Differentially-Private Hierarchical Federated Learning
arxiv(2024)
摘要
While federated learning (FL) eliminates the transmission of raw data over a
network, it is still vulnerable to privacy breaches from the communicated model
parameters. In this work, we propose Hierarchical
Federated Learning with Hierarchical
Differential Privacy (H^2FDP), a DP-enhanced FL
methodology for jointly optimizing privacy and performance in hierarchical
networks. Building upon recent proposals for Hierarchical Differential Privacy
(HDP), one of the key concepts of H^2FDP is adapting DP noise injection
at different layers of an established FL hierarchy – edge devices, edge
servers, and cloud servers – according to the trust models within particular
subnetworks. We conduct a comprehensive analysis of the convergence behavior of
H^2FDP, revealing conditions on parameter tuning under which the
training process converges sublinearly to a finite stationarity gap that
depends on the network hierarchy, trust model, and target privacy level.
Leveraging these relationships, we develop an adaptive control algorithm for
H^2FDP that tunes properties of local model training to minimize
communication energy, latency, and the stationarity gap while striving to
maintain a sub-linear convergence rate and meet desired privacy criteria.
Subsequent numerical evaluations demonstrate that H^2FDP obtains
substantial improvements in these metrics over baselines for different privacy
budgets, and validate the impact of different system configurations.
更多查看译文
AI 理解论文
溯源树
样例
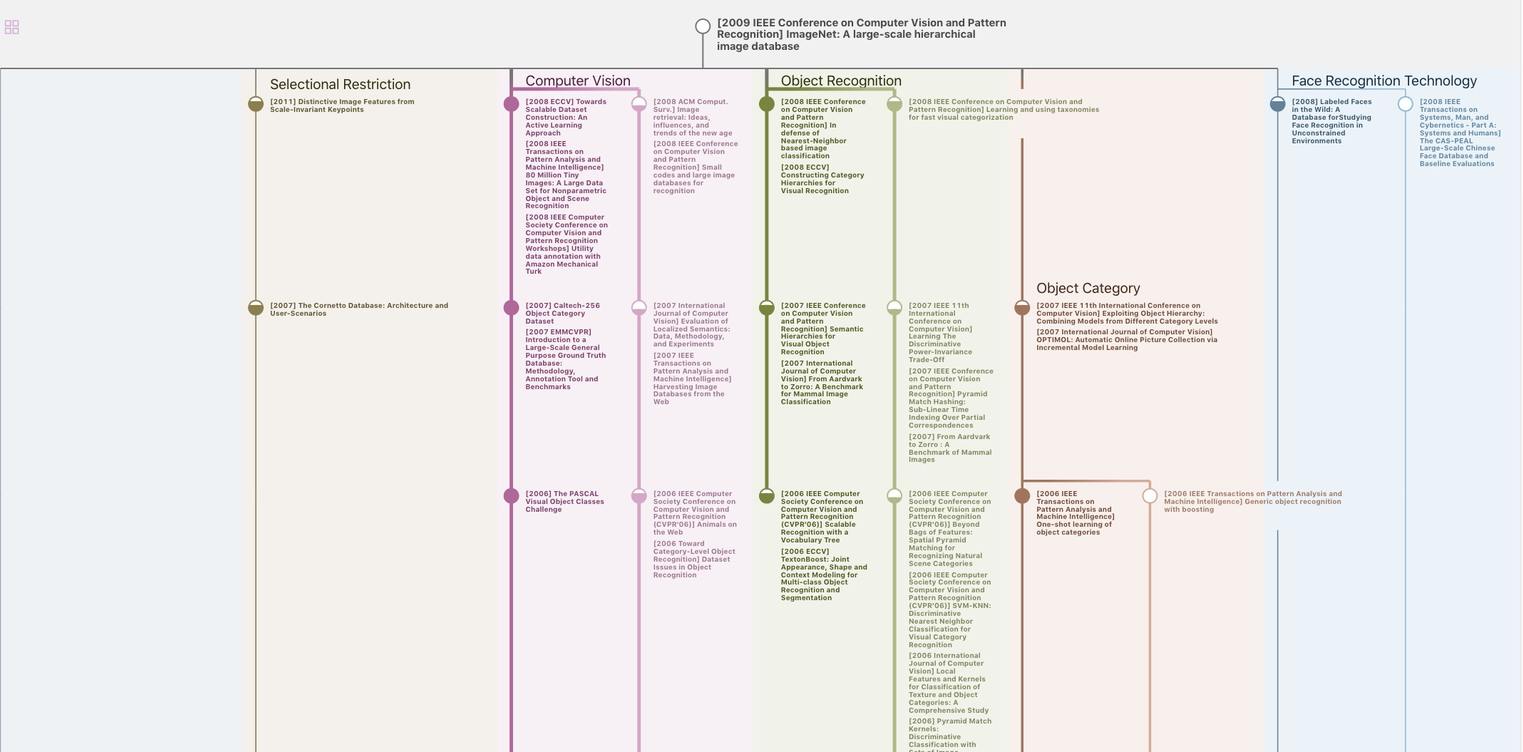
生成溯源树,研究论文发展脉络
Chat Paper
正在生成论文摘要