A Cross-View Hierarchical Graph Learning Hypernetwork for Skill Demand-Supply Joint Prediction
AAAI 2024(2024)
摘要
The rapidly changing landscape of technology and industries leads to dynamic skill requirements, making it crucial for employees and employers to anticipate such shifts to maintain a competitive edge in the labor market. Existing efforts in this area either relies on domain-expert knowledge or regarding the skill evolution as a simplified time series forecasting problem. However, both approaches overlook the sophisticated relationships among different skills and the inner-connection between skill demand and supply variations.
In this paper, we propose a Cross-view Hierarchical Graph learning Hypernetwork (CHGH) framework for joint skill demand-supply prediction. Specifically, CHGH is an encoder-decoder network consisting of i) a cross-view graph encoder to capture the interconnection between skill demand and supply, ii) a hierarchical graph encoder to model the co-evolution of skills from a cluster-wise perspective, and iii) a conditional hyper-decoder to jointly predict demand and supply variations by incorporating historical demand-supply gaps. Extensive experiments on three real-world datasets demonstrate the superiority of the proposed framework compared to seven baselines and the effectiveness of the three modules.
更多查看译文
关键词
PEAI: AI & Jobs/Labor,DMKM: Graph Mining, Social Network Analysis & Community
AI 理解论文
溯源树
样例
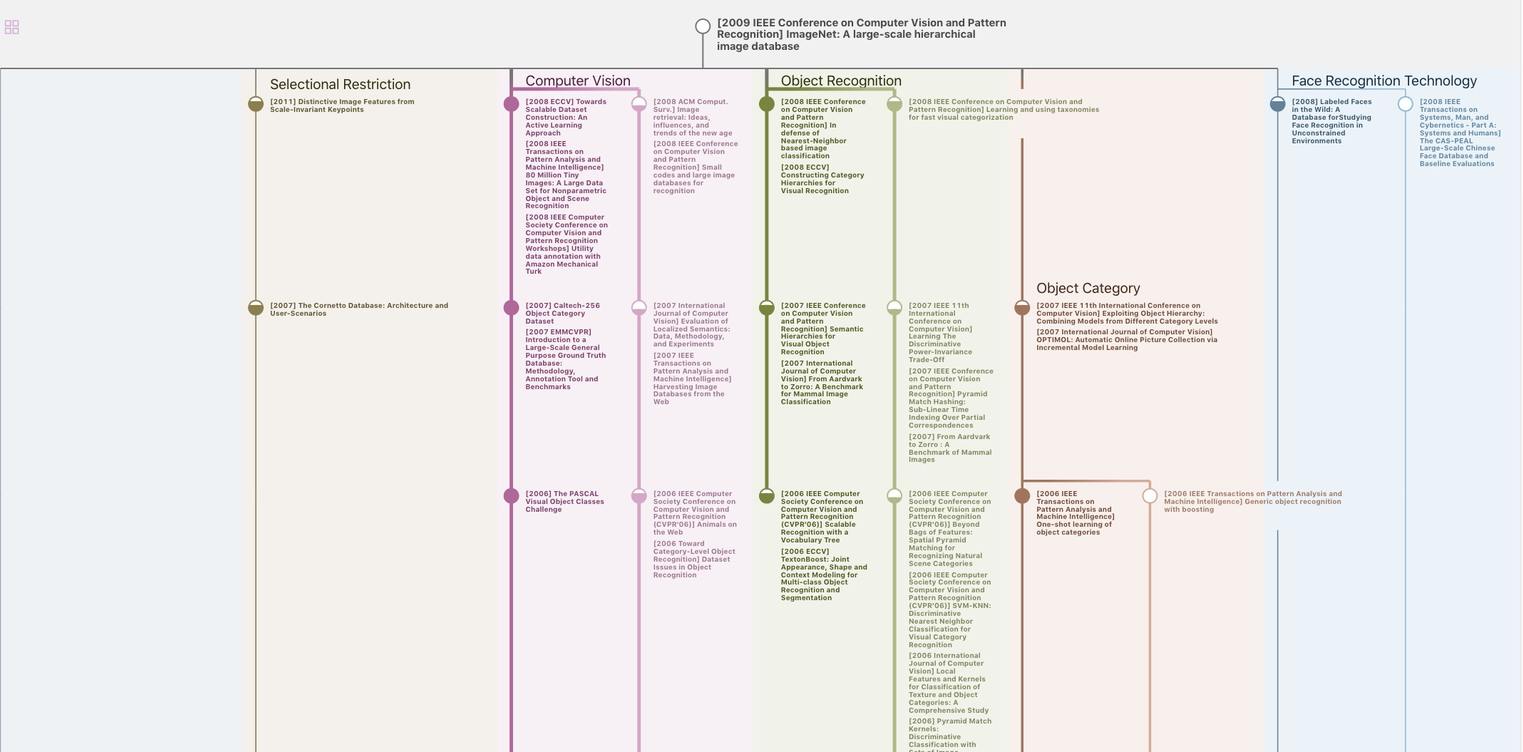
生成溯源树,研究论文发展脉络
Chat Paper
正在生成论文摘要