Approximating the Core Via Iterative Coalition Sampling
AAMAS '24 Proceedings of the 23rd International Conference on Autonomous Agents and Multiagent Systems(2024)
摘要
The core is a central solution concept in cooperative game theory, defined asthe set of feasible allocations or payments such that no subset of agents hasincentive to break away and form their own subgroup or coalition. However, ithas long been known that the core (and approximations, such as the least-core)are hard to compute. This limits our ability to analyze cooperative games ingeneral, and to fully embrace cooperative game theory contributions in domainssuch as explainable AI (XAI), where the core can complement the Shapley valuesto identify influential features or instances supporting predictions byblack-box models. We propose novel iterative algorithms for computing variantsof the core, which avoid the computational bottleneck of many other approaches;namely solving large linear programs. As such, they scale better to very largeproblems as we demonstrate across different classes of cooperative games,including weighted voting games, induced subgraph games, and marginalcontribution networks. We also explore our algorithms in the context of XAI,providing further evidence of the power of the core for such applications.
更多查看译文
AI 理解论文
溯源树
样例
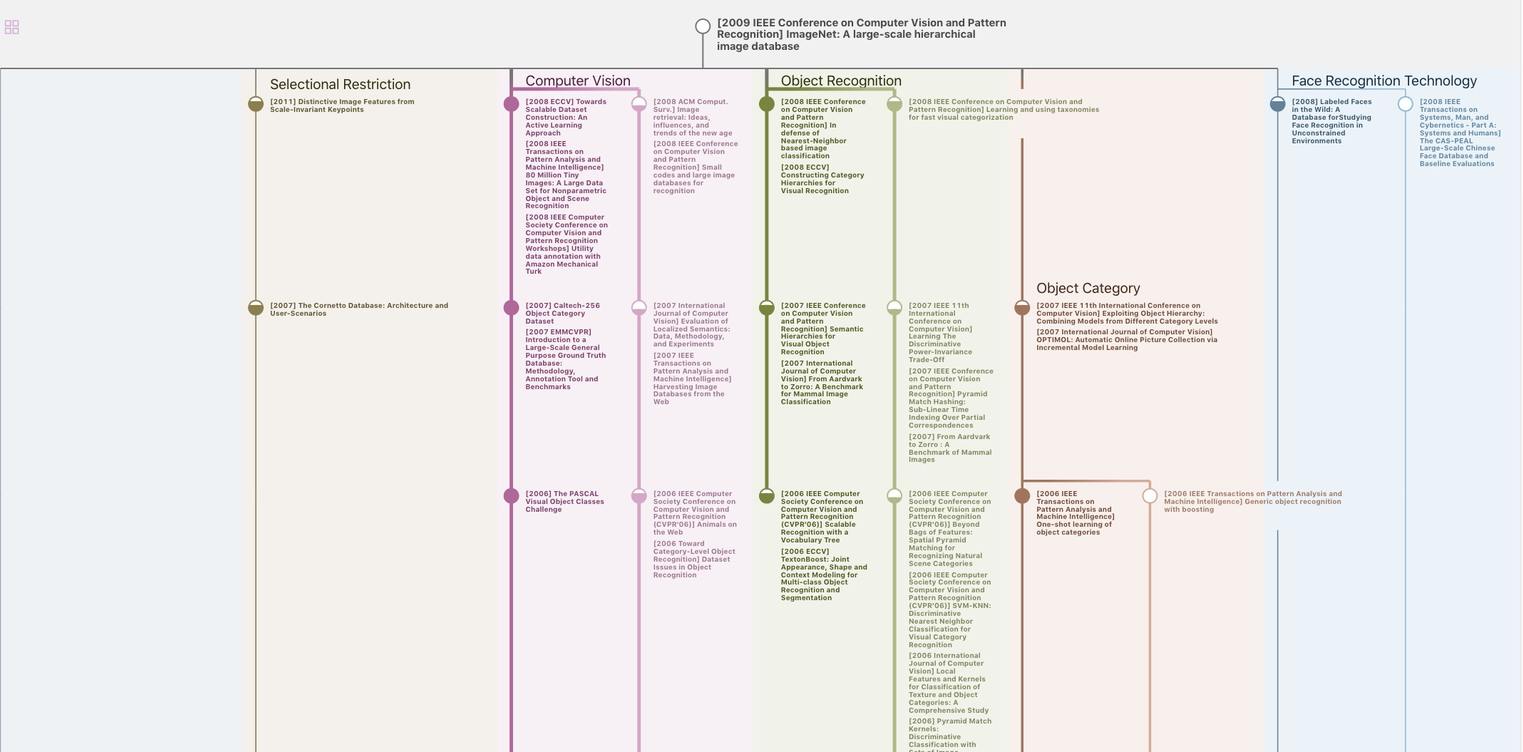
生成溯源树,研究论文发展脉络
Chat Paper
正在生成论文摘要