Diffusion models for out-of-distribution detection in digital pathology
MEDICAL IMAGE ANALYSIS(2024)
摘要
The ability to detect anomalies, i.e. anything not seen during training or out -of -distribution (OOD), in medical imaging applications is essential for successfully deploying machine learning systems. Filtering out OOD data using unsupervised learning is especially promising because it does not require costly annotations. A new class of models called AnoDDPMs, based on denoising diffusion probabilistic models (DDPMs), has recently achieved significant progress in unsupervised OOD detection. This work provides a benchmark for unsupervised OOD detection methods in digital pathology. By leveraging fast sampling techniques, we apply AnoDDPM on a large enough scale for whole -slide image analysis on the complete test set of the Camelyon16 challenge. Based on ROC analysis, we show that AnoDDPMs can detect OOD data with an AUC of up to 94.13 and 86.93 on two patch -level OOD detection tasks, outperforming the other unsupervised methods. We observe that AnoDDPMs alter the semantic properties of inputs, replacing anomalous data with more benign -looking tissue. Furthermore, we highlight the flexibility of AnoDDPM towards different information bottlenecks by evaluating reconstruction errors for inputs with different signal-to-noise ratios. While there is still a significant performance gap with fully supervised learning, AnoDDPMs show considerable promise in the field of OOD detection in digital pathology.
更多查看译文
关键词
Denoising diffusion probabilistic models,Out-of-distribution detection,Deep learning,Histopathology,Unsupervised learning
AI 理解论文
溯源树
样例
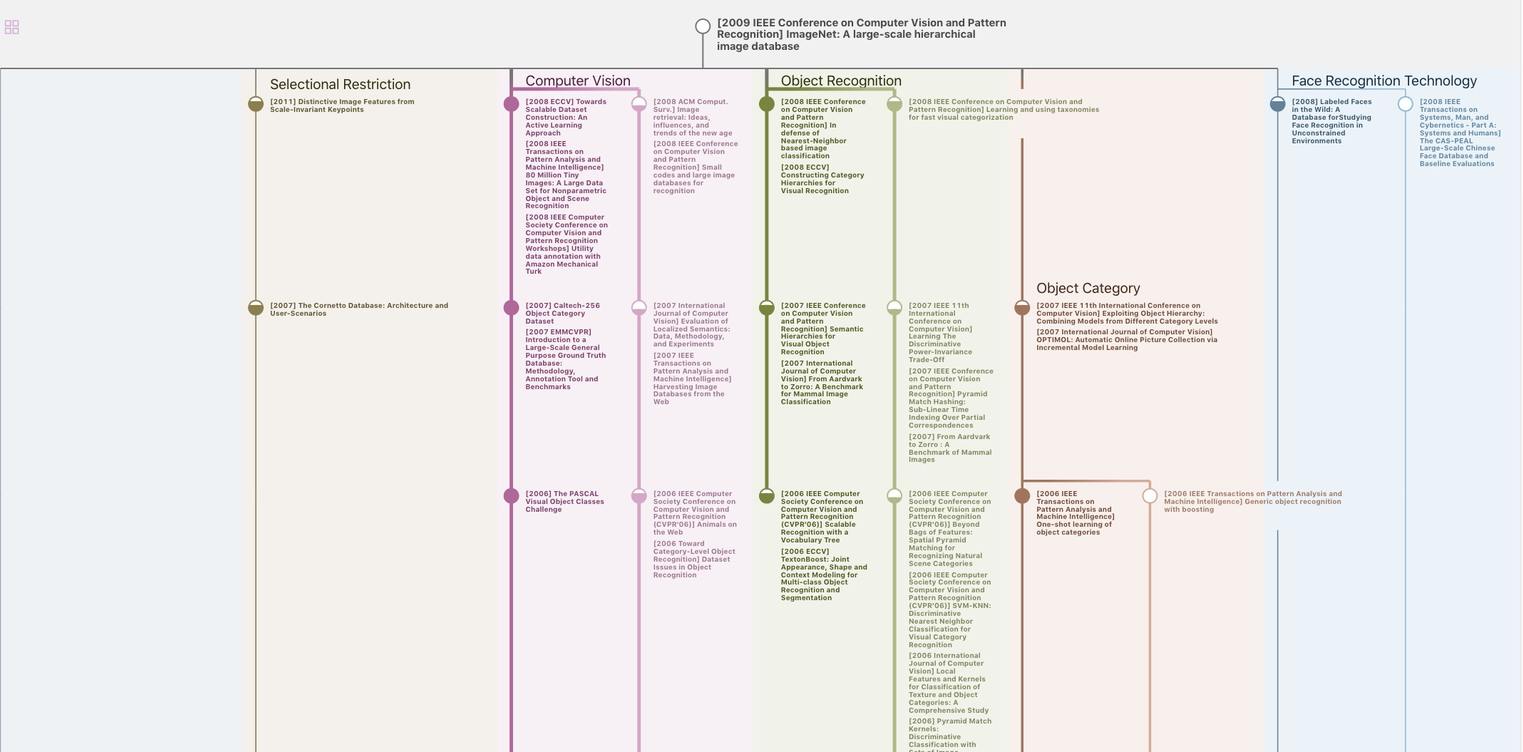
生成溯源树,研究论文发展脉络
Chat Paper
正在生成论文摘要