Toward training NLP models to take into account privacy leakages.
2023 IEEE International Conference on Big Data (BigData)(2023)
摘要
With the rise of machine learning and data-driven models especially in the field of Natural Language Processing (NLP), a strong demand for sharing data between organisations has emerged. However datasets are usually composed of personal data and thus subject to numerous regulations which require anonymization before disseminating the data. In the medical domain for instance, patient records are extremely sensitive and private, but the de-identification of medical documents is a complex task. Recent advances in NLP models have shown encouraging results in this field, but the question of whether deploying such models is safe remains.In this paper, we evaluate three privacy risks on NLP models trained on sensitive data. Specifically, we evaluate counterfactual memorization, which corresponds to rare and sensitive information which has too much influence on the model. We also evaluate membership inference as well as the ability to extract verbatim training data from the model. With this evaluation, we can cure data at risk from the training data and calibrate hyper parameters to provide a supplementary utility and privacy trade-off to the usual mitigation strategies such as using differential privacy. We exhaustively illustrate the privacy leakage of NLP models through a use-case using medical texts and discuss the impact of both the proposed methodology and mitigation schemes.
更多查看译文
关键词
NLP models,Privacy,Membership Inference,Counterfactual Memorisation,Data Extraction
AI 理解论文
溯源树
样例
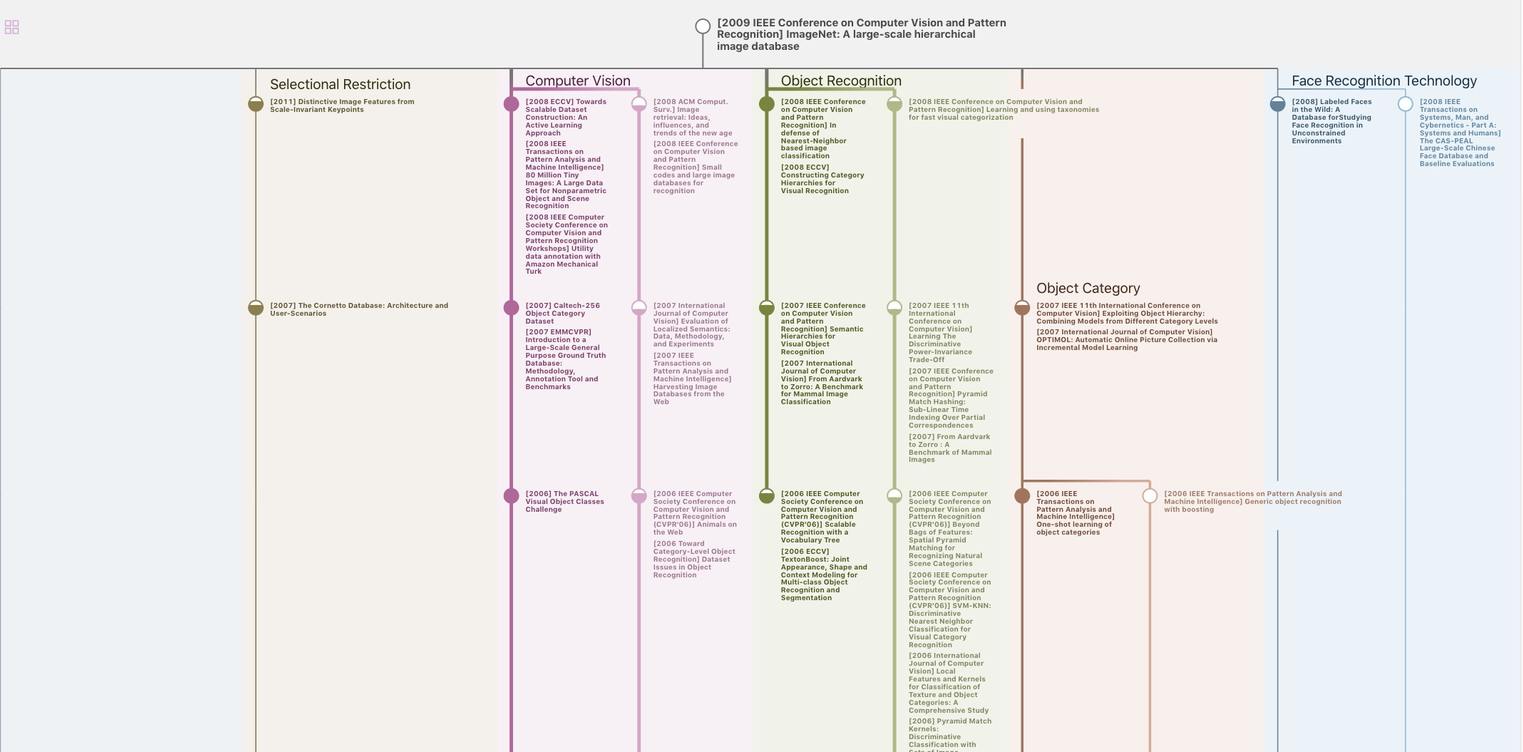
生成溯源树,研究论文发展脉络
Chat Paper
正在生成论文摘要