An Effective Dynamic Reweighting Method for Unbiased Scene Graph Generation
PATTERN RECOGNITION AND COMPUTER VISION, PRCV 2023, PT I(2024)
摘要
Despite the remarkable advancements in Scene Graph Generation (SGG) in recent years, the precise capture and modeling of longtail object relationships remain persistent challenges in the field. Conventional methods generally employ resampling and reweighting techniques to achieve unbiased predictions. Existing reweighting methods in SGG calculate weights based on the class distribution of the dataset. And they focus on the reweighting of the related samples while overlooking the reweighting of the samples whose objects are unrelated. However, the sample distribution during the training process is inconsistent with the class distribution of the dataset, and the reweighting of samples whose objects are unrelated should not be overlooked. In this paper, we propose a novel method named Dynamic Reweighting based on the Sample Distribution (DRSD). The DRSD method calculates the weights of classes based on the sample distribution during the training process and incorporates reweighting for the samples whose objects are unrelated. Specifically, we utilize a sample queue mechanism to record and update the sample distribution and introduce a transition mechanism to ensure training stability. The experiments conducted on the Visual Genome dataset demonstrate the effectiveness of our method. Our method exhibits model-agnostic characteristics and yields significant performance improvements on three benchmark models (Motif, VCTree, and Transformer). Specifically, it achieves an increase of 23.4%, 25.1%, and 27.6% on the mR@100 metric for the Predicate Classification task, achieving 40.9%, 41.2%, and 43.4%, respectively. Moreover, our method outperforms the state-of-the-art reweighting method in SGG, i.e. FGPL, by 3%.
更多查看译文
关键词
Scene graph generation,Reweighting method,Long-tail
AI 理解论文
溯源树
样例
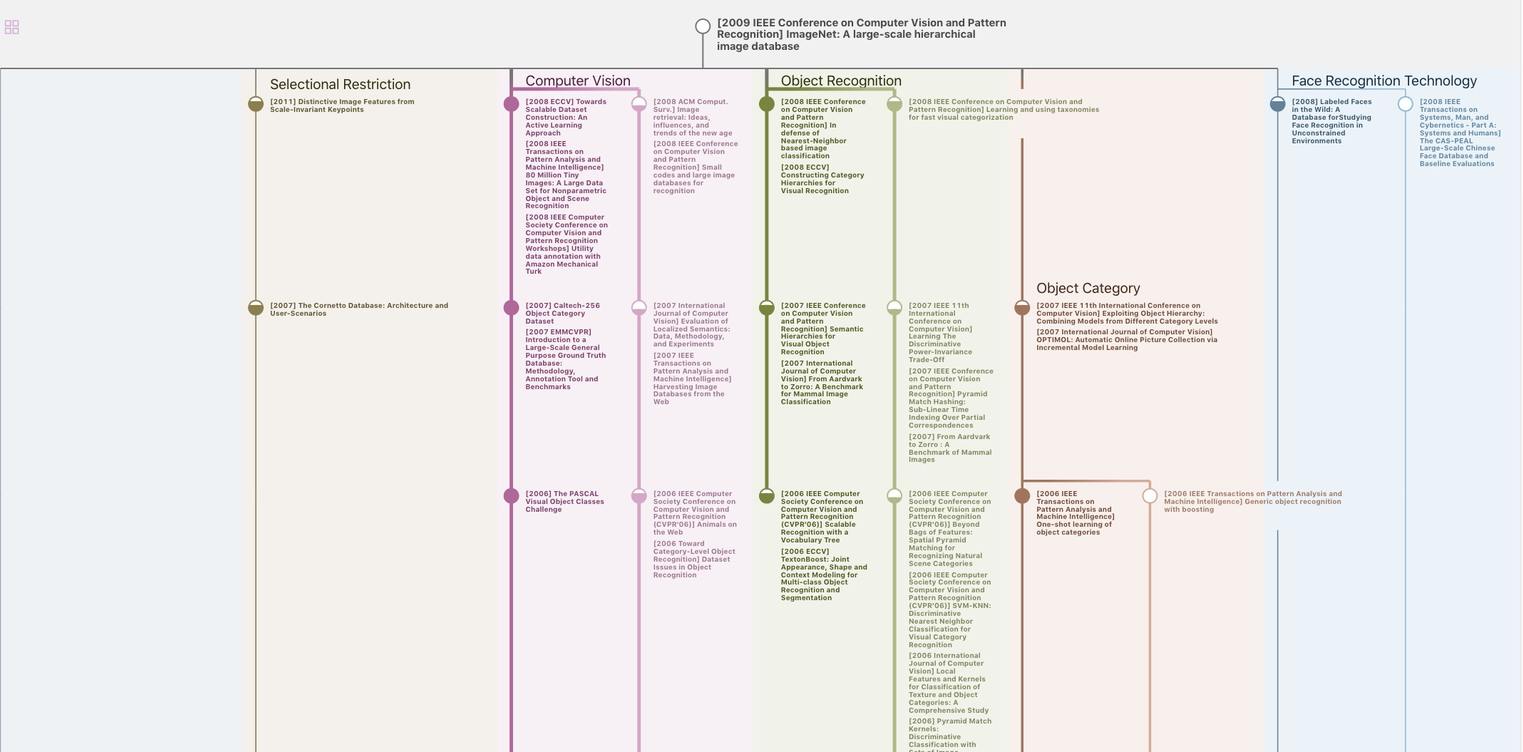
生成溯源树,研究论文发展脉络
Chat Paper
正在生成论文摘要