Mapping the Multiverse of Latent Representations
ICML 2024(2024)
摘要
Echoing recent calls to counter reliability and robustness concerns inmachine learning via multiverse analysis, we present PRESTO, a principledframework for mapping the multiverse of machine-learning models that rely onlatent representations. Although such models enjoy widespread adoption, thevariability in their embeddings remains poorly understood, resulting inunnecessary complexity and untrustworthy representations. Our framework usespersistent homology to characterize the latent spaces arising from differentcombinations of diverse machine-learning methods, (hyper)parameterconfigurations, and datasets, allowing us to measure their pairwise(dis)similarity and statistically reason about their distributions. As wedemonstrate both theoretically and empirically, our pipeline preservesdesirable properties of collections of latent representations, and it can beleveraged to perform sensitivity analysis, detect anomalous embeddings, orefficiently and effectively navigate hyperparameter search spaces.
更多查看译文
AI 理解论文
溯源树
样例
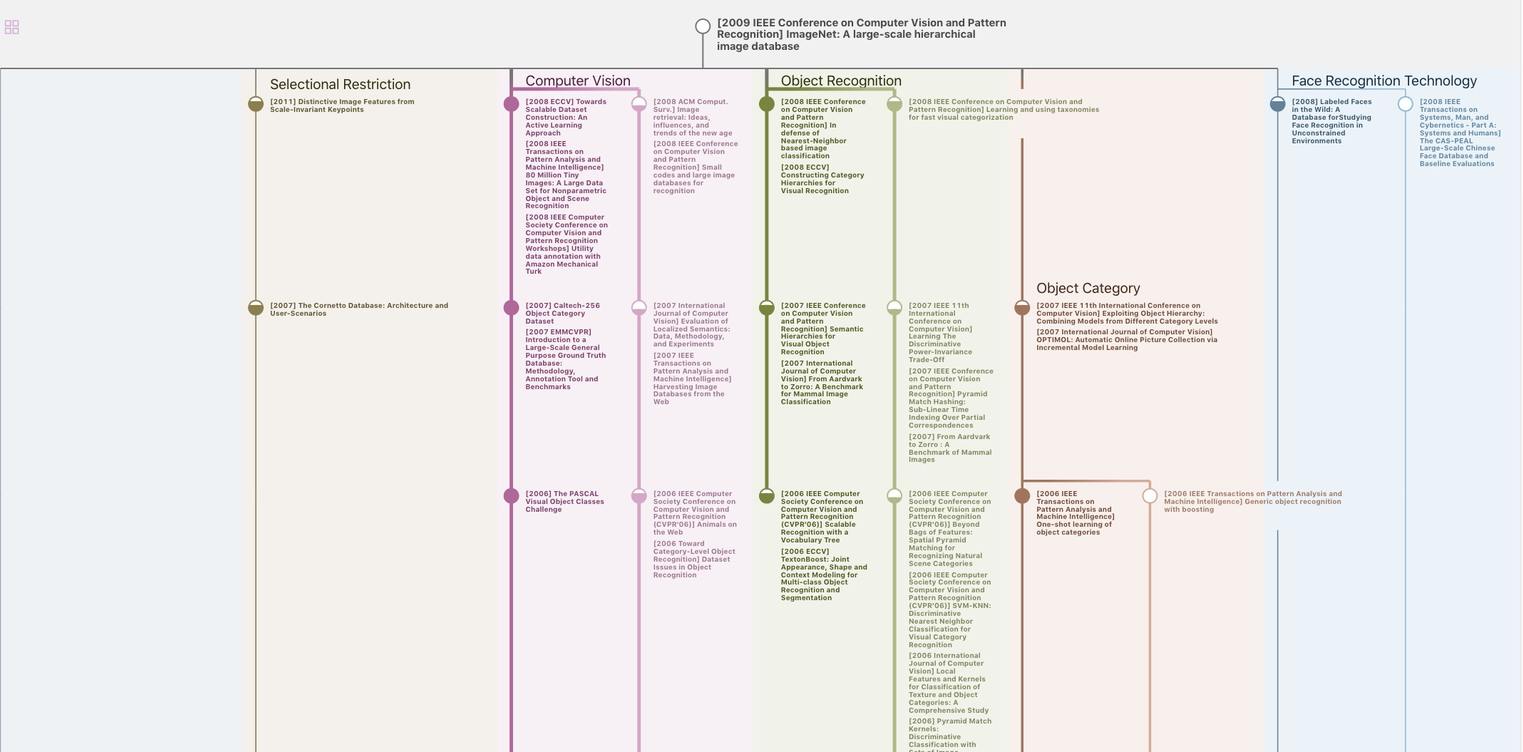
生成溯源树,研究论文发展脉络
Chat Paper
正在生成论文摘要