Divide and not forget: Ensemble of selectively trained experts in Continual Learning
CoRR(2024)
摘要
Class-incremental learning is becoming more popular as it helps models widen
their applicability while not forgetting what they already know. A trend in
this area is to use a mixture-of-expert technique, where different models work
together to solve the task. However, the experts are usually trained all at
once using whole task data, which makes them all prone to forgetting and
increasing computational burden. To address this limitation, we introduce a
novel approach named SEED. SEED selects only one, the most optimal expert for a
considered task, and uses data from this task to fine-tune only this expert.
For this purpose, each expert represents each class with a Gaussian
distribution, and the optimal expert is selected based on the similarity of
those distributions. Consequently, SEED increases diversity and heterogeneity
within the experts while maintaining the high stability of this ensemble
method. The extensive experiments demonstrate that SEED achieves
state-of-the-art performance in exemplar-free settings across various
scenarios, showing the potential of expert diversification through data in
continual learning.
更多查看译文
关键词
continual learning,class incremental learning
AI 理解论文
溯源树
样例
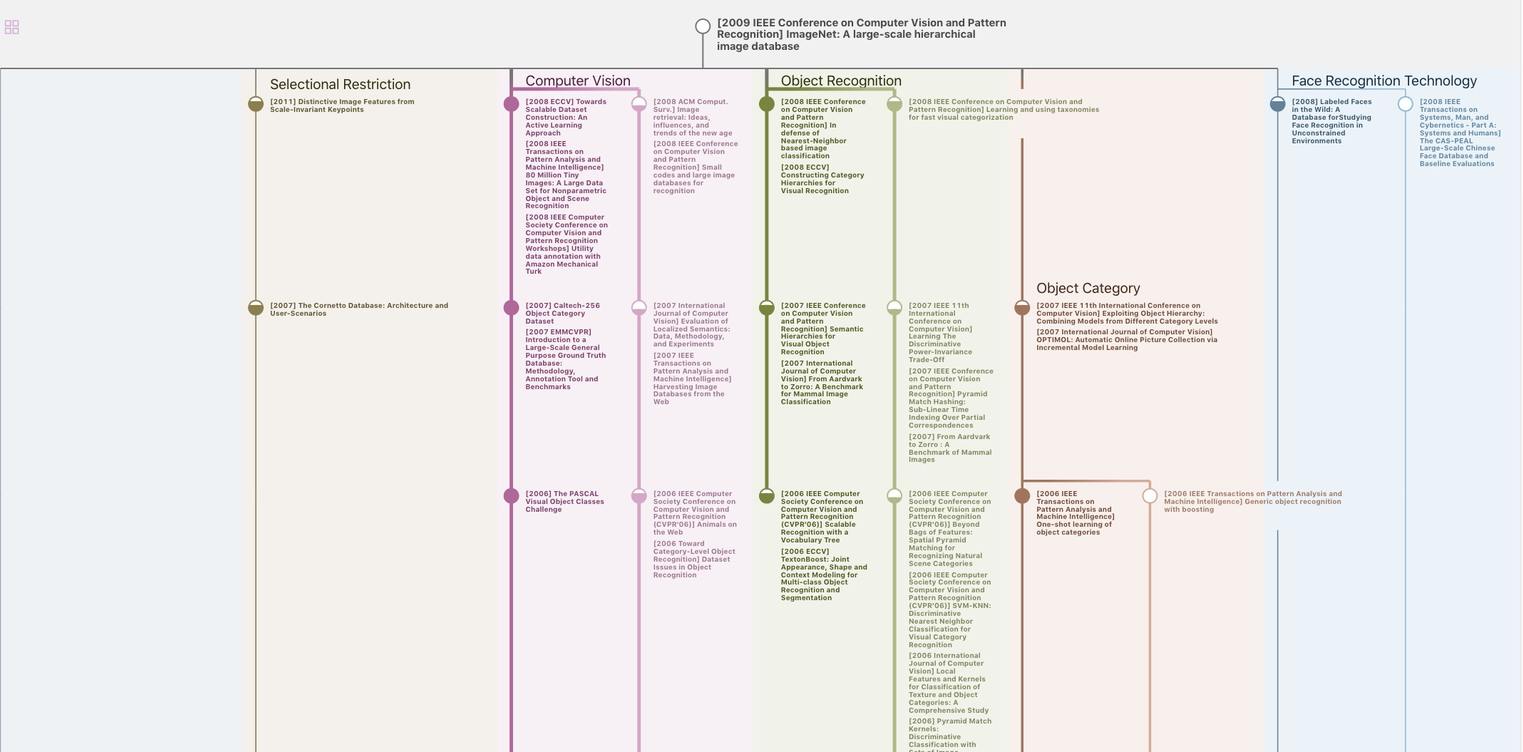
生成溯源树,研究论文发展脉络
Chat Paper
正在生成论文摘要