Quantum Speedup for Spectral Approximation of Kronecker Products
CoRR(2024)
摘要
Given its widespread application in machine learning and optimization, the
Kronecker product emerges as a pivotal linear algebra operator. However, its
computational demands render it an expensive operation, leading to heightened
costs in spectral approximation of it through traditional computation
algorithms. Existing classical methods for spectral approximation exhibit a
linear dependency on the matrix dimension denoted by n, considering matrices
of size A_1 ∈ℝ^n × d and A_2 ∈ℝ^n ×
d. Our work introduces an innovative approach to efficiently address the
spectral approximation of the Kronecker product A_1 ⊗ A_2 using quantum
methods. By treating matrices as quantum states, our proposed method
significantly reduces the time complexity of spectral approximation to
O_d,ϵ(√(n)).
更多查看译文
AI 理解论文
溯源树
样例
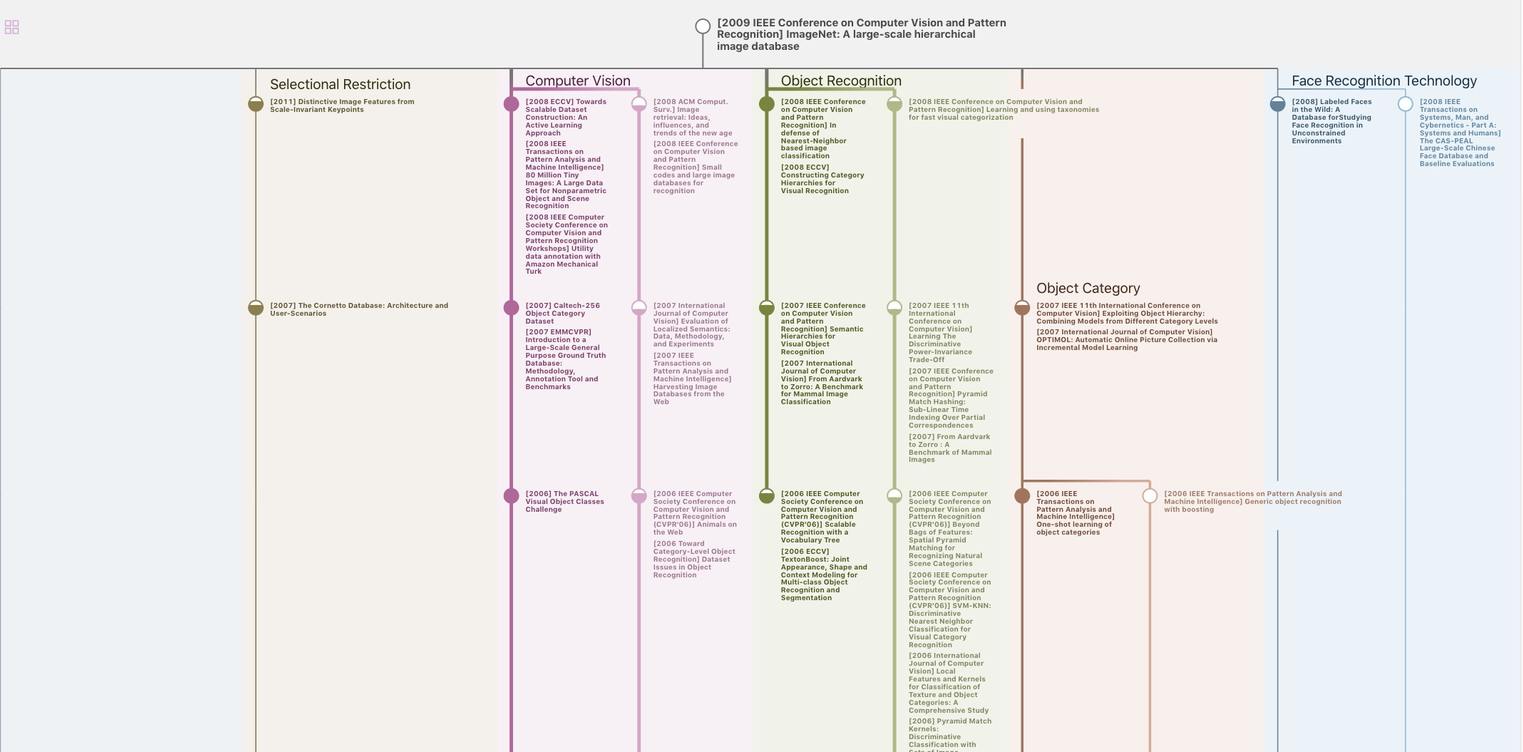
生成溯源树,研究论文发展脉络
Chat Paper
正在生成论文摘要