An Effective Multi-Scale Framework for Driver Behavior Recognition With Incomplete Skeletons
IEEE TRANSACTIONS ON VEHICULAR TECHNOLOGY(2024)
摘要
One essential issue in skeleton-based driver action recognition is that incomplete skeletons collected from real scenes would degrade model performance. However, existing models often ignore the missing joint preprocessing and tend to be over-parameterized. In this work, we propose 1) a padding strategy SmoothNode and 2) a skeleton-based Multi-Scale Excitation Graph Convolution Network (MSE-GCN). Firstly, SmoothNode, as a part of preprocessing, fills both missing frames and nodes in a smooth style and repairs the incomplete skeletons to a relatively complete state. Secondly, inspired by the efficient modeling ability of EfficientGCN in dynamic skeletons, the MSE-GCN model is designed to reason multi-scale spatial-temporal features through two improvements, i.e., Spatial Graph Convolution layer based on the Independent Self-connecting formulation mode (SGC-IS) and Multi-Scale Wrapper Fused Spatial-Temporal Excitation layer (MSW-FSTE). SGC-IS optimizes the normalized adjacency matrix formulation mode and strengthens connections between each pair of nodes, while MSW-FSTE excites temporal patterns with the global spatiotemporal features in a hierarchical residual-like style and learns multi-scale features in the temporal domain. By coupling these proposals, we develop SMOMS, a driver behavior recognition framework. Extensive experiments on three released datasets, i.e., Drive&Act, 3MDAD, and EBDD, demonstrate that the proposed SMOMS framework outperforms other methods.
更多查看译文
关键词
Driver behavior recognition,graph convolution network,incomplete skeletons,multi-scale features
AI 理解论文
溯源树
样例
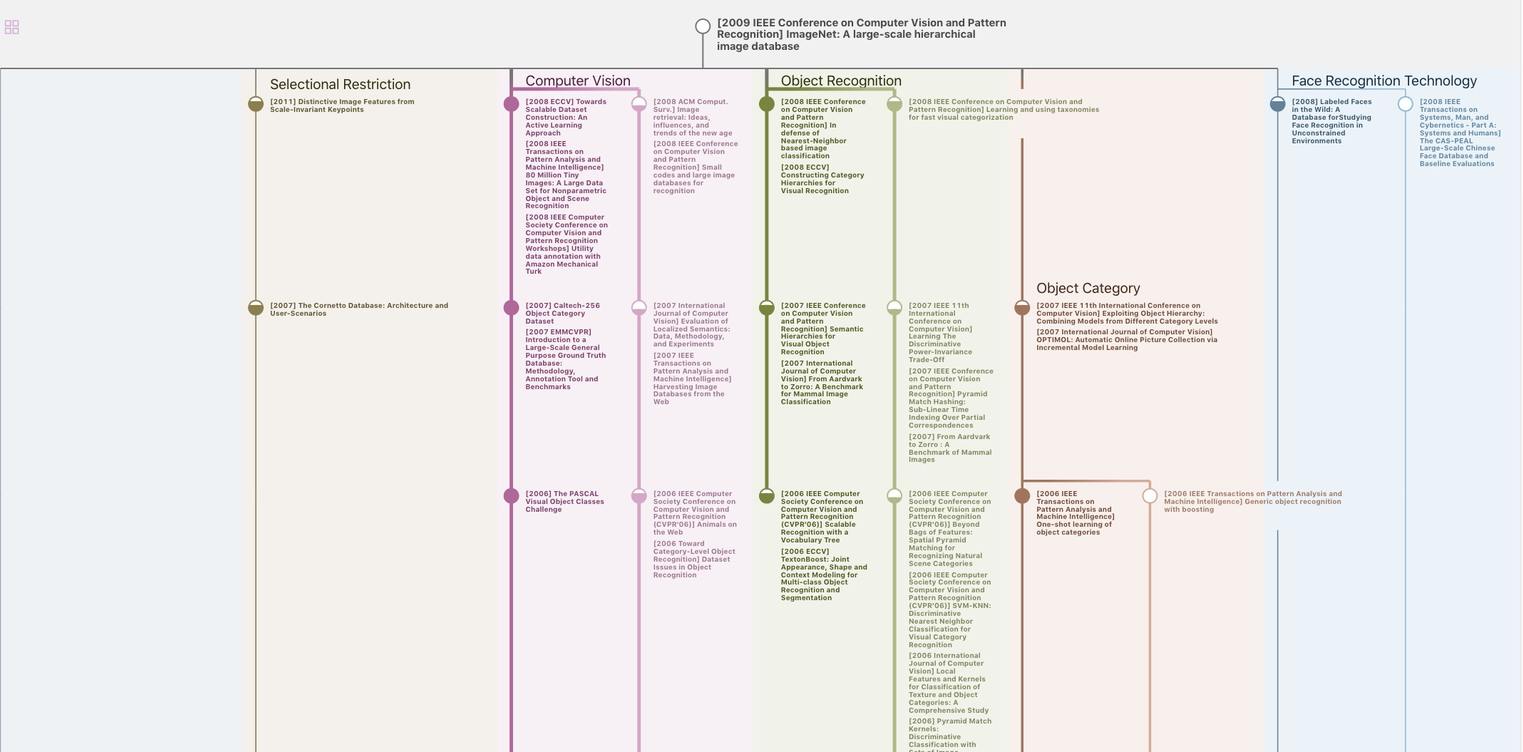
生成溯源树,研究论文发展脉络
Chat Paper
正在生成论文摘要