Knowledge Graph Embedding via Triplet Component Interactions
Neural Processing Letters(2024)
摘要
In knowledge graph embedding, multidimensional representations of entities and relations are learned in vector space. Although distance-based graph embedding methods have shown promise in link prediction, they neglect context information among the triplet components, i.e., the head_entity, relation, and tail_entity, limiting their ability to describe multivariate relation patterns and mapping properties. Such context information denotes the entity structural association inside the same triplet and implies the correlation between entities that are not directly connected. In this work, we propose a novel knowledge graph embedding model that explicitly considers context information in graph e mbedding via t riplet c omponent i nteractions (TCIE). To build connections between components and incorporate contextual information, entities and relations are represented as vectors comprised of two specialized parts, enabling comprehensive interaction. By simultaneously interacting with one-hop related head and tail entities, TCIE strengthens the connections between distant entities and enables contextual information to be transmitted across the knowledge graph. Mathematical proofs and experiments are performed to analyse the modelling ability of TCIE in knowledge graph embedding. TCIE shows a strong capacity for modelling four relation patterns (i.e., symmetry, antisymmetry, inverse, and composition) and four mapping properties (i.e., one-to-one, one-to-many, many-to-one, and many-to-many). The experimental evaluation of ogbl-wikikg2, ogbl-biokg, FB15k, and FB15k-237 shows that TCIE achieves state-of-the-art results in link prediction.
更多查看译文
关键词
Knowledge graph embedding,Knowledge graph completion,Triplet component interaction,Context information
AI 理解论文
溯源树
样例
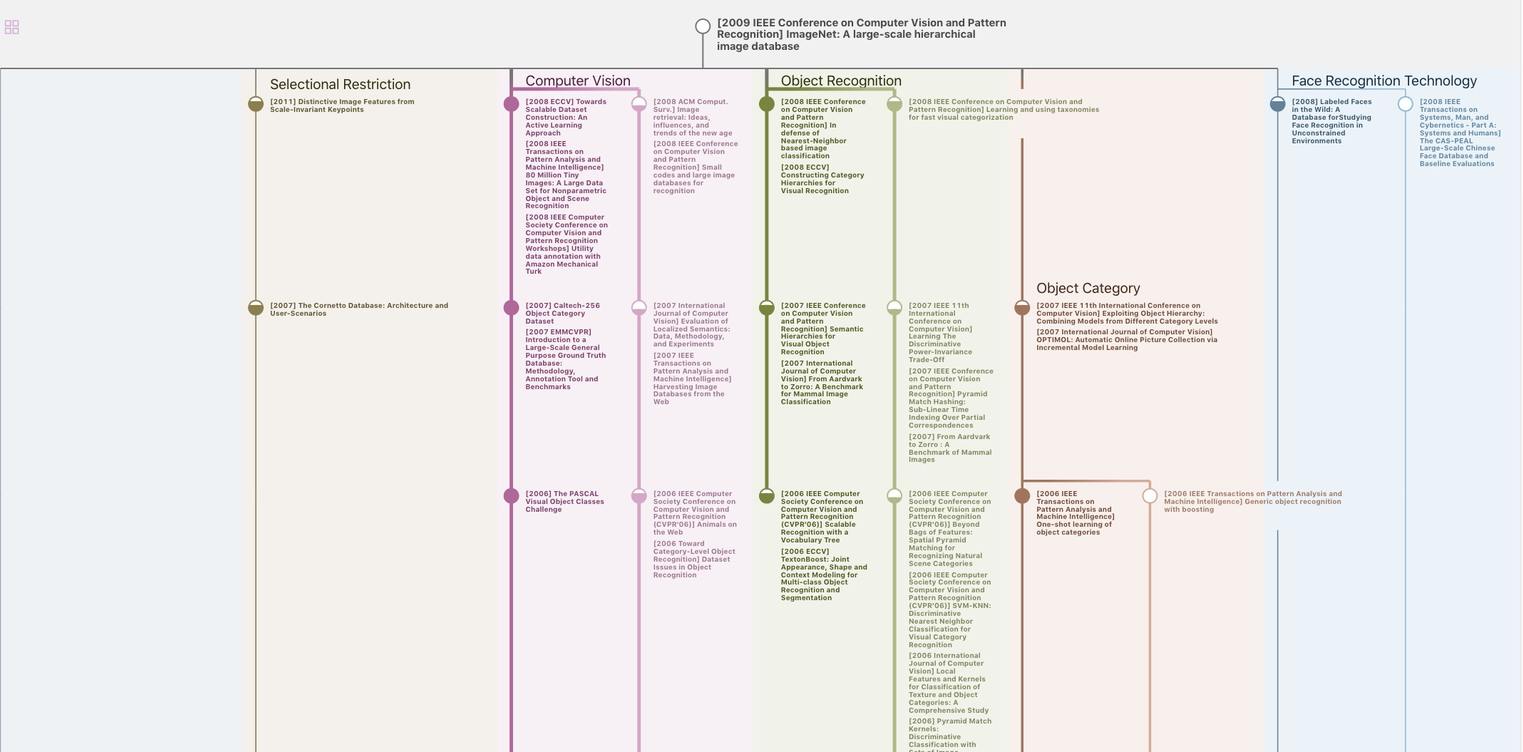
生成溯源树,研究论文发展脉络
Chat Paper
正在生成论文摘要