Revisiting Silhouette Aggregation
arxiv(2024)
摘要
Silhouette coefficient is an established internal clustering evaluation
measure that produces a score per data point, assessing the quality of its
clustering assignment. To assess the quality of the clustering of the whole
dataset, the scores of all the points in the dataset are typically (micro)
averaged into a single value. An alternative path, however, that is rarely
employed, is to average first at the cluster level and then (macro) average
across clusters. As we illustrate in this work with a synthetic example, the
typical micro-averaging strategy is sensitive to cluster imbalance while the
overlooked macro-averaging strategy is far more robust. By investigating
macro-Silhouette further, we find that uniform sub-sampling, the only available
strategy in existing libraries, harms the measure's robustness against
imbalance. We address this issue by proposing a per-cluster sampling method. An
experimental study on eight real-world datasets is then used to analyse both
coefficients in two clustering tasks.
更多查看译文
AI 理解论文
溯源树
样例
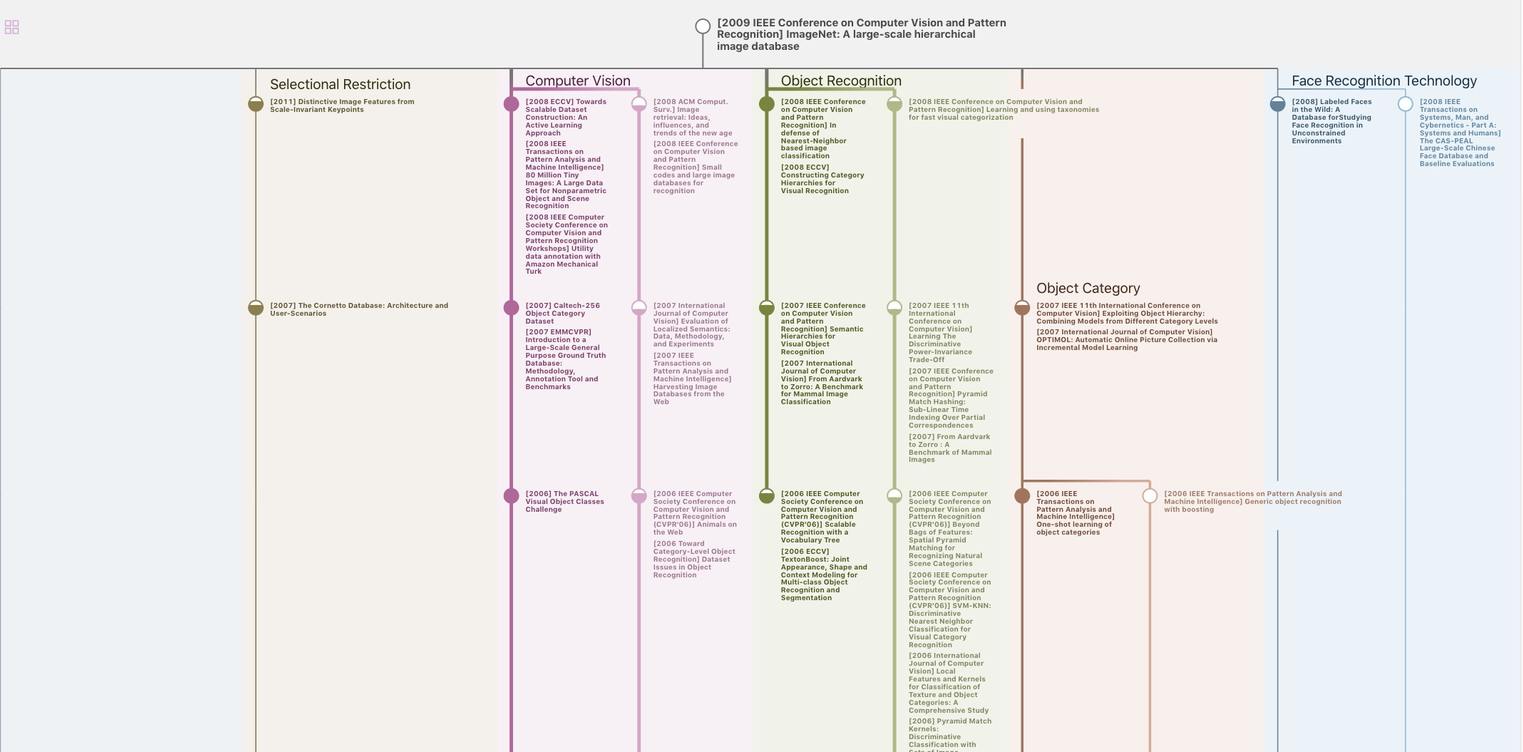
生成溯源树,研究论文发展脉络
Chat Paper
正在生成论文摘要