Learn What You Need in Personalized Federated Learning
CoRR(2024)
摘要
Personalized federated learning aims to address data heterogeneity across
local clients in federated learning. However, current methods blindly
incorporate either full model parameters or predefined partial parameters in
personalized federated learning. They fail to customize the collaboration
manner according to each local client's data characteristics, causing
unpleasant aggregation results. To address this essential issue, we propose
Learn2pFed, a novel algorithm-unrolling-based personalized federated
learning framework, enabling each client to adaptively select which part of its
local model parameters should participate in collaborative training. The key
novelty of the proposed Learn2pFed is to optimize each local model
parameter's degree of participant in collaboration as learnable parameters via
algorithm unrolling methods. This approach brings two benefits: 1)
mathmatically determining the participation degree of local model parameters in
the federated collaboration, and 2) obtaining more stable and improved
solutions. Extensive experiments on various tasks, including regression,
forecasting, and image classification, demonstrate that Learn2pFed
significantly outperforms previous personalized federated learning methods.
更多查看译文
AI 理解论文
溯源树
样例
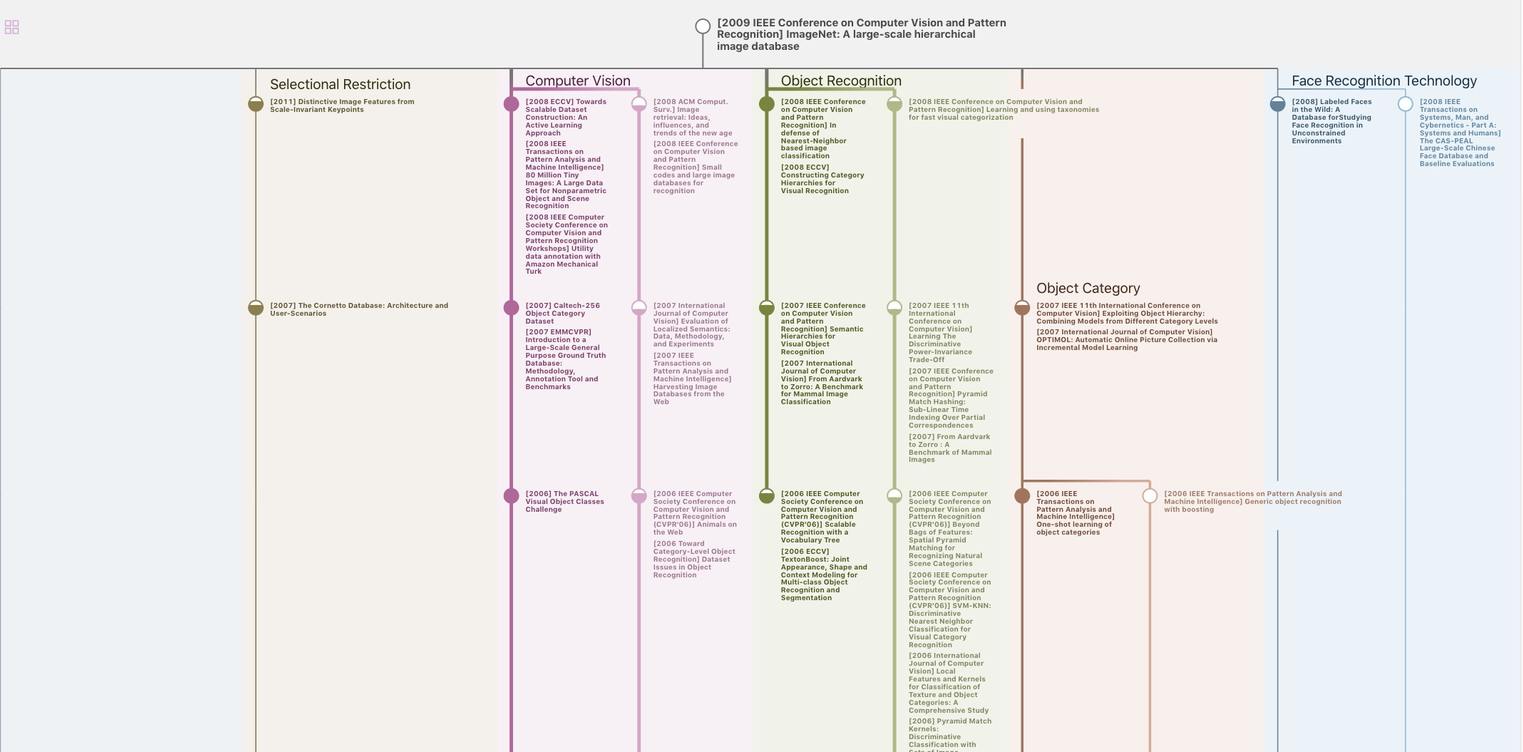
生成溯源树,研究论文发展脉络
Chat Paper
正在生成论文摘要