Inter-Class and Inter-Domain Semantic Augmentation for Domain Generalization
IEEE TRANSACTIONS ON IMAGE PROCESSING(2024)
摘要
The domain generalization approach seeks to develop a universal model that performs well on unknown target domains with the aid of diverse source domains. Data augmentation has proven to be an effective method to enhance domain generalization in computer vision. Recently, semantic-level based data augmentation has yielded remarkable results. However, these methods focus on sampling semantic directions on feature space from intra-class and intra-domain, limiting the diversity of the source domain. To address this issue, we propose a novel approach called Inter-Class and Inter-Domain Semantic Augmentation (CDSA) for domain generalization. We first introduce a sampling-based method called CrossSmooth to obtain semantic directions from inter-class. Then, CrossVariance obtains the styles of different domains by sampling semantic directions. Our experiments on four well-known domain generalization benchmark datasets (Digits-DG, PACS, Office-Home, and DomainNet) demonstrate the effectiveness of our approach. We also validate our approach on commonly-used semantic segmentation datasets, namely GTAV, SYNTHIA, Cityscapes, Mapillary, and BDDS which also show significant improvements.
更多查看译文
关键词
Semantics,Data augmentation,Training,Self-supervised learning,Painting,Data models,Picture archiving and communication systems,Domain generalization,data augmentation,inter-class,inter-domain
AI 理解论文
溯源树
样例
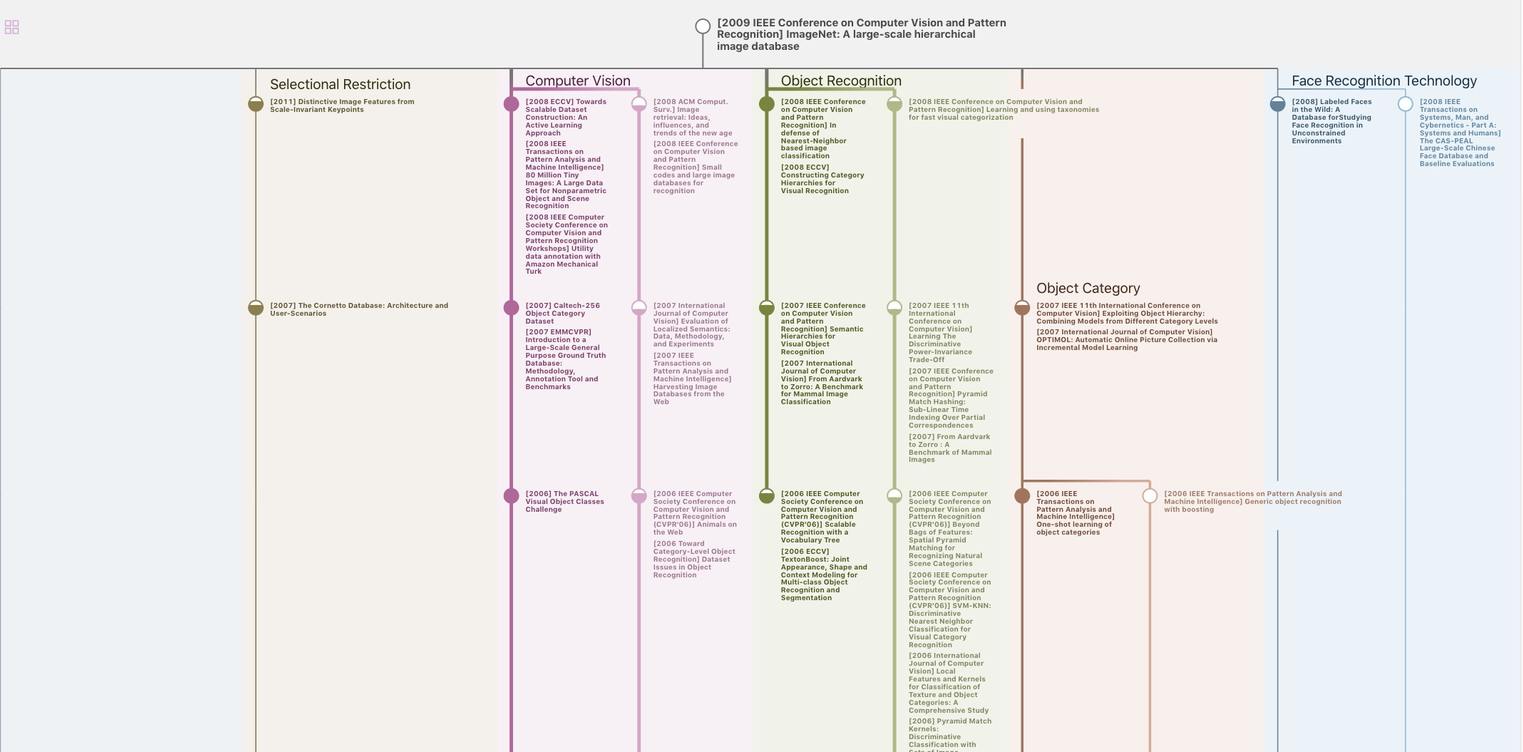
生成溯源树,研究论文发展脉络
Chat Paper
正在生成论文摘要