GTAN: graph-based tracklet association network for multi-object tracking
Neural Computing and Applications(2024)
摘要
Multi-object tracking (MOT) is a thriving research field in computer vision. The tracklet-based MOT frameworks are frequently employed to generate long and stable trajectories in work scenes that involve long-term occlusion. However, most of these methods train tracklet feature encoders using complex loss functions, lacking an end-to-end paradigm guided by association results, which ultimately leads to limited MOT performance. To address this issue, a graph-based tracklet association framework that seamlessly integrates tracklet feature learning with tracklet association, thereby achieving tracklet association in an end-to-end manner. Specifically, we perform tracklet-based MOT in the graph domain and transform the tracklet association problem into an edge classification task. A message passing network (MPN) is used to update the tracklet features globally, which enhances the robustness of the tracklet features. Additionally, an attention-based feature update function is proposed to ensure the significance of current object. The effectiveness of the proposed framework is demonstrated using MOT17 and MOT20 benchmark datasets, and the experimental results show that the graph-based tracklet association network is a model-independent and plug-and-play component that could combine with different frame-based trackers to boost the MOT performance significantly.
更多查看译文
关键词
Multi-object tracking, Tracklet association, Transformer,Graph neural network
AI 理解论文
溯源树
样例
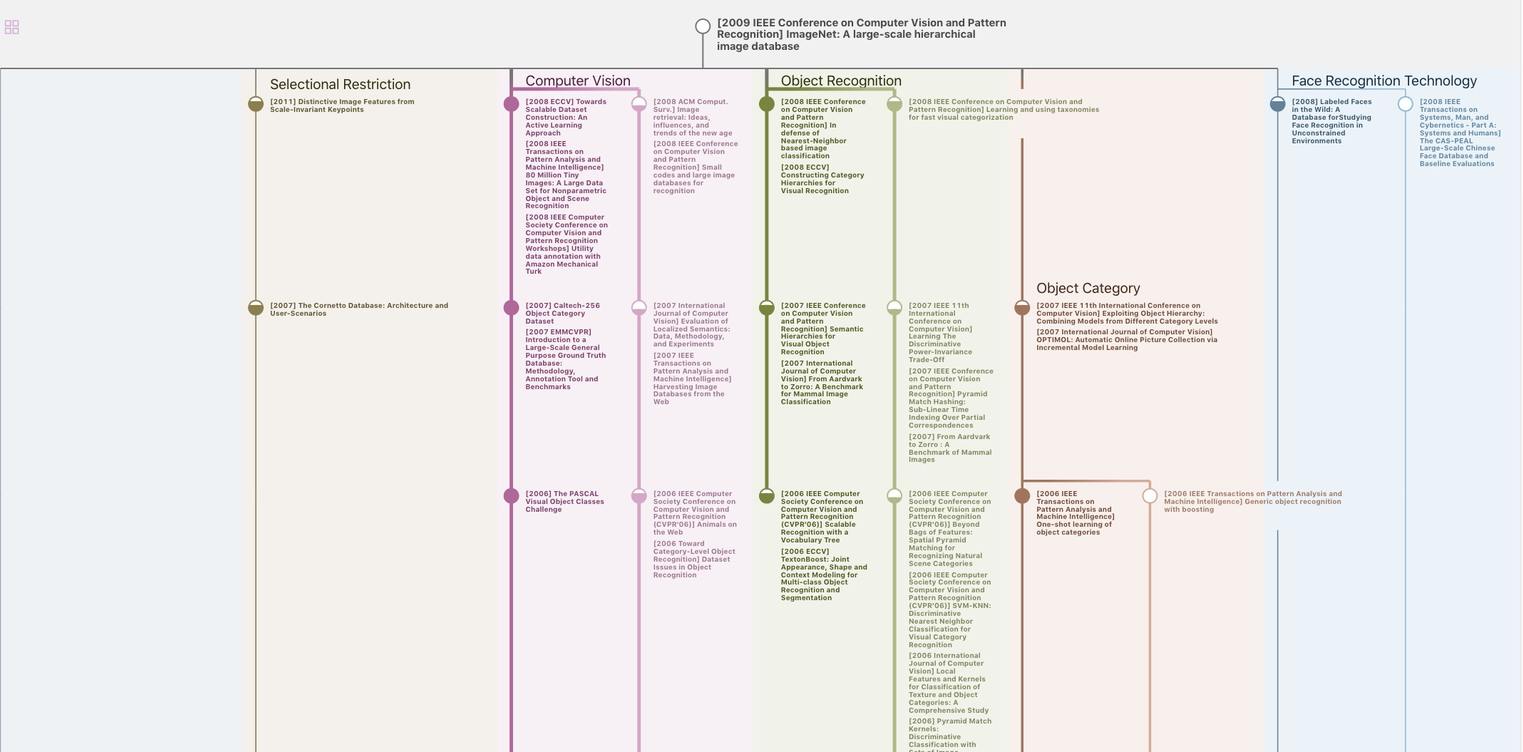
生成溯源树,研究论文发展脉络
Chat Paper
正在生成论文摘要