Incorporating Retrieval-based Causal Learning with Information Bottlenecks for Interpretable Graph Neural Networks
CoRR(2024)
摘要
Graph Neural Networks (GNNs) have gained considerable traction for their
capability to effectively process topological data, yet their interpretability
remains a critical concern. Current interpretation methods are dominated by
post-hoc explanations to provide a transparent and intuitive understanding of
GNNs. However, they have limited performance in interpreting complicated
subgraphs and can't utilize the explanation to advance GNN predictions. On the
other hand, transparent GNN models are proposed to capture critical subgraphs.
While such methods could improve GNN predictions, they usually don't perform
well on explanations. Thus, it is desired for a new strategy to better couple
GNN explanation and prediction. In this study, we have developed a novel
interpretable causal GNN framework that incorporates retrieval-based causal
learning with Graph Information Bottleneck (GIB) theory. The framework could
semi-parametrically retrieve crucial subgraphs detected by GIB and compress the
explanatory subgraphs via a causal module. The framework was demonstrated to
consistently outperform state-of-the-art methods, and to achieve 32.71% higher
precision on real-world explanation scenarios with diverse explanation types.
More importantly, the learned explanations were shown able to also improve GNN
prediction performance.
更多查看译文
AI 理解论文
溯源树
样例
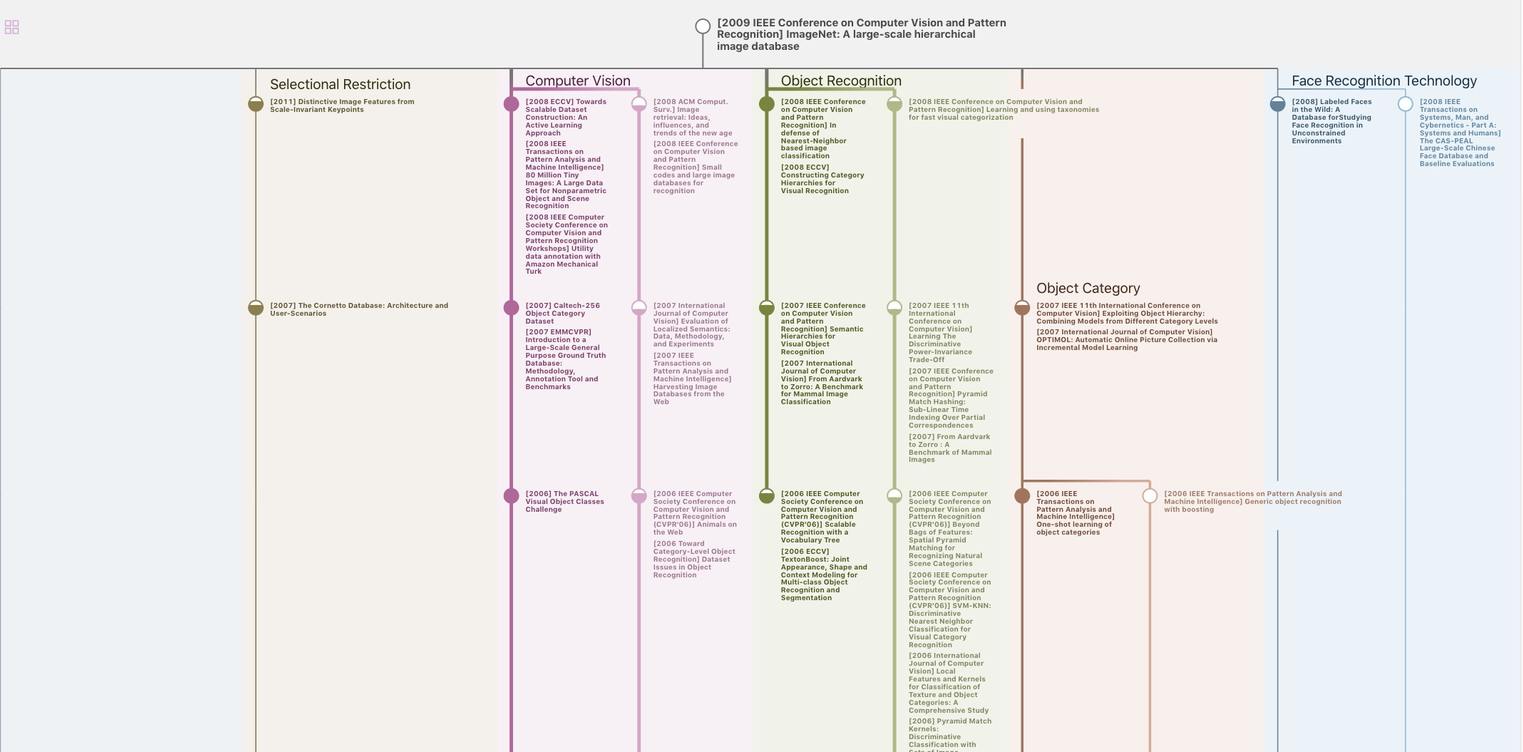
生成溯源树,研究论文发展脉络
Chat Paper
正在生成论文摘要