Safety and Performance, Why Not Both? Bi-Objective Optimized Model Compression Against Heterogeneous Attacks Toward AI Software Deployment
IEEE TRANSACTIONS ON SOFTWARE ENGINEERING(2024)
摘要
The size of deep learning models in artificial intelligence (AI) software is increasing rapidly, hindering the large-scale deployment on resource-restricted devices (e.g., smartphones). To mitigate this issue, AI software compression plays a crucial role, which aims to compress model size while keeping high performance. However, the intrinsic defects in a big model may be inherited by the compressed one. Such defects may be easily leveraged by adversaries, since a compressed model is usually deployed in a large number of devices without adequate protection. In this article, we aim to address the safe model compression problem from the perspective of safety-performance co-optimization. Specifically, inspired by the test-driven development (TDD) paradigm in software engineering, we propose a test-driven sparse training framework called SafeCompress. By simulating the attack mechanism as safety testing, SafeCompress can automatically compress a big model to a small one following the dynamic sparse training paradigm. Then, considering two kinds of representative and heterogeneous attack mechanisms, i.e., black-box membership inference attack and white-box membership inference attack, we develop two concrete instances called BMIA-SafeCompress and WMIA-SafeCompress. Further, we implement another instance called MMIA-SafeCompress by extending SafeCompress to defend against the occasion when adversaries conduct black-box and white-box membership inference attacks simultaneously. We conduct extensive experiments on five datasets for both computer vision and natural language processing tasks. The results show the effectiveness and generalizability of our framework. We also discuss how to adapt SafeCompress to other attacks besides membership inference attack, demonstrating the flexibility of SafeCompress.
更多查看译文
关键词
AI software safe compression,test-driven development,heterogeneous membership inference attack
AI 理解论文
溯源树
样例
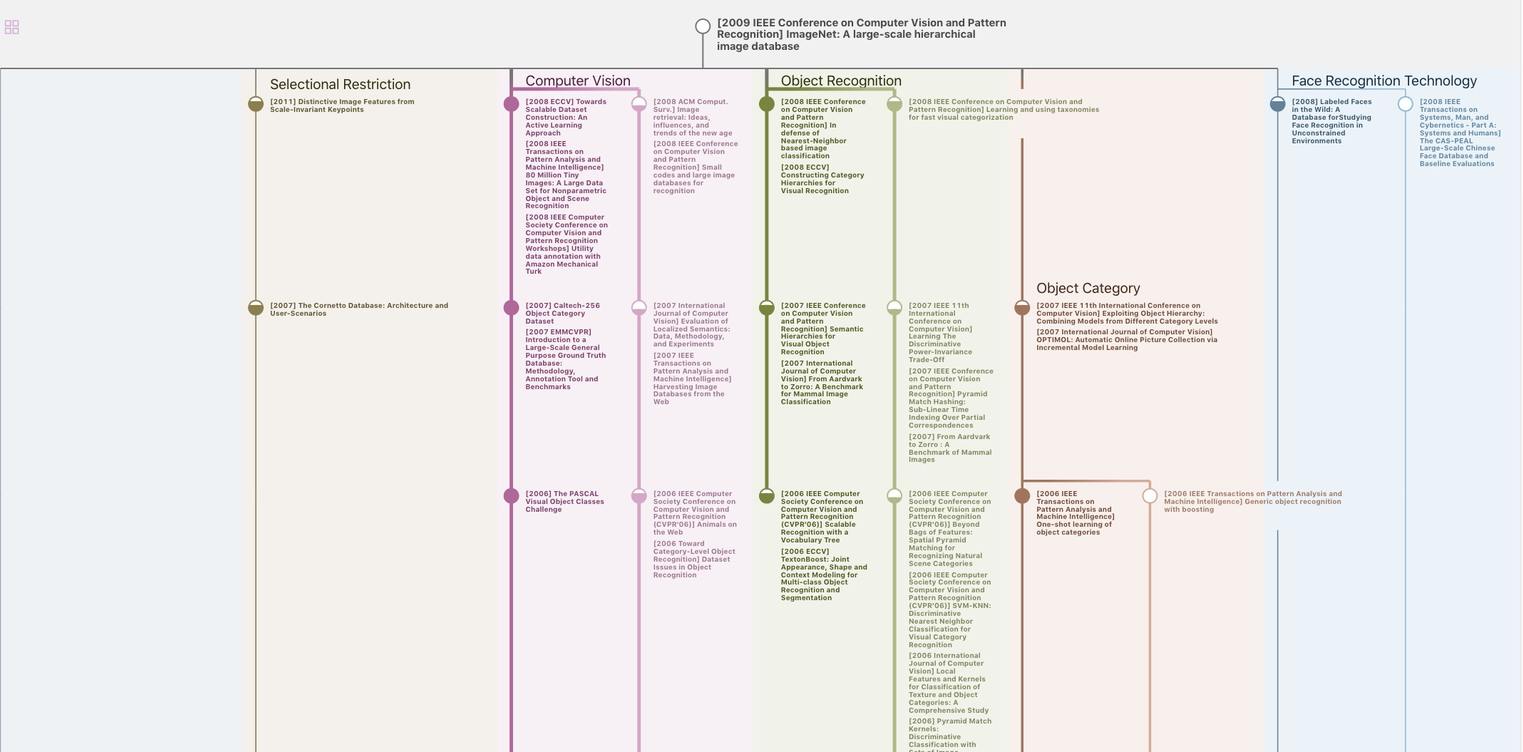
生成溯源树,研究论文发展脉络
Chat Paper
正在生成论文摘要