Two Heads Are Better Than One: Boosting Graph Sparse Training via Semantic and Topological Awareness
CoRR(2024)
摘要
Graph Neural Networks (GNNs) excel in various graph learning tasks but face
computational challenges when applied to large-scale graphs. A promising
solution is to remove non-essential edges to reduce the computational overheads
in GNN. Previous literature generally falls into two categories:
topology-guided and semantic-guided. The former maintains certain graph
topological properties yet often underperforms on GNNs due to low integration
with neural network training. The latter performs well at lower sparsity on
GNNs but faces performance collapse at higher sparsity levels. With this in
mind, we take the first step to propose a new research line and concept termed
Graph Sparse Training (GST), which dynamically manipulates sparsity at the data
level. Specifically, GST initially constructs a topology semantic anchor at a
low training cost, followed by performing dynamic sparse training to align the
sparse graph with the anchor. We introduce the Equilibria Sparsification
Principle to guide this process, effectively balancing the preservation of both
topological and semantic information. Ultimately, GST produces a sparse graph
with maximum topological integrity and no performance degradation. Extensive
experiments on 6 datasets and 5 backbones showcase that GST (I) identifies
subgraphs at higher graph sparsity levels (1.67
state-of-the-art sparsification methods, (II) preserves more key spectral
properties, (III) achieves 1.27-3.42× speedup in GNN inference and (IV)
successfully helps graph adversarial defense and graph lottery tickets.
更多查看译文
AI 理解论文
溯源树
样例
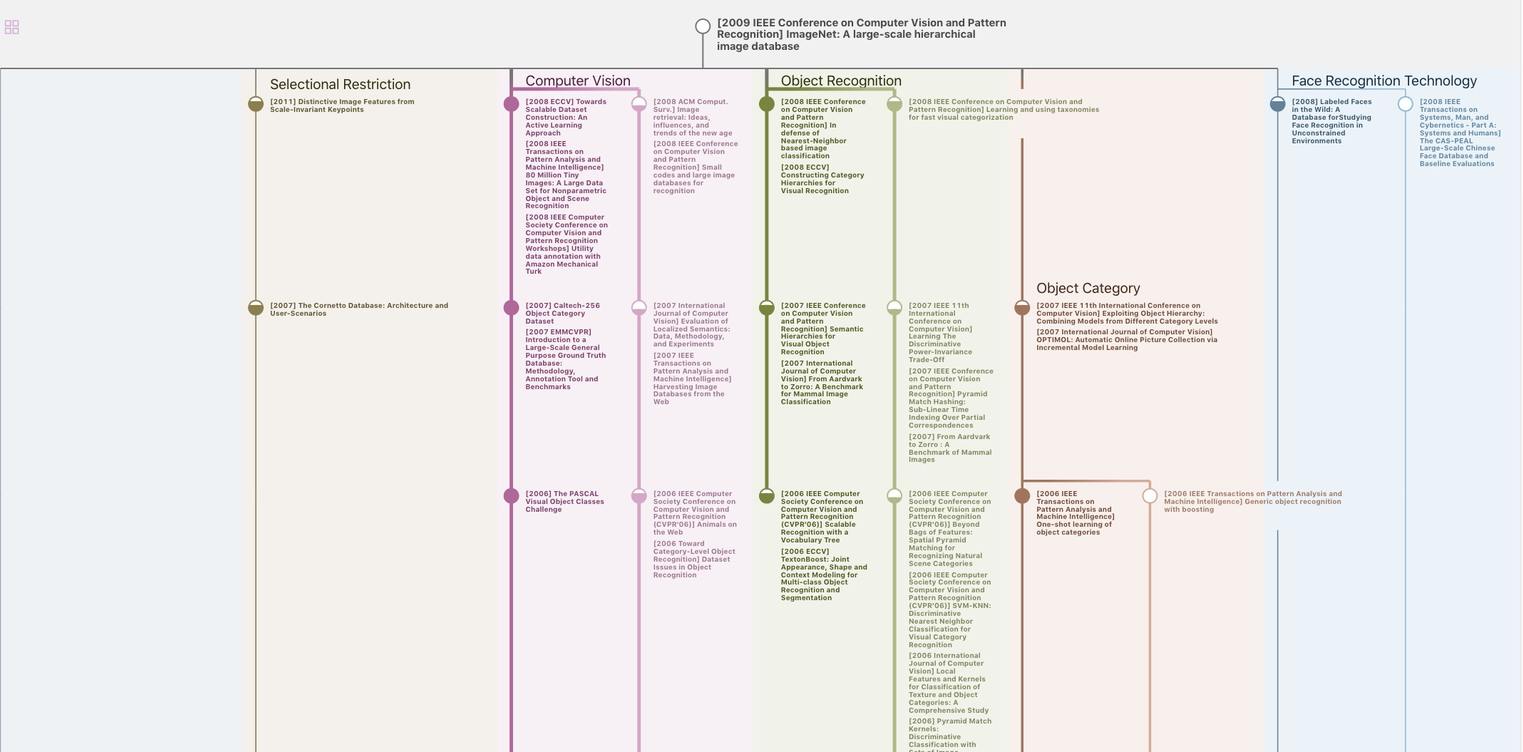
生成溯源树,研究论文发展脉络
Chat Paper
正在生成论文摘要