Lightweight Attention-Guided YOLO With Level Set Layer for Landslide Detection From Optical Satellite Images
IEEE JOURNAL OF SELECTED TOPICS IN APPLIED EARTH OBSERVATIONS AND REMOTE SENSING(2024)
摘要
Landslide inventory is significant for landslide disaster reduction. To construct the landslide inventory, deep learning has received growing attention to detect landslides from satellite images. Among various deep learning algorithms, you-only-look-once (YOLO) has a strong ability to detect objects efficiently and has been widely used in landslide extraction. Despite its efficiency, there is no general rule to select the backbone and attention mechanism for YOLO. The selection of these two modules depends on specific application needs. Meanwhile, YOLO output is a series of anchor boxes, not accurate landslide boundaries. A single bounding box may contain many landslides and cannot extract individual landslides, limiting the YOLO applications in constructing landslide inventory. To address these issues, this article presents a lightweight attention-guided YOLO with level set layer (LA-YOLO-LLL) for landslide detection from optical satellite images. First, we introduced the MobileNetv3 to replace the original backbone of YOLO to simultaneously reduce the parameter complexity and improve the model transferability. Then, we presented a light pyramid features reuse fusion attention mechanism to improve landslide detection performance. Finally, we integrated the level set layer into YOLO head to produce accurate landslide boundaries. This article validated the accuracy and transferability of the presented method in two study areas (Bijie and Taiwan) with similar geo-environmental conditions. Experimental results show that the presented LA-YOLO-LLL model outperformed traditional YOLO in landslide detection. Findings in this article are valuable for landslide inventory construction, land use planning and risk control.
更多查看译文
关键词
Attention mechanism,landslide boundary,landslide inventory,object detection,the level set layer,you-only-look-once (YOLO)
AI 理解论文
溯源树
样例
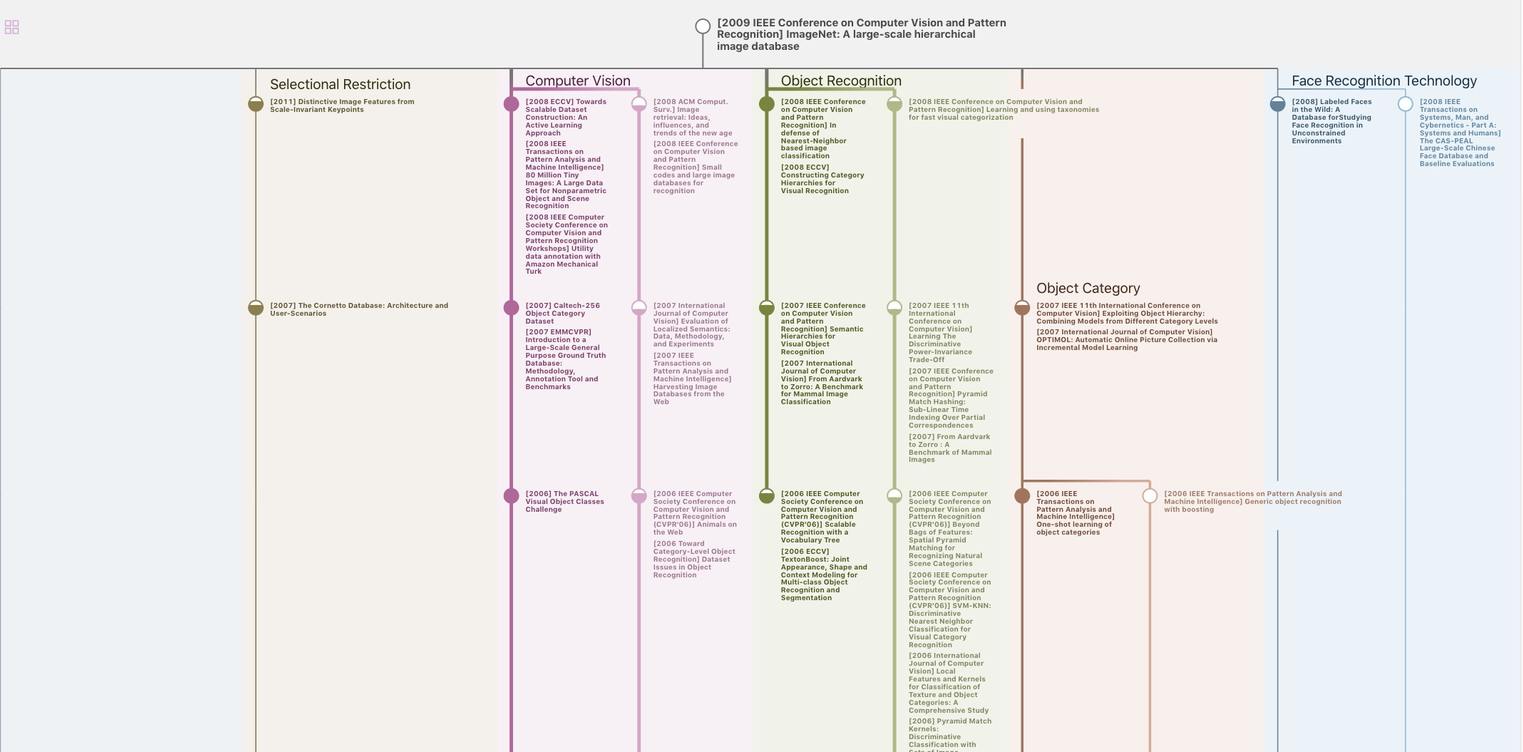
生成溯源树,研究论文发展脉络
Chat Paper
正在生成论文摘要