Decision Theoretic Foundations for Experiments Evaluating Human Decisions
arXiv (Cornell University)(2024)
摘要
DeHow well people use information displays to make decisions is of primary interest in human-centered AI, model explainability, data visualization, and related areas. However, what constitutes a decision problem, and what is required for a study to establish that human decisions could be improved remain open to speculation. We propose a widely applicable definition of a decision problem synthesized from statistical decision theory and information economics as a standard for establishing when human decisions can be improved in HCI. We argue that to attribute loss in human performance to forms of bias, an experiment must provide participants with the information that a rational agent would need to identify the utility-maximizing decision. As a demonstration, we evaluate the extent to which recent evaluations of decision-making from the literature on AI-assisted decisions achieve these criteria. We find that only 10 (26%) of 39 studies that claim to identify biased behavior present participants with sufficient information to characterize their behavior as deviating from good decision-making in at least one treatment condition. We motivate the value of studying well-defined decision problems by describing a characterization of performance losses they allow us to conceive. In contrast, the ambiguities of a poorly communicated decision problem preclude normative interpretation. We conclude with recommendations for practice.
更多查看译文
关键词
Prediction Accuracy
AI 理解论文
溯源树
样例
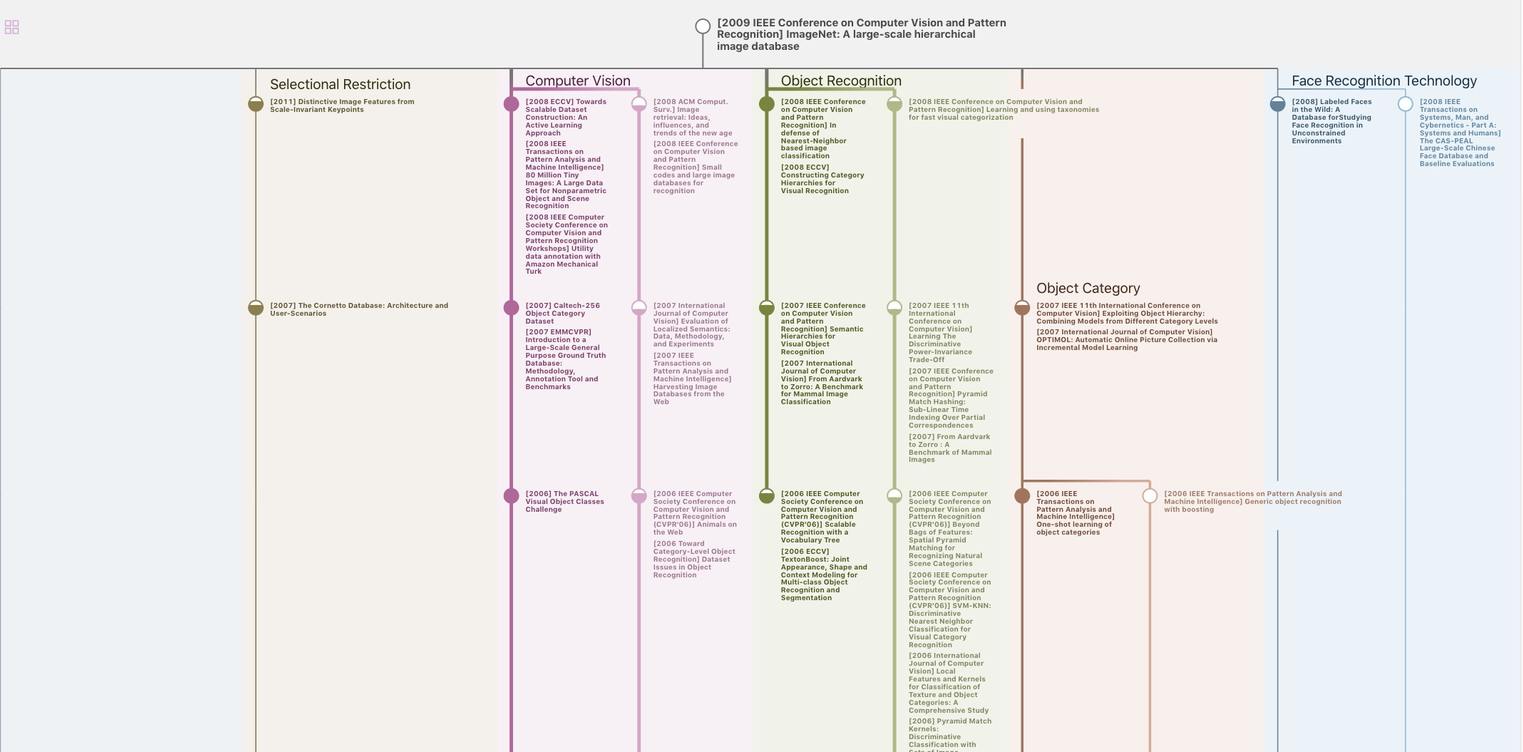
生成溯源树,研究论文发展脉络
Chat Paper
正在生成论文摘要