An Automatic Depression Detection Method with Cross-Modal Fusion Network and Multi-head Attention Mechanism
PATTERN RECOGNITION AND COMPUTER VISION, PRCV 2023, PT V(2024)
摘要
Audio-visual based multimodal depression detection has gained significant attention due to its high efficiency and convenience as a computer-aided detection tool, resulting in promising performance. In this paper, we propose a cross-modal fusion network based on multi-head attention and residual structures (CMAFN) for depression recognition. CMAFN consists of three core modules: the Local Temporal Feature Extract Block (LTF), the Cross-Model Fusion Block (CFB), and the Multi-Head Temporal Attention Block (MTB). The LTF module performs feature extraction and encodes temporal information for audio and video modalities separately, while the CFB module facilitates complementary learning between the modalities. The MTB module accounts for the temporal influence of all modalities on each unimodal branch. With the incorporation of the three well-designed modules, CMAFN can refine the inter-modality complementarity and intra-modality temporal dependencies, achieving the interaction between unimodal branches and adaptive balance between modalities. Evaluation results on widely used depression datasets, AVEC2013 and AVEC2014, demonstrate that the proposed CMAFN method outperforms state-of-the-art approaches for depression recognition tasks. The results highlight the potential of CMAFN as an effective tool for the early detection and diagnosis of depression.
更多查看译文
关键词
Depression,Automatic detection,Multi-modal fusion,Multimodal depression detection
AI 理解论文
溯源树
样例
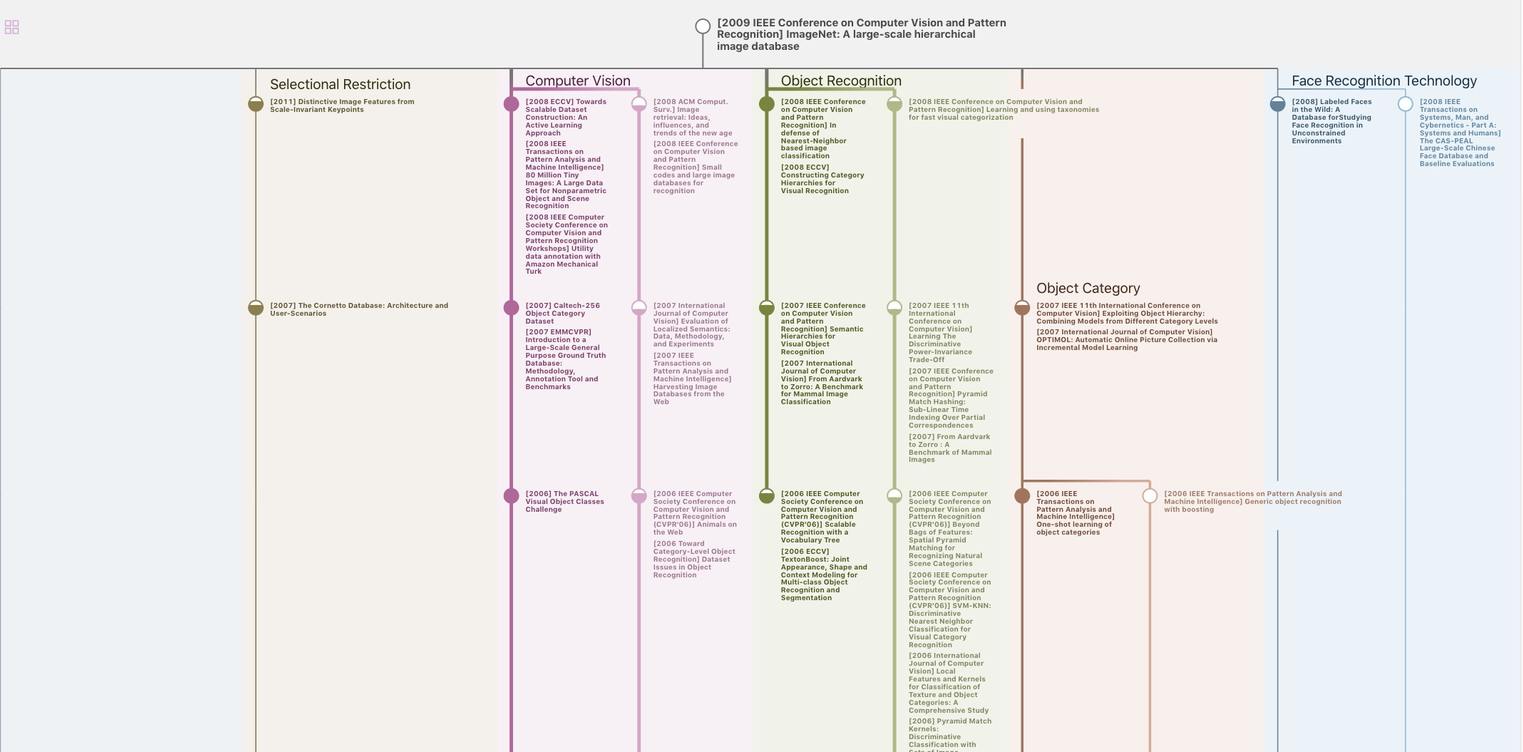
生成溯源树,研究论文发展脉络
Chat Paper
正在生成论文摘要