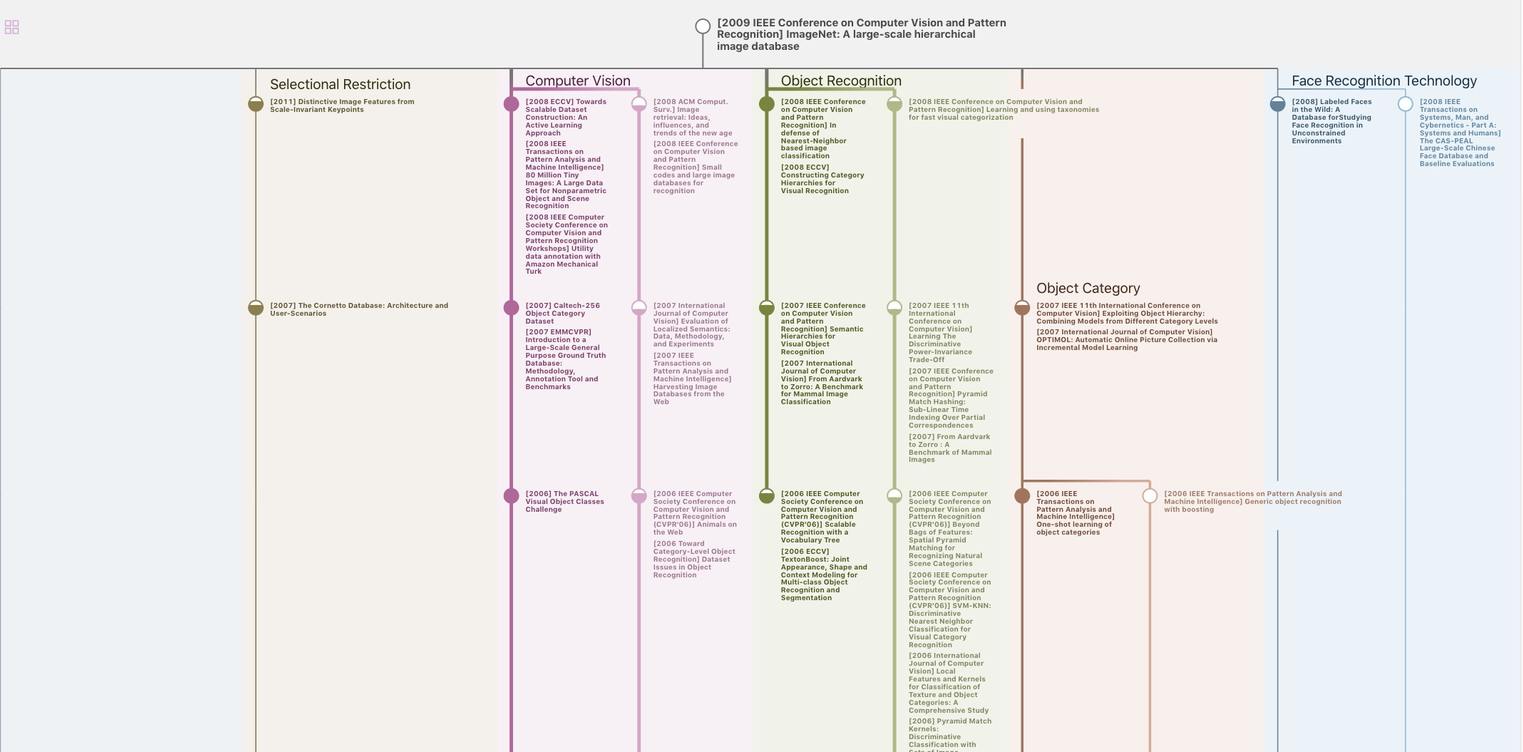
生成溯源树,研究论文发展脉络
Chat Paper
正在生成论文摘要