ASE-Net for Segmentation of Post-Operative Glioblastoma and Patient-Specific Fine-Tuning for Segmentation Refinement of Follow-Up MRI Scans
SN Computer Science(2023)
摘要
Volumetric quantification of tumors is usually done manually by radiologists requiring precious medical time and suffering from inter-observer variability. An automatic tool for accurate volume quantification of post-operative glioblastoma would reduce the workload of radiologists and improve the quality of follow-up monitoring and patient care. This paper deals with the 3-D segmentation of post-operative glioblastoma using channel squeeze and excitation based attention gated network (ASE-Net). The proposed deep neural network has a 3-D encoder and decoder based architecture with channel squeeze and excitation (CSE) blocks and attention blocks. The CSE block reduces the dependency on space information and put more emphasize on the channel information. The attention block suppresses the feature maps of irrelevant background and helps highlighting the relevant feature maps. The Uppsala university data set used has post-operative follow-up MRI scans for fifteen patients. A patient specific fine-tuning approach is used to improve the segmentation results for each patient. ASE-Net is also cross-validated with BraTS-2021 data set. The mean dice score of five-fold cross validation results with BraTS-2021 data set for enhanced tumor is 0.8244. The proposed network outperforms the competing networks like U-Net, Attention U-Net and Res U-Net. On the Uppsala University glioblastoma data set, the mean Dice score obtained with the proposed network is 0.7084, Hausdorff Distance-95 is 7.14 and the mean volumetric similarity achieved is 0.8579. With fine-tuning the pre-trained network, the mean dice score improved to 0.7368, Hausdorff Distance-95 decreased to 6.10 and volumetric similarity improved to 0.8736. ASE-Net outperforms the competing networks and can be used for volumetric quantification of post-operative glioblastoma from follow-up MRI scans. The network significantly reduces the probability of over segmentation.
更多查看译文
关键词
Post-operative glioblastoma, MRI scans, ASE-Net, Volumetric quantification, Patient-specific fine-tuning
AI 理解论文
溯源树
样例
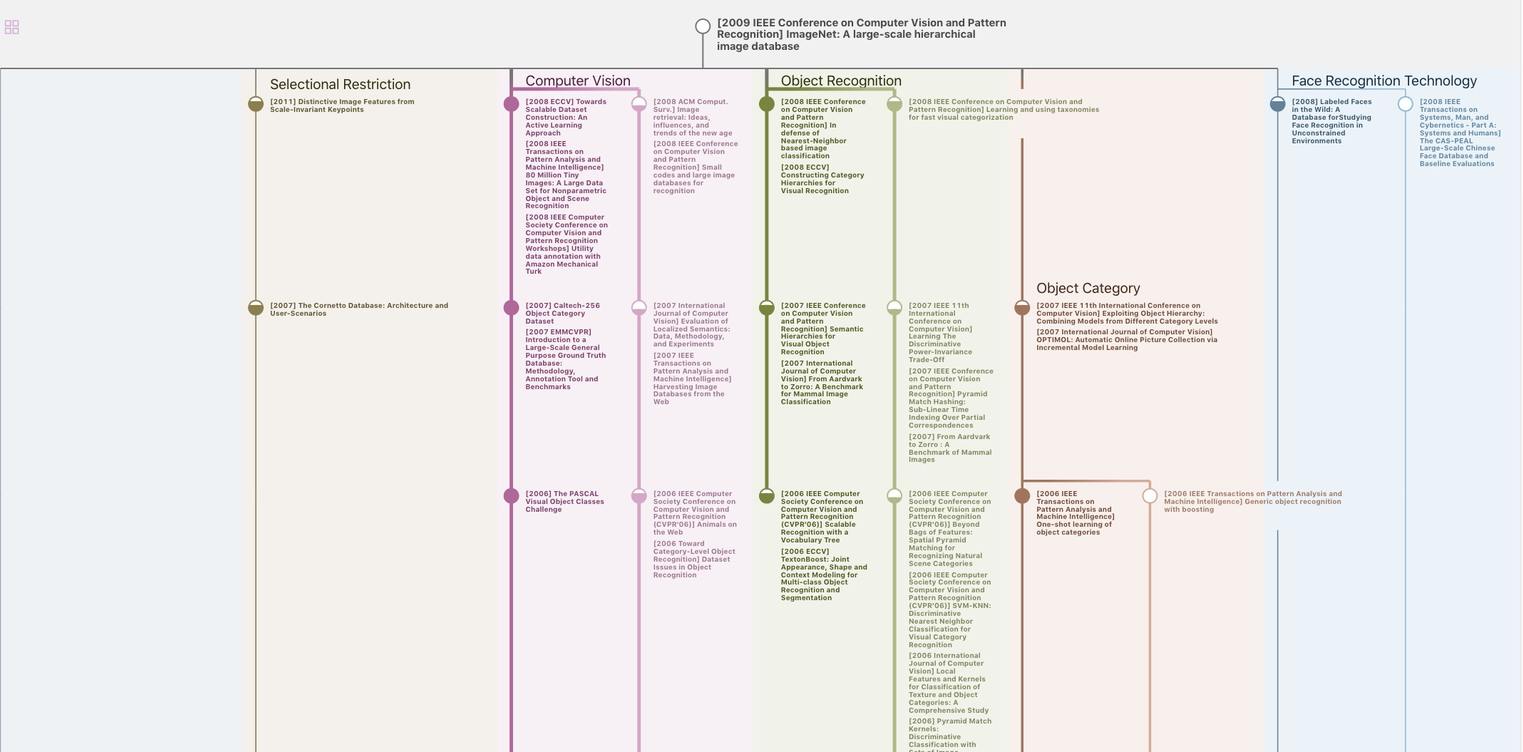
生成溯源树,研究论文发展脉络
Chat Paper
正在生成论文摘要