Validating Siamese embedded neural networks with identical representations for efficient model convergence
KNOWLEDGE-BASED SYSTEMS(2024)
摘要
Deep learning, neural architecture search, reinforcement learning, and embedded learning use scaled data, hyperspace, reward, and similarity to produce efficient converging neural networks. However, all these state-ofthe-art frameworks require training to evaluate whether the examples are identical. Therefore, we propose our Probabilistic Asymmetric Convergence Network with Validation and Transform -Learning (PACNVT) framework that learns with fewer data, reduces the hyperspace with our validation technique and modified tangent activation function, reinforces the learning with our transform learning algorithms, and ascertains similarity independent of spatial and mask for transformational consistency. Furthermore, our framework generates neural networks that yield state-of-the-art accuracies on MNIST, OMNIGLOT, and CIFAR datasets. Moreover, representing regression as a similarity problem unveils previously unseen residual fluid intelligence patterns, yielding 99.92% accuracy with as little as 20 pairs.
更多查看译文
关键词
Zero-shot,One-shot,Few-shot,Validation,Similarity
AI 理解论文
溯源树
样例
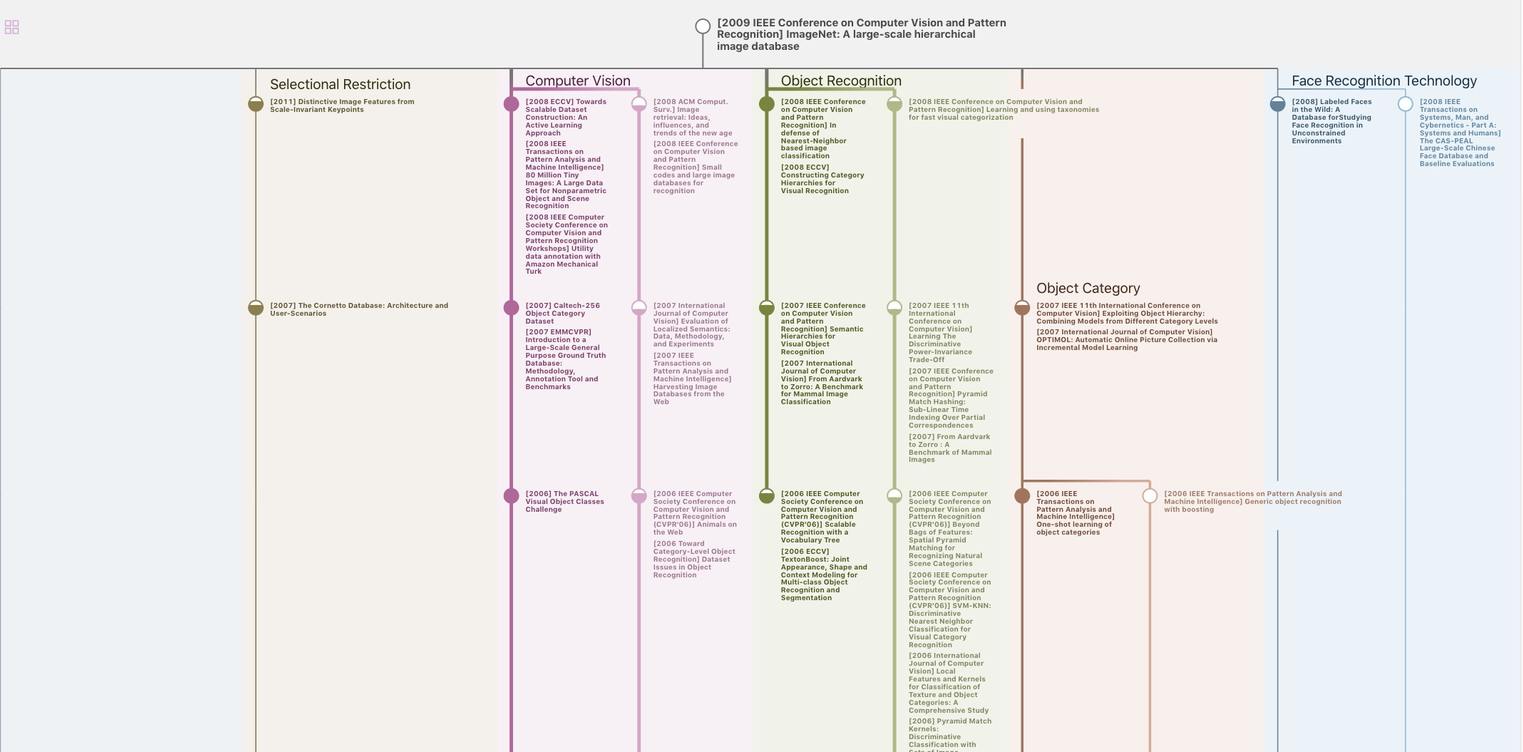
生成溯源树,研究论文发展脉络
Chat Paper
正在生成论文摘要