Task Oriented Dialogue As a Catalyst for Self-Supervised Automatic Speech Recognition
IEEE International Conference on Acoustics, Speech, and Signal Processing(2024)
摘要
While word error rates of automatic speech recognition (ASR) systems haveconsistently fallen, natural language understanding (NLU) applications built ontop of ASR systems still attribute significant numbers of failures tolow-quality speech recognition results. Existing assistant systems collectlarge numbers of these unsuccessful interactions, but these systems usuallyfail to learn from these interactions, even in an offline fashion. In thiswork, we introduce CLC: Contrastive Learning for Conversations, a family ofmethods for contrastive fine-tuning of models in a self-supervised fashion,making use of easily detectable artifacts in unsuccessful conversations withassistants. We demonstrate that our CLC family of approaches can improve theperformance of ASR models on OD3, a new public large-scale semi-syntheticmeta-dataset of audio task-oriented dialogues, by up to 19.2transfer to real-world systems as well, where we show that CLC can help toimprove performance by up to 6.7available at https://github.com/amazon-science/amazon-od3 .
更多查看译文
关键词
Task Oriented Dialogue,Automatic Speech Recognition,Self-Supervised Learning
AI 理解论文
溯源树
样例
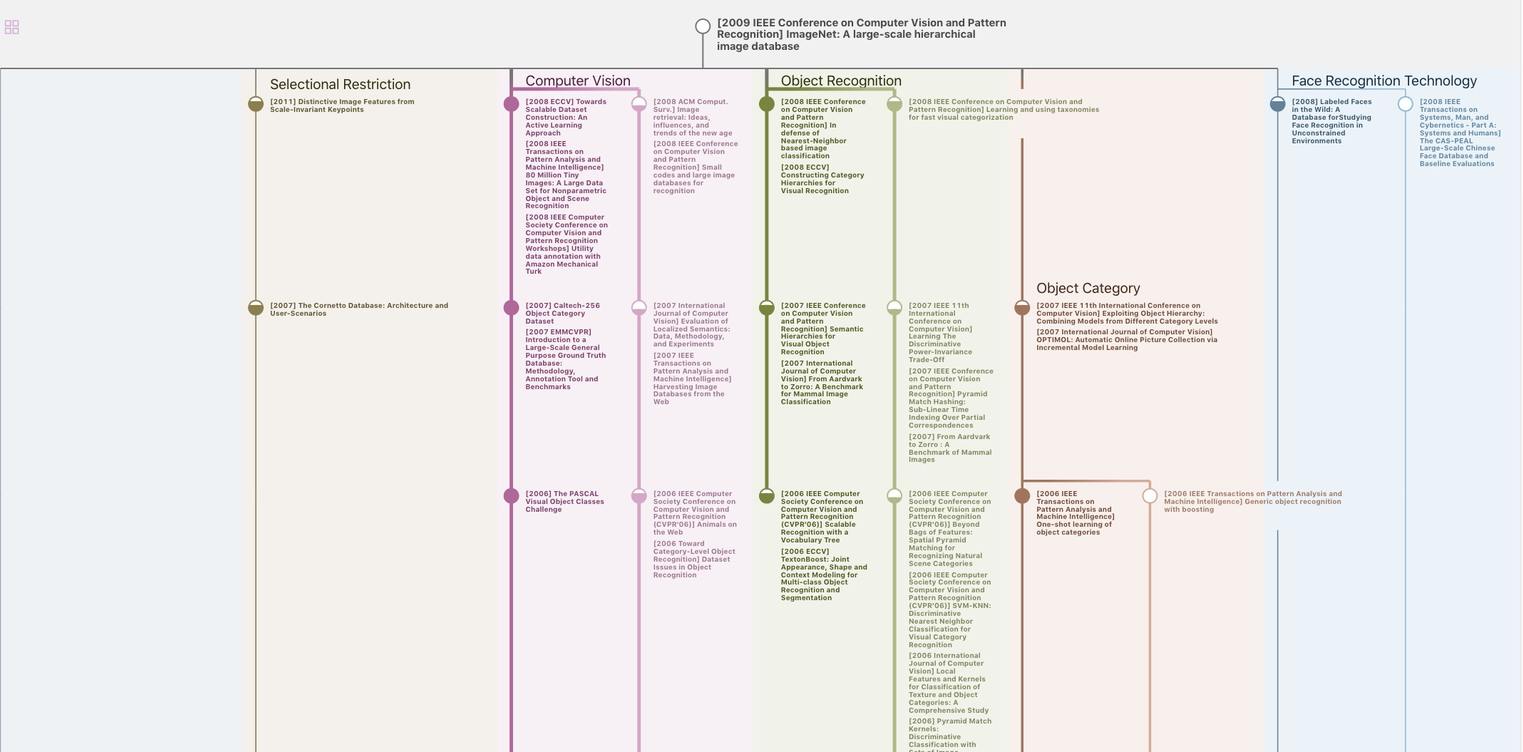
生成溯源树,研究论文发展脉络
Chat Paper
正在生成论文摘要