A large dataset curation and benchmark for drug target interaction
CoRR(2024)
摘要
Bioactivity data plays a key role in drug discovery and repurposing. The
resource-demanding nature of in vitro and in vivo
experiments, as well as the recent advances in data-driven computational
biochemistry research, highlight the importance of in silico drug
target interaction (DTI) prediction approaches. While numerous large public
bioactivity data sources exist, research in the field could benefit from better
standardization of existing data resources. At present, different research
works that share similar goals are often difficult to compare properly because
of different choices of data sources and train/validation/test split
strategies. Additionally, many works are based on small data subsets, leading
to results and insights of possible limited validity. In this paper we propose
a way to standardize and represent efficiently a very large dataset curated
from multiple public sources, split the data into train, validation and test
sets based on different meaningful strategies, and provide a concrete
evaluation protocol to accomplish a benchmark. We analyze the proposed data
curation, prove its usefulness and validate the proposed benchmark through
experimental studies based on an existing neural network model.
更多查看译文
AI 理解论文
溯源树
样例
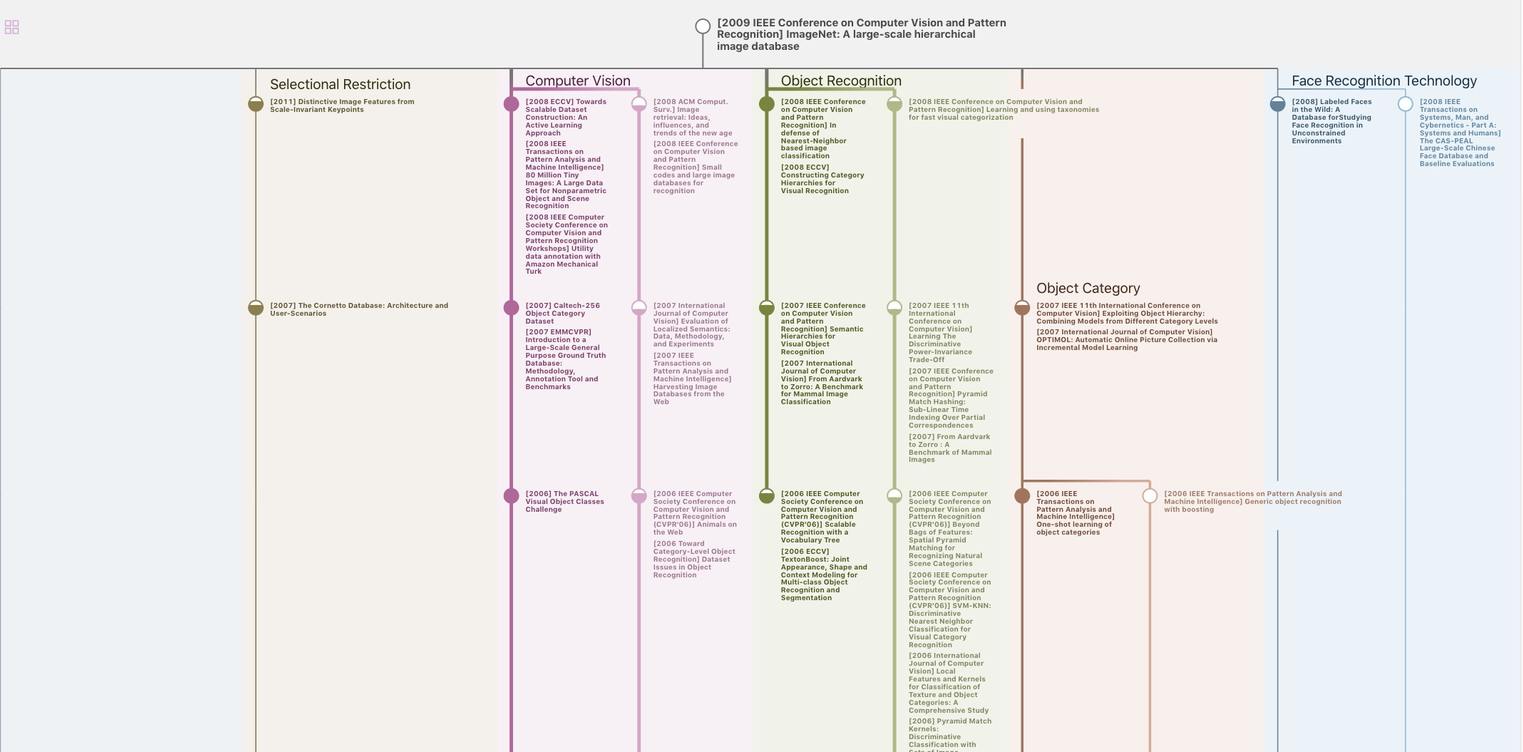
生成溯源树,研究论文发展脉络
Chat Paper
正在生成论文摘要