Adapt and explore: Multimodal mixup for representation learning
INFORMATION FUSION(2024)
摘要
Research on general multimodal systems has gained significant attention due to the proliferation of multimodal data in the real world. Despite the remarkable performance achieved by existing multimodal representation learning schemes, missing modalities remain a persistent issue, thereby limiting the overall applicability of multimodal systems. Intending to address the issue, we propose a novel approach named M3ixup (Multi -Modal Mixup), which leverages the mixup strategy to improve unimodal and multimodal representation learning while simultaneously increasing robustness against missing modalities. First, we adopt productive multimodal learning scheme to model representations with modality -specific and joint -modality encoders. The general scheme ensuring the proposed approach transferable for various multimodal learning scenarios, including supervised, unsupervised, and reinforcement learning. Then, the unimodal input and manifold mixup is used to enhance the modality -specific encoders to capture intra-modal dynamics. Next, we present multimodal mixup to mix different modalities and generate mixed multimodal representations in adapting and exploring steps. The former step aims at bridging the huge information gaps between unimodal and multimodal representations in the joint space in the alignment, while the latter step further captures the inter -modal dynamics and exploits the non-linear relationships among different modalities. After that, the mixed views are aligned with the original multimodal representations by contrastive learning. Additionally, we innovatively extend the mixup strategy to the loss function of multimodal contrastive learning in two steps to improve the alignment between mixed and original views. Extensive experiments on public datasets in various multimodal learning scenarios demonstrate the superiority of the proposed M3ixup. The codes are available at https://github.com/RH-Lin/m3ixup.
更多查看译文
关键词
Unimodal and multimodal mixup,Multimodal representation learning,Multimodal contrastive learning,Missing modality issues,Various multimodal learning scenarios
AI 理解论文
溯源树
样例
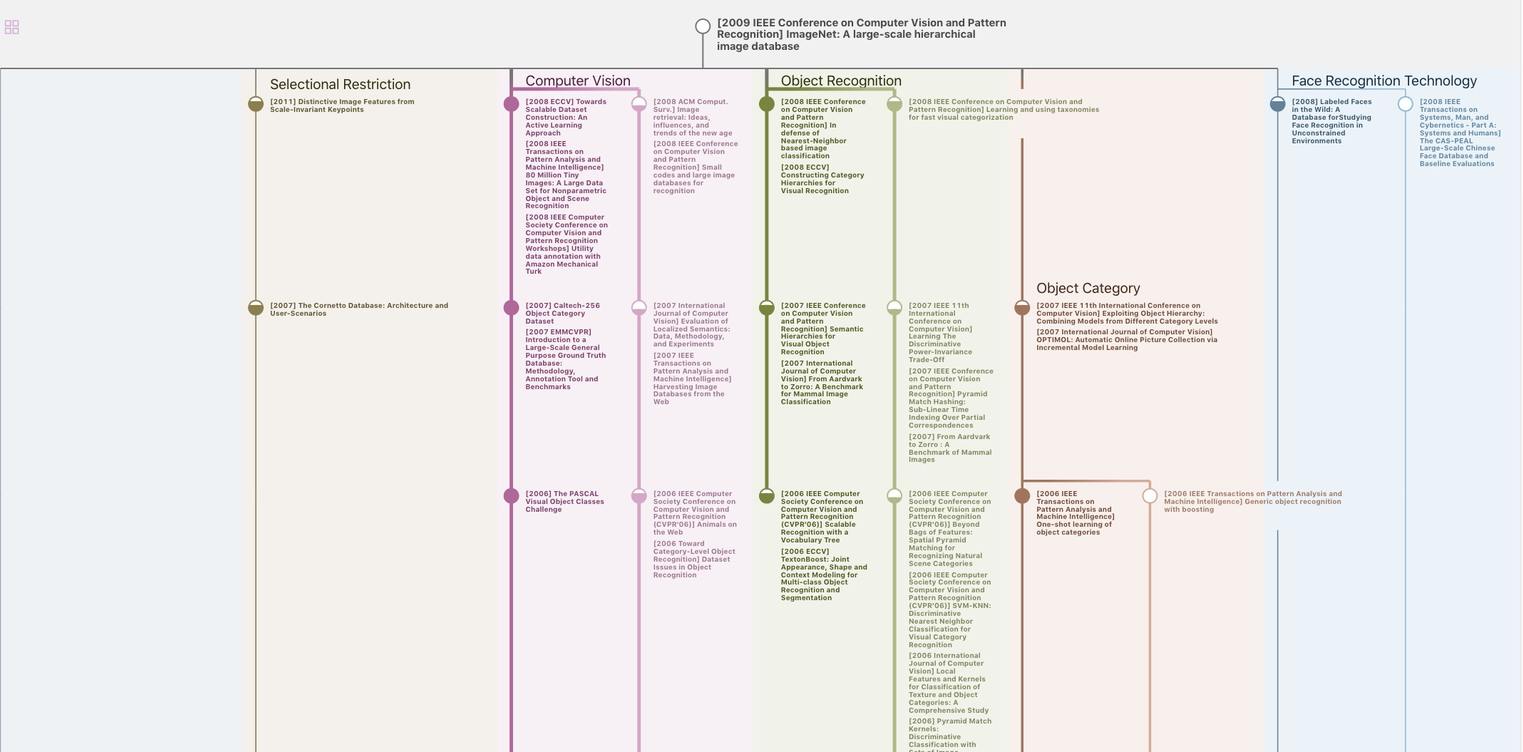
生成溯源树,研究论文发展脉络
Chat Paper
正在生成论文摘要