Retrieval Augmented End-to-End Spoken Dialog Models
CoRR(2024)
摘要
We recently developed SLM, a joint speech and language model, which fuses a
pretrained foundational speech model and a large language model (LLM), while
preserving the in-context learning capability intrinsic to the pretrained LLM.
In this paper, we apply SLM to speech dialog applications where the dialog
states are inferred directly from the audio signal.
Task-oriented dialogs often contain domain-specific entities, i.e.,
restaurants, hotels, train stations, and city names, which are difficult to
recognize, however, critical for the downstream applications. Inspired by the
RAG (retrieval-augmented generation) paradigm, we propose a retrieval augmented
SLM (ReSLM) that overcomes this weakness. We first train a speech retriever to
retrieve text entities mentioned in the audio. The retrieved entities are then
added as text inputs to the underlying SLM to bias model predictions. We
evaluated ReSLM on speech MultiWoz task (DSTC-11 challenge), and found that
this retrieval augmentation boosts model performance, achieving joint goal
accuracy (38.6
rate (5.5
is broadly applicable to other speech tasks requiring contextual information or
domain-specific entities, such as contextual ASR with biasing capability.
更多查看译文
关键词
Restaurants,Language Model,Train Station,Input Text,City Names,Audio Input,Word Error Rate,Speech Recognition,User Responses,Named Entity Recognition,Rare Entity,Speech Input,Correct Spelling,Speech Coding,Dialogue System,Text Modality,Chatbot
AI 理解论文
溯源树
样例
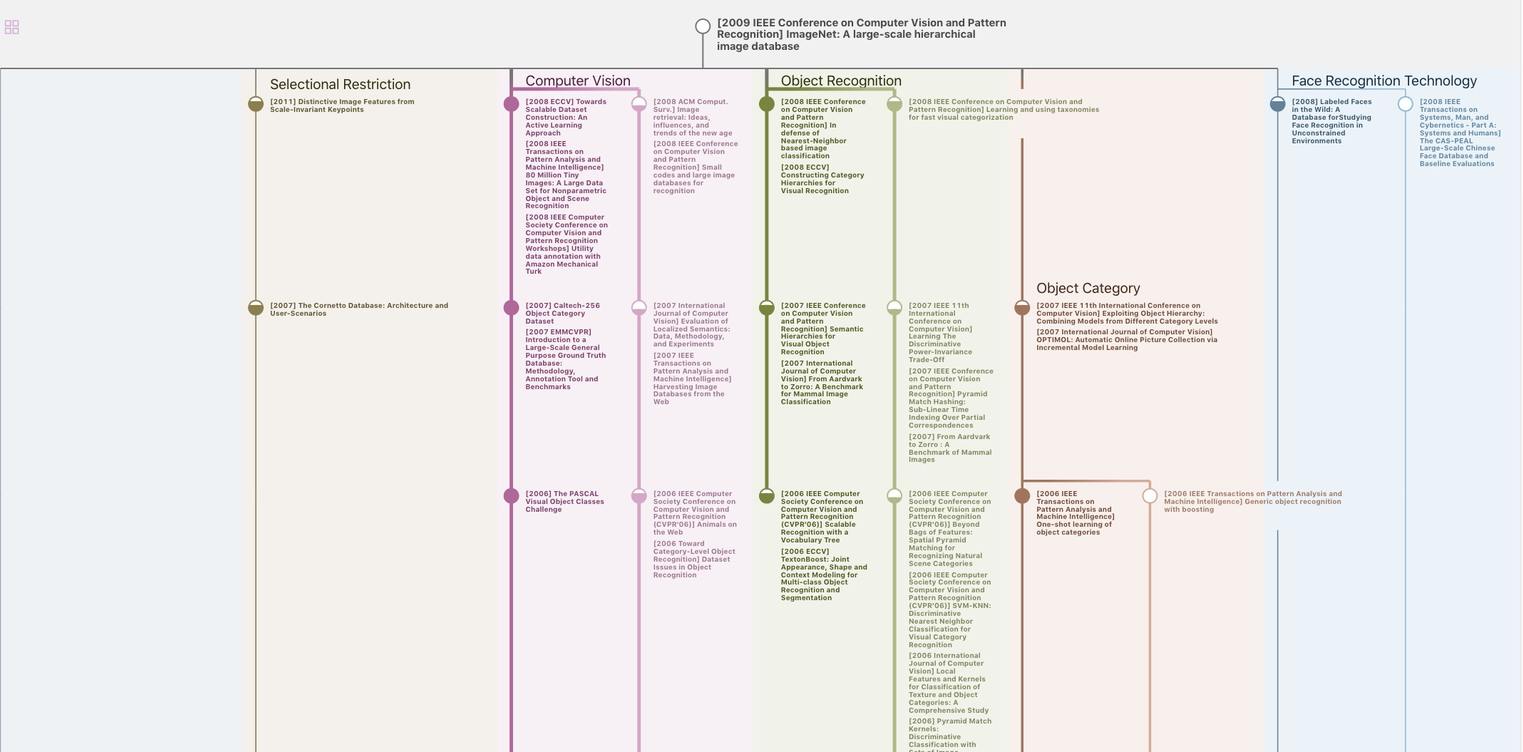
生成溯源树,研究论文发展脉络
Chat Paper
正在生成论文摘要