BENO: Boundary-embedded Neural Operators for Elliptic PDEs
ICLR(2024)
摘要
Elliptic partial differential equations (PDEs) are a major class of time-independent PDEs that play a key role in many scientific and engineering domains such as fluid dynamics, plasma physics, and solid mechanics. Recently, neural operators have emerged as a promising technique to solve elliptic PDEs more efficiently by directly mapping the input to solutions. However, existing networks typically neglect complex geometries and inhomogeneous boundary values present in the real world. Here we introduce Boundary-Embedded Neural Operators (BENO), a novel neural operator architecture that embeds the complex geometries and inhomogeneous boundary values into the solving of elliptic PDEs. Inspired by classical Green's function, BENO consists of two Graph Neural Networks (GNNs) for interior source term and boundary values, respectively. Furthermore, a Transformer encoder maps the global boundary geometry into a latent vector which influences each message passing layer of the GNNs. We test our model and strong baselines extensively in elliptic PDEs with complex boundary conditions. We show that all existing baseline methods fail to learn the solution operator. In contrast, our model, endowed with boundary-embedded architecture, outperforms state-of-the-art neural operators and strong baselines by an average of 60.96%.
更多查看译文
关键词
AI for PDEs,physical simulation,neural operators,boundary-embedded
AI 理解论文
溯源树
样例
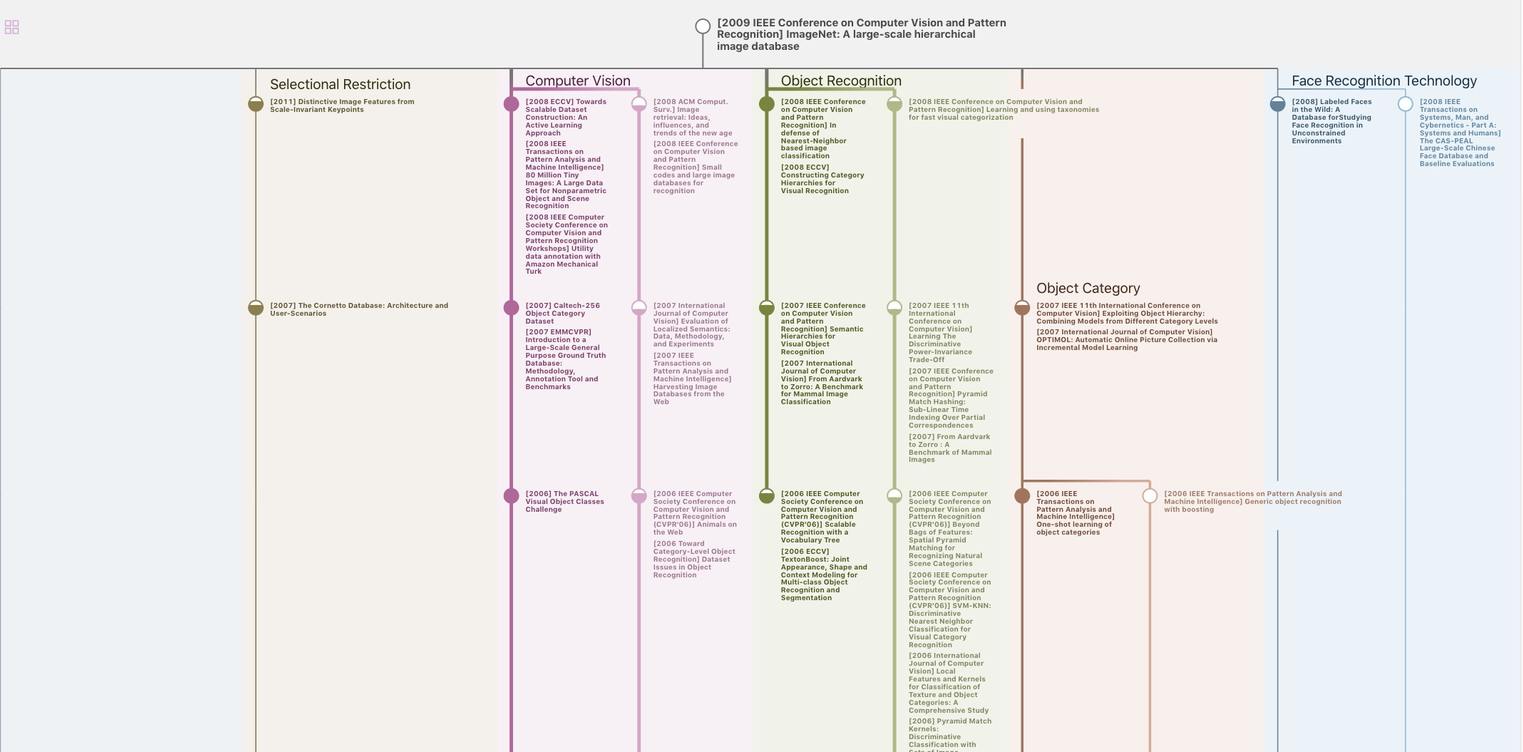
生成溯源树,研究论文发展脉络
Chat Paper
正在生成论文摘要