Reinforced Knowledge Distillation for Time Series Regression
IEEE Transactions on Artificial Intelligence(2023)
摘要
As one of the most popular and effective methods in model compression, knowledge distillation (KD) attempts to transfer knowledge from single or multiple large-scale networks (i.e.,
Teachers
) to a compact network (i.e.,
Student
). For the multi-teacher scenario, existing methods either assign equal or fixed weights for different teacher models during distillation, which can be inefficient as teachers might perform variously or even oppositely on different training samples. To address this issue, we propose a novel reinforced knowledge distillation method with negatively correlated teachers which are generated via negative correlation learning. The negatively correlated teachers would encourage teachers to learn different aspects of data and thus the ensemble of them can be more comprehensive and suitable for multi-teacher KD. Subsequently, a reinforced KD algorithm is proposed to dynamically employ proper teachers for different training instances via dueling Double Deep Q-Network (DDQN). Our proposed method complements the existing KD procedure on teacher generation and selection. Extensive experimental results on two real-world time series regression tasks clearly demonstrate that the proposed approach could achieve superior performance over state-of-the-art methods. The PyTorch implementation of our proposed approach is available at
https://github.com/xuqing88/RL-KD-for-time-series-regression
.
更多查看译文
关键词
Reinforcement learning,knowledge distillation,time series regression
AI 理解论文
溯源树
样例
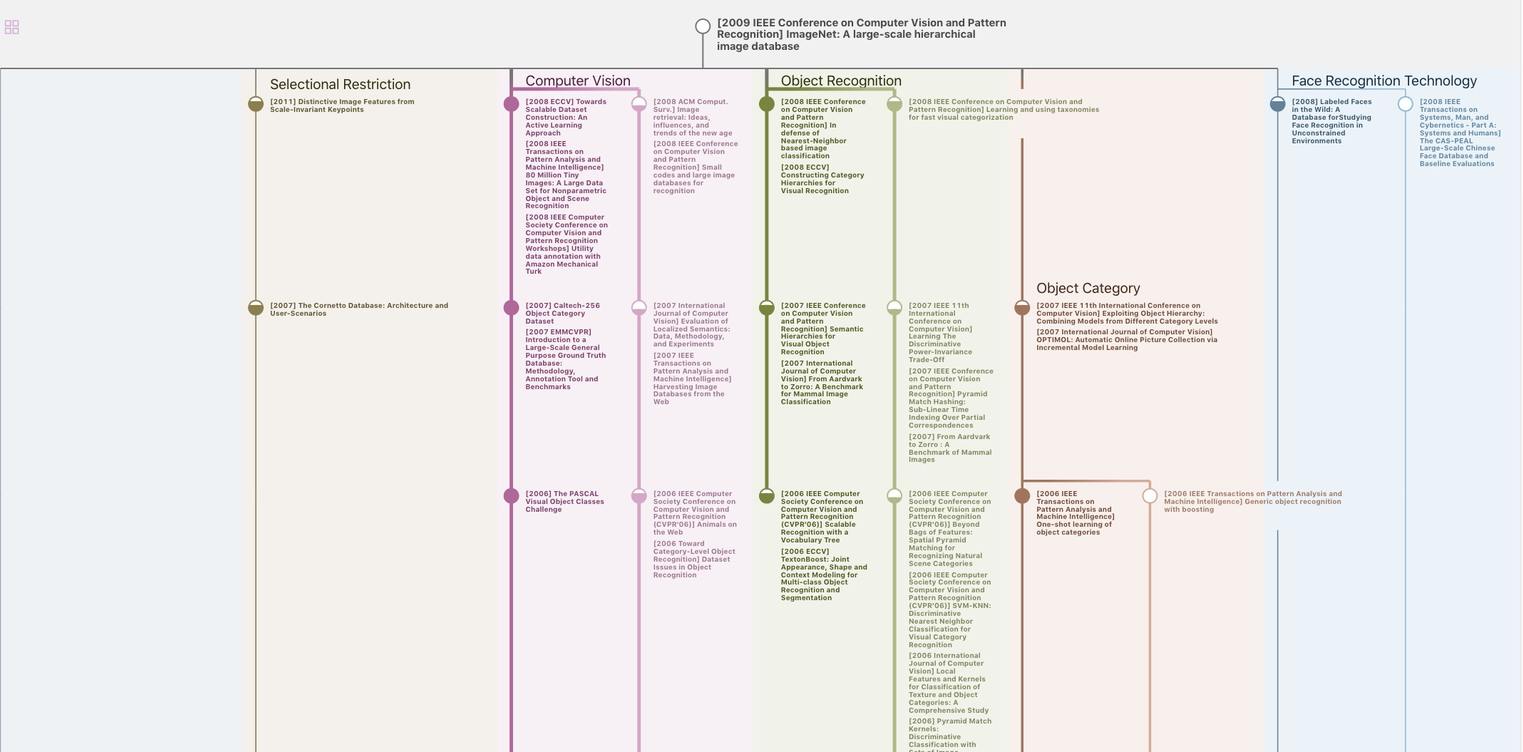
生成溯源树,研究论文发展脉络
Chat Paper
正在生成论文摘要