RoMAT: Role-based multi-agent transformer for generalizable heterogeneous cooperation
Neural Networks(2024)
摘要
Multi-task multi-agent systems (MASs) are challenging to model because they involve heterogeneous agents with different behavior patterns that need to cooperate across various tasks. Existing networks for single-agent policies are not suitable for this setting, as they cannot share policies among agents without losing task-specific performance. We propose a novel framework called Role-based Multi-Agent Transformer (RoMAT), which uses a sequence modeling technique and a role-based actor to enable agents to adapt to different tasks and roles in MASs. RoMAT has a modular model architecture, where backbone networks are shared by all agents, but a small part of the parameters (role-based actor) is independent, depending on the agents’ exclusive structures. We evaluate RoMAT on several benchmark tasks and show that it can capture the behavior patterns of heterogeneous agents and achieve better performance and generalization than other methods in both single and multi-task settings.
更多查看译文
关键词
Multi-agent system,Multi-task generalization,Imitation learning
AI 理解论文
溯源树
样例
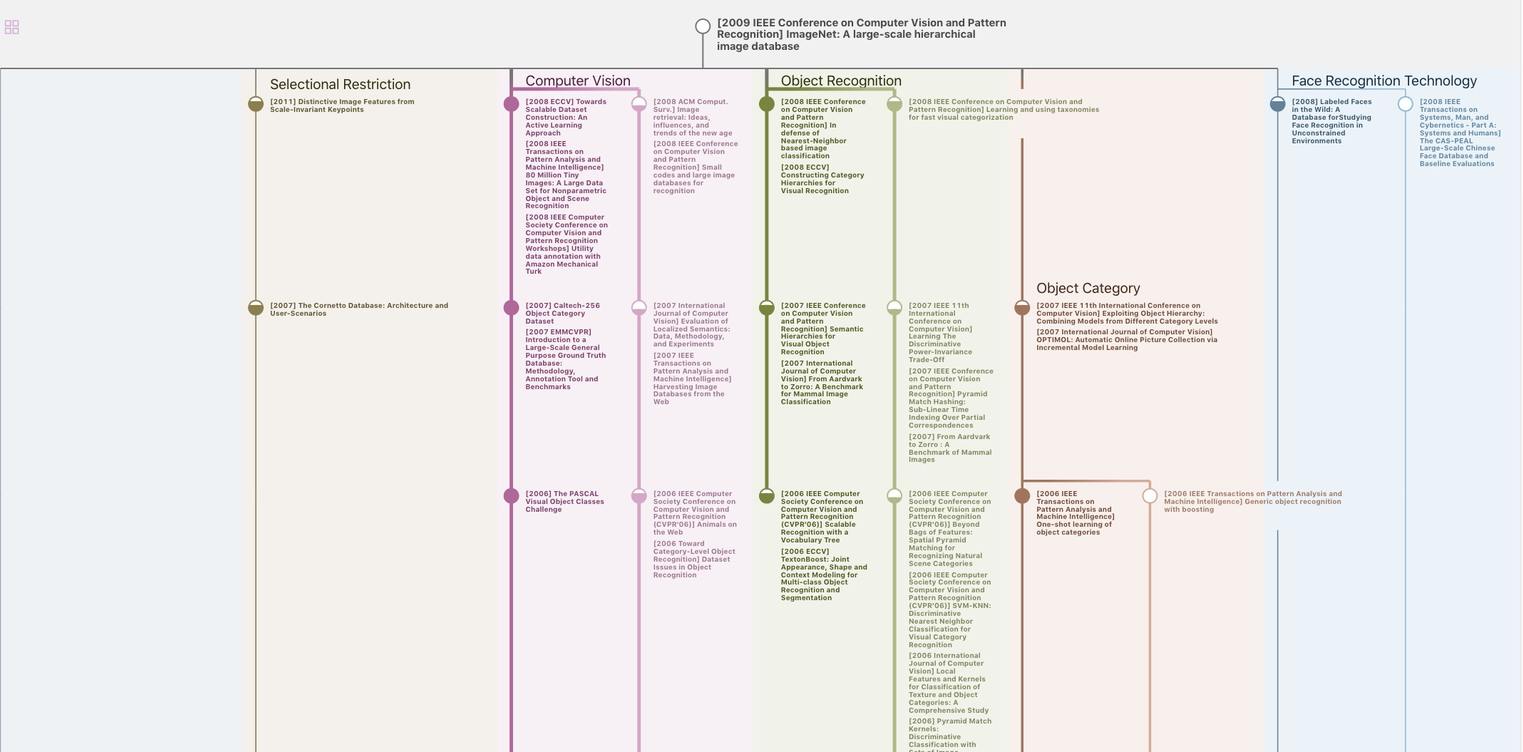
生成溯源树,研究论文发展脉络
Chat Paper
正在生成论文摘要