Interaction-Aware Trajectory Prediction with Point Transformer
2023 IEEE 26TH INTERNATIONAL CONFERENCE ON INTELLIGENT TRANSPORTATION SYSTEMS, ITSC(2023)
摘要
To ensure safe and efficient autonomous driving, trajectory prediction system must account for social interactions among road participants. Graph-based models are leading approaches in modeling social interactions for trajectory prediction, but they face the challenges of designing an appropriate graph structure and processing complex interactions. We consider that the participants in a scene are a set of unstructured points, which are similar to point cloud data. Inspired by point cloud learning networks, we view the road participants in a scene as point cloud in a two-dimensional coordinate system, and utilize Point Transformer aggregator to process the interactions on both local and global level. Besides, we present a multiplex fusion of social and temporal information for trajectory prediction. We perform extensive experiments on the Argoverse motion forecasting dataset, and the results demonstrate the superior performance of our model for multi-agent trajectory prediction.
更多查看译文
关键词
Trajectory Prediction,Interaction-aware Trajectory Prediction,Social Interaction,Global Level,Point Cloud,Temporal Information,Social Information,Graph Structure,Information Fusion,Point Cloud Data,Graph-based Models,Time Step,Validation Set,Attention Mechanism,Local Interactions,Temporal Correlation,Motion Patterns,Map Information,Embedding Dimension,Interaction Module,Heterogeneous Graph,Transformer Encoder,Graph Neural Networks,Global Interaction,Temporal Coding,Future Trajectories,Displacement Error,Global Motion,Long-range Dependencies,Local Module
AI 理解论文
溯源树
样例
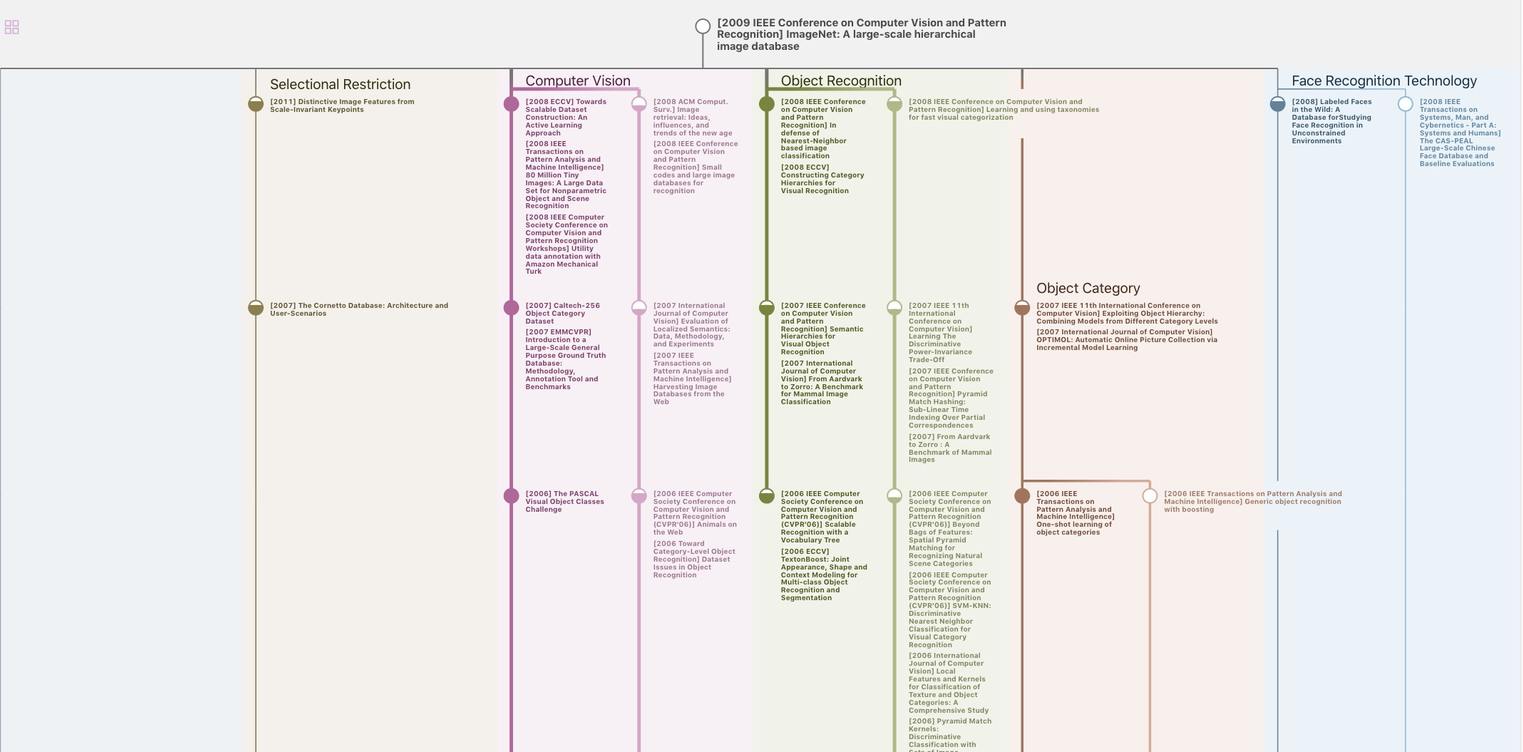
生成溯源树,研究论文发展脉络
Chat Paper
正在生成论文摘要