Self-supervised Pre -training for Robust and Generic Spatial -Temporal Representations
23RD IEEE INTERNATIONAL CONFERENCE ON DATA MINING, ICDM 2023(2023)
摘要
Advancements in mobile sensing, data mining, and artificial intelligence have revolutionized the collection and analysis of Human -generated Spatial -Temporal Data (HSTD), paving the way for diverse applications across multiple domains. However, previous works have primarily focused on designing taskspecific models for different problems, which lack transferability and generalizability when confronted with diverse IISTD. Additionally, these models often require a large amount of labeled data for optimal performance. While pre-trained models in Natural Language Processing (NLP) and Computer Vision (CV) domains have showcased impressive transferability and generalizability, similar efforts in the spatial-temporal data domain have been limited. In this paper, we take the lead and introduce the SpatialTemporal Pre -Training model, i.e., STPT, which is connected with a self-supervised learning task, to address these limitations. STPT enables the creation of robust and versatile representations of HSTD. We validate our framework using real-world data and demonstrate its efficacy through two downstream tasks, i.e., trajectory classification and driving activity identification (e.g., identifying seeking vs. serving behaviors in taxi trajectories). Our results achieve an accuracy of 83.125% (16.2% higher than the average baseline) for human mobility identification and an accuracy of 77.88% (13.0% higher than the average baseline) for the human activity identification task. These outcomes underscore the potential of our pre-trained model for diverse downstream applications within the spatial -temporal data domain.
更多查看译文
关键词
pre-training,self-supervised learning,spatial temporal data mining,driver identification,human decision analysis
AI 理解论文
溯源树
样例
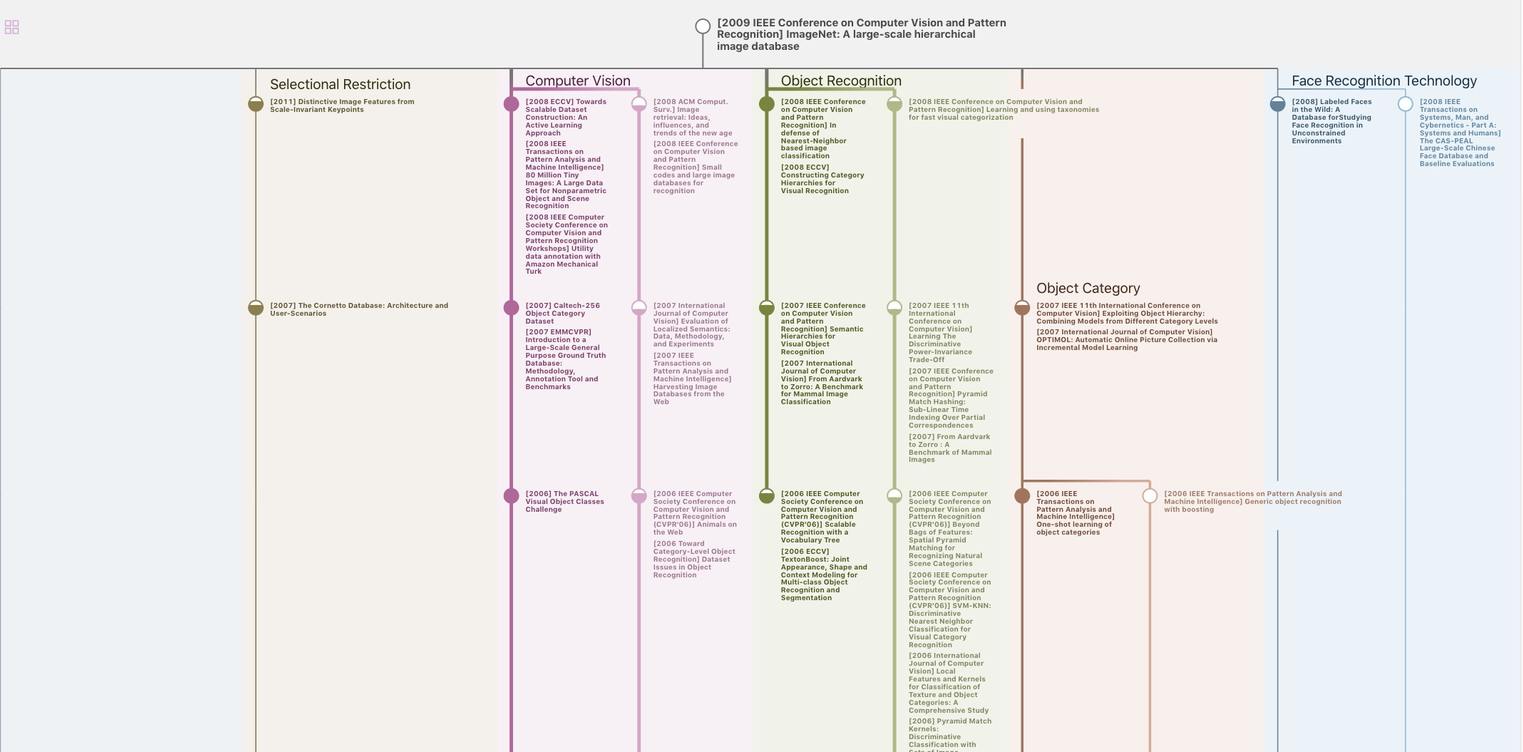
生成溯源树,研究论文发展脉络
Chat Paper
正在生成论文摘要